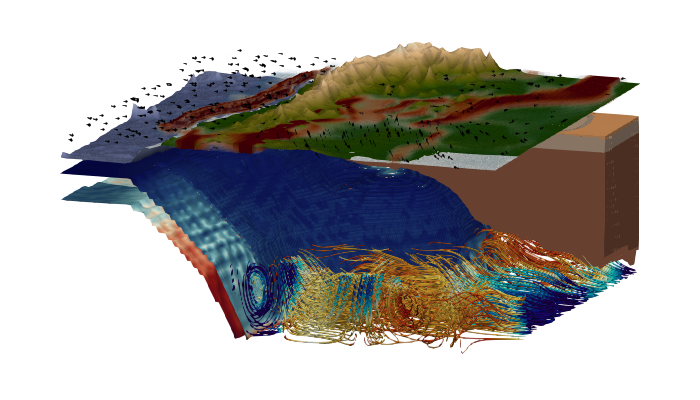
Geodynamic data-driven models are powerful tools that combine the strengths of both dynamic and static geodynamic models, enabling researchers to answer specific questions about the Earth’s processes. By assimilating observations and integrating physics-based models, data-driven simulations offer a way to gain a better understanding of how long- and short-term tectonic processes interact. In this article, we explore the advantages of geodynamic data-driven models, their assimilation of observations, and examples of their applications in addressing long-standing questions about the Earth’s processes. We also discuss their potential in tackling contemporary challenges such as geohazard mitigation and climate change.
The rise of data-driven models
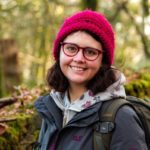
Dr. Constanza Rodriguez Piceda is a postdoctoral researcher at Plymouth University (United Kingdom). Her work ranges from lithosphere to earthquake cycle modelling. Follow her on Twitter for the latest updates on her research.
One of the biggest challenges in the field of Geodynamics is to cover processes taking place at multiple spatial and temporal scales: from the deep Earth to the surface. This is necessary to address questions such as what drives plate motions, the formation of mountains, volcanism and sedimentary basins. The study of these processes is a difficult task because some of the scales are beyond our observations and measurements. Unfortunately, unlike in the infamous movie ‘The Core’, we cannot call Aaron Eckhart and Hillary Swank to drill the Earth all the way down to its centre; nor, we do not have the luxury of riding in a DeLorean to travel back in time and observe the Earth as it appeared tens of millions of years ago, like in the movie ‘Back to the Future’. Therefore, we use computational modelling to simulate different processes by incorporating the physics of our world.
Two types of simulations are generally used in computational modelling. Classical “dynamic” models simulate plate motion in a given time interval, thus they are powerful tools that give insights on the evolution of a process. The second type of simulations, “static” models, is useful to describe the structure and state of the Earth’s layers at a given moment in time, most commonly at present-day, by integrating a maximum of observations from different sources. Despite being complementary, these two types of models have been evolving side-by-side. However, in the last few years, with the increasing amount of geological and geophysical data available, a new type of model, which takes advantage of both of the modelling approaches, started to rise. In this blog, I would like to develop on the use of this specific type of model called “geodynamic data-driven model”. In the following, I will briefly try to answer the following questions: What types of models are these? What are the perks of combining these models? What is the starting point for using them? Which kind of applications do they have? What are their limitations and how can they be used in the future?
Snapshots of the Earth: static lithosphere models
Static geodynamic models describe the state of the Earth’s layers in terms of their composition, geometry and temperature. These simulations are built upon different geological, geophysical, geochemical and geodetic observations which tell us about the rock properties of the Earth. For example, we can use seismic tomography and gravity modelling to characterise the Earth structure and, by coupling to mineral physics, we can define the composition and rheology of crustal and mantle rocks. For instance, forward modelling and/or inversion of gravimetric data has helped to constrain the thickness and composition of the lithosphere to build static lithospheric-scale models (just to name a few, Afonso et al., 2019; Fullea et al., 2014; Gómez Dacal et al., 2017; Rodriguez Piceda et al., 2021; Tassara et al., 2006). Moreover, given the increasing resolution of the data, we are now able to draw sharper and more accurate terrain boundaries (e.g., Gómez-García et al., 2019). Integrating data of different nature is also done in applied geophysics related to oil and gas and mining, where the structures of sedimentary layers and the shallow crystalline crust are constrained over a few kilometres depth. Although these static models allow us to stay as close as possible to the data, they cannot tell us a lot more about the evolution of the system. For example, what is the exact role of the inherited structures on the present-day deformation ? Where is the stress-strain most likely to localise? To answer these questions, the use of another type of model is required.
A recipe for a static lithosphere model
During my PhD at GFZ Potsdam (Section 4.5), I have built a static lithospheric-scale model of regional subduction in the context of the Pampean flat subduction in the Andes that can be summarised in 3 steps (Figure 1).
In step 1, I have constrained the structure and density of the lithosphere, and inferred the average composition of the South American plate (Rodriguez Piceda et al., 2021). In step 2, I have built a thermal model of the lithosphere by: (i) converting the seismic velocity of the mantle to temperature using mineral physics, and (ii) running a numerical thermal simulation using the previous lithosphere structures, further constrained by temperature and surface heat flow measurements (Rodriguez Piceda et al., 2022a). Finally, in step 3, the compositional and thermal models were used to compute the strength of the lithosphere into a rheological model (Rodriguez Piceda et al., 2022b).
Fig. 1. General workflow to build a static model describing the density, thickness, temperature and strength of the lithosphere. Boundary conditions used for step (2) are the top and bottom temperature of the model.
As you probably noticed already, the quality of the final lithospheric model directly results from the quality of the data in the input. The final lithospheric strength map is therefore sensitive to the long-term lithospheric scale structure. Additionally, as faults are not explicitly accounted for, the impact of these smaller-scale features cannot be observed. To capture the deformation across faults and their dynamic influence on lithospheric strength, a geodynamic data-driven model can be employed. But what do these models entail?
Assimilating Observations with Geodynamic Data-Driven Models: Moving from Static to Dynamic Modeling
In their “Geodynamics 101” article, Van Zelst et al. (2022) described two types of modelling philosophies: generic and specific models. In the first type, geodynamic models are used to assess what causes the general behaviour of a system. In the second type, geodynamic modelling is used to understand a specific state of a particular regional tectonic setting. The latter case requires a specific model with a more constrained parametrization to reconcile physics-based processes with observations. It is common to use a “forward modelling” approach that begins with a simple initial description of the system and refine the model through an iterative process, to simulate the effect of plate geometry, temperature, and velocities. This modelling choice stems from the fact that we don’t have full information about the past lithospheric architecture, and geoscientists can have a better grasp of what factors are more important than others for a given process by starting with a simplified initial condition. With each iteration, the model becomes more accurate and sophisticated. The preferred simulation is the one that matches the best the observational data. These specific models are sometimes called “data-driven models” (Ismail-Zadeh (2021) provides a comprehensive review of them).
Another kind of “data-driven” simulations are those that integrate observations as part of the initial conditions, significantly reducing the amount of solutions. One approach to achieve this is by integrating previous “static” models. A key characteristic of these models is that, if well done, they become quasi-instantaneous, meaning that they are only run for a couple of hundred to few hundreds of thousand years, the time it takes to isostatically re-equilibrate. Given these gains in computational time, we can test more parameters which allows a more statistical approach of the results compared to the specific models previously discussed. Data-driven models were early-on used in mantle dynamics, by converting seismic tomography to temperature and viscosity using mineral physics, by inverting the momentum and energy equations and “playing backward” the mantle flow of the Earth. (e.g., CitcomS, G-ADOPT initiative). More recently, advances in numerical codes (e.g., LAMEM and ASPECT ) have allowed us to combine “static” and “dynamic” models to solve questions about lithosphere dynamics. For instance, the possibility to deform the mesh by prescribing an initial topography instead of a “sticky layer” (Kaus et al., 2010) let us reach a quicker isostatic balance without deviating too much from the initial geometry of the plate, which is an advantage for modelling work focusing on crustal and lithospheric deformation.
Data-driven models can be used to address long-standing questions on the role of the inherited crustal or lithospheric scale structures on the present-day deformation. How does the present-day architecture of the plates influence the distribution of surface deformation that we observe in the Andes? (Ibarra et al., 2019, LAMEM;, Pons & Rodriguez Piceda, et al., 2023-preprint, ASPECT)? What is the effect of crustal-scale heterogeneities and oceanic slabs in the observed uplift of the Alps? (Kumar et al., 2022, LAMEM)? How sensible are surface plate motions to variations in sublithospheric processes such as plate coupling, mantle flow and slab pull forces? (Glerum et al., 2021-preprint, ASPECT) How does inherited lithospheric strength affect the opening of the East-African rift (Glerum et al., 2020, ASPECT)? are some of the questions that were tackled with this approach. Therefore, these models can constitute a ground for communication between different disciplines such as geodesy, geodynamics, structural geology and geochemistry.
Assimilating Observations in Geodynamic Data-Driven Models: Moving from Static to Dynamic Modeling
Many advanced geodynamic codes offer the possibility to customise the geometry of the plate (e.g., LAMEM, ASPECT, Underworld). As mentioned earlier, static lithospheric scale models are very much dependent on the input information and do not allow to compute the dynamic deformation. During my PhD with Michael Pons, we tried to connect static and dynamic models by using the open-source software ASPECT to incorporate the static model of the Pampean flat subduction in the Andes into a geodynamic simulation (Pons and Rodriguez Piceda et al., 2023a-b-preprint). The structural configuration, including the topography, and thermal model were assigned as initial conditions through ASCII files. Finally we prescribed plate velocities at the boundaries. Unlike the static simulations described before, the advantage now is that faults can emerge spontaneously due to the interactions between different layers and forces (Figure 2). These faults appear without explicit pre-defined parameters and can provide valuable insights into the behaviour and evolution of the system.
Fig. 2. Geodynamic data driven Model showing strain localization at the surface (red areas) as the result of plate convergence and inherited structures of the South American plate.
Data-driven models are not all roses
While we can gain valuable insights from these models, they are not without limitations. For instance, the quality of input data in terms of resolution, spatial coverage, and uncertainty is crucial for static models. On the other hand, 3D simulations can become excessively intricate. Model complexity is another limitation, as it requires a balance between computational resources and the solver’s ability to converge. We must always consider the feasibility of running a model in terms of computational resources and time. Despite efforts to refine areas that will experience more deformation, such as fault regions, there is always a resolution limit due to computational resources.
The future of geodynamic modelling: towards an Earth digital twin
The lithosphere plays a crucial role to humans because it serves as the primary source of natural resources that are essential to modern society. Additionally, it is a critical location where many essential geological processes occur, including some that pose geohazard risks, such as earthquakes and volcanic activity. These recent advancements in geodynamic modelling have brought about new opportunities to better understand such geological processes. By integrating present-day observations with physics-based models, data-driven simulations can be used to tackle contemporary geohazard and climatic challenges.
For instance, future earthquake simulators could be improved by coupling data-driven models with dynamic simulations of the earthquake cycle, being useful for probabilistic hazard assessment. Similarly, including surface processes and the effects of climatic variability into these models could help us to increase our understanding of other geohazards like landslides. Finally, using these models, we can use these models to study how mantle convection contributes to the formation of topography, which has an impact on changes in sea-level and ice-sheet dynamics.
By combining data-driven simulations with physics-based models, we can gain a better understanding of how long- and short-term tectonic processes interact with one another. Exciting times lie ahead for geodynamics!
References Afonso, J. C., Salajegheh, F., Szwillus, W., Ebbing, J., & Gaina, C. (2019). A global reference model of the lithosphere and upper mantle from joint inversion and analysis of multiple data sets. Geophysical Journal International, 217(3), 1602-1628. Fullea, J., Muller, M. R., Jones, A. G., & Afonso, J. C. (2014). The lithosphere–asthenosphere system beneath Ireland from integrated geophysical–petrological modeling II: 3D thermal and compositional structure. Lithos, 189, 49-64. Gomez Dacal, M., Tocho, C., Aragón, E., Sippel, J., Scheck-Wenderoth, M., & Ponce, A. (2017). Lithospheric 3D gravity modelling using upper-mantle density constraints: Towards a characterization of the crustal configuration in the North Patagonian Massif area, Argentina. Tectonophysics, 700, 150-161. Fullea, J., Muller, M. R., Jones, A. G., & Afonso, J. C. (2014). The lithosphere–asthenosphere system beneath Ireland from integrated geophysical–petrological modeling II: 3D thermal and compositional structure. Lithos, 189, 49-64. Glerum, A., Brune, S., Stamps, D. S., & Strecker, M. R. (2020). Victoria continental microplate dynamics controlled by the lithospheric strength distribution of the East African Rift. Nature Communications, 11(1), 2881. Glerum, A., Spakman, W., van Hinsbergen, D. J., Thieulot, C., & Pranger, C. (2021). Sensitivity of horizontal surface deformation to mantle dynamics from 3D instantaneous dynamics modeling of the eastern Mediterranean region. (preprint EarthArxiv) Ibarra, F., Liu, S., Meeßen, C., Prezzi, C. B., Bott, J., Scheck-Wenderoth, M., ... & Strecker, M. R. (2019). 3D data-derived lithospheric structure of the Central Andes and its implications for deformation: insights from gravity and geodynamic modelling. Tectonophysics, 766, 453-468. Ismail-Zadeh, A. (2021). Data-driven Geodynamics. Journal of the Geological Society of India, 97, 223-226. Kaus, B. J., Mühlhaus, H., & May, D. A. (2010). A stabilization algorithm for geodynamic numerical simulations with a free surface. Physics of the Earth and Planetary Interiors, 181(1-2), 12-20. Kumar, A., Cacace, M., Scheck‐Wenderoth, M., Götze, H. J., & Kaus, B. J. (2022). Present‐Day Upper‐Mantle Architecture of the Alps: Insights From Data‐Driven Dynamic Modeling. Geophysical Research Letters, 49(18), e2022GL099476. Pons, M., Rodriguez Piceda, C., Sobolev, S., Scheck-Wenderoth, M., & Strecker, M. (2023a). Flat-slab conveyor effect induces precursory crustal contraction in the Central Andes (preprint Research Square). Pons, M., Rodriguez Piceda, C., Sobolev, S., Scheck-Wenderoth, M., & Strecker, M. (2023b). Localization of deformation in a non-collisional subduction orogen: the roles of dip geometry and plate strength on the evolution of the broken Andean foreland, Sierras Pampeanas, Argentina (preprint Essoar). Rodriguez Piceda, C., Scheck Wenderoth, M., Gomez Dacal, M. L., Bott, J., Prezzi, C. B., & Strecker, M. R. (2021). Lithospheric density structure of the southern Central Andes constrained by 3D data-integrative gravity modelling. International Journal of Earth Sciences, 110(7), 2333-2359. Rodriguez Piceda, C., Scheck-Wenderoth, M., Bott, J., Gomez Dacal, M. L., Cacace, M., Pons, M., ... & Strecker, M. R. (2022a). Controls of the lithospheric thermal field of an ocean-continent subduction zone: the southern Central Andes. Lithosphere, 2022(1), 2237272. Rodriguez Piceda, C., Scheck‐Wenderoth, M., Cacace, M., Bott, J., & Strecker, M. R. (2022b). Long‐Term Lithospheric Strength and Upper‐Plate Seismicity in the Southern Central Andes, 29°–39° S. Geochemistry, Geophysics, Geosystems, 23(3), e2021GC010171. Tassara, A., Götze, H. J., Schmidt, S., & Hackney, R. (2006). Three‐dimensional density model of the Nazca plate and the Andean continental margin. Journal of Geophysical Research: Solid Earth, 111(B9). Van Zelst, I., Crameri, F., Pusok, A. E., Glerum, A., Dannberg, J., & Thieulot, C. (2022). 101 geodynamic modelling: how to design, interpret, and communicate numerical studies of the solid Earth. Solid Earth, 13(3), 583-637.