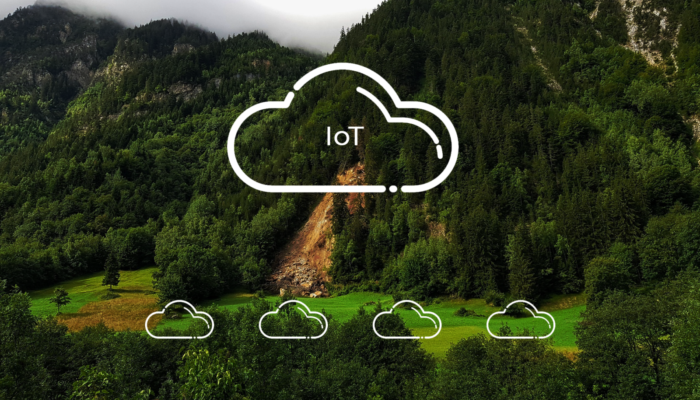
In my blog post last year, I talked about the escalation of extreme events in Brazil, and one of the main questions was whether the scientific community would need new methods and technologies to deal with extreme events aggravated by climate change. This year, during one of the Innovations (WG05) working group meetings of the International Network on Landslide Early Warning Systems (LandAware), I was introduced to the use of the Internet of Things (IoT) applied to landslide monitoring. Although the use of IoT in natural hazards is pretty new – at least applied to landslides – today I present to you why this technology presents a great potential towards Disaster Risk Reduction (DRR).
Before addressing the key benefits of IoT to natural hazards, it is worth going through what this technology actually is, how it was enabled, and how it operates. IoT or the Internet of Things describes the online hi-tech machine-to-machine interaction of data between physical devices – “things” – and other web-based receiving devices. An everyday example of how IoT works is when you routinely order from your mobile phone an app-based transportation service when leaving the office. Thanks to the connections and information extracted by the IoT, the app then uses this pattern of requests to suggest new ones. Their connections can be broader than that, ranging from household objects to buildings, cities and, why not, environmental applications. The idea of IoT is not brand new; however, it has become practical and low-cost based on the advances of other technologies, including low-power sensor technology, cloud computing platforms, big data, machine learning and analytics and conversational artificial intelligence [1].
IoT applications within the landslide community
The first LandAware meetings on IoT applied to Landslide Early Warning Systems (LEWS) run in May 2022, showed the potential of IoT to improve landslides early warning. Subsequently, to share experiences in real-time monitoring and modelling for early warning purposes, the IoT-based methods and analyses (WG08) working group was launched. The experiences shared by members acting in pilot and local projects have demonstrated the advantages of using real-time data, such as anticipating an alert and using Artificial Intelligence to obtain predictions [2].
Learning more about landslide science, IoT can be used to obtain real-time weather forecasts and soil moisture monitoring with geosensors. The main advantage of using geosensors and other field equipment, such as rain gauges, drones, etc., is their accessibility to be connected to an online network that allows the transmission of this data to a receiving station. It means that we can monitor and gather environmental conditions from several objects in real time with reduced levels of uncertainty. The very first application of the IoT-based LEWS was in railways in Norway where they utilised water volumetry and temperature sensors to monitor, model, and determine the stabilisation alerts for an unsaturated soil slope. After its first successful application, it was concluded that it is possible to use real-time monitoring in a local LEWS to alert authorities in advance of a landslide warning [2].
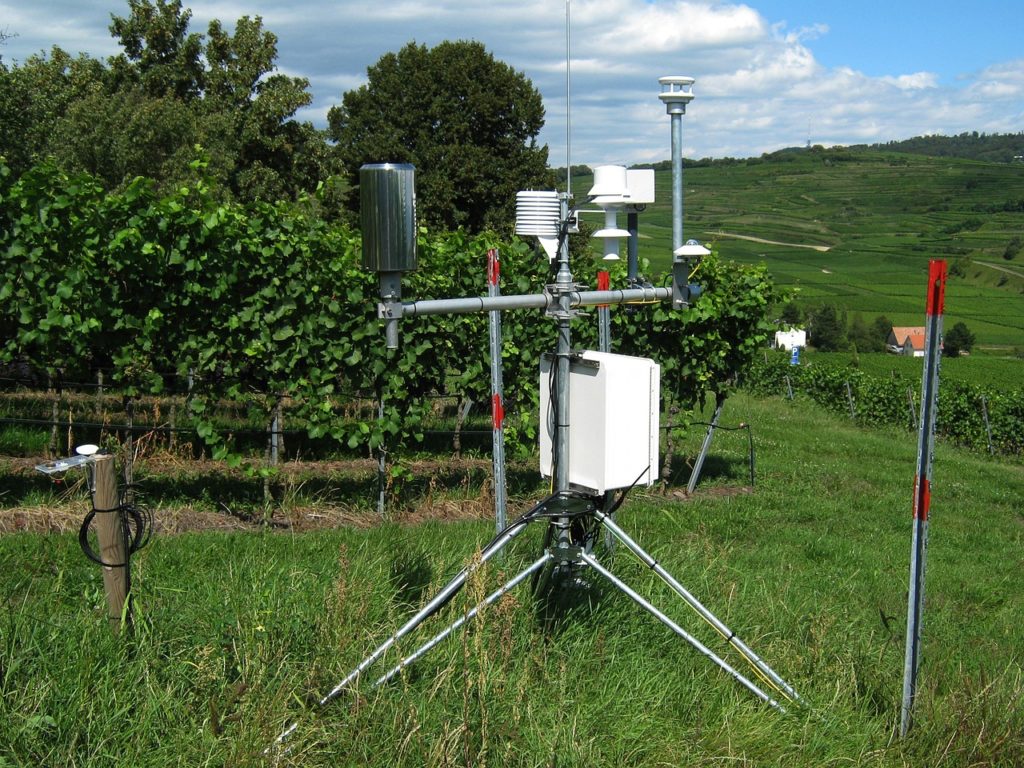
A field device such as this can be attached to a sensor and transmit data in real-time online. Photo credit: Image by Gerd Altmann from Pixabay (Pixabay licence)
Why is IoT handy in a climate-changing world?
One of our biggest challenges with climate change is trying to predict the behaviour of extremely rapid climate change, which makes weather forecasts have high levels of uncertainty and no accuracy under extreme events. By contrast, the IoT allows us to integrate the information obtained by multiple equipment operating in the field simultaneously and through software, plot them on a web page to monitor their behaviour. This advantage extends to modelling, as there is always a gap in the intersection of the occurrence of a landslide and the amount of rain that triggered it, and real-time monitoring decreases this uncertainty. Recent scientific work, such as the one by Thirugnanam et al. [1], proves that it is possible to make this prediction with greater accuracy. Conversely, it is still unclear whether it is possible to obtain anticipations for extreme rainfall events. Above all, IoT technology is a promising way forward and a new card available to play as it is deployable to what is already existing and has a low cost, albeit it has to be further explored.
References
[1] H. Thirugnanam, S. Uhlemann, R. Reghunadh, M. V. Ramesh and V. P. Rangan, “Review of Landslide Monitoring Techniques With IoT Integration Opportunities,” in IEEE Journal of Selected Topics in Applied Earth Observations and Remote Sensing, vol. 15, pp. 5317-5338, 2022, doi: 10.1109/JSTARS.2022.3183684
[2] Piciullo, L., Capobianco, V. & Heyerdahl, H. A first step towards a IoT-based local early warning system for an unsaturated slope in Norway. Nat Hazards (2022) https://doi.org/10.1007/s11069-022-05524-3
Post edited by Silvia De Angeli and Shreya Arora