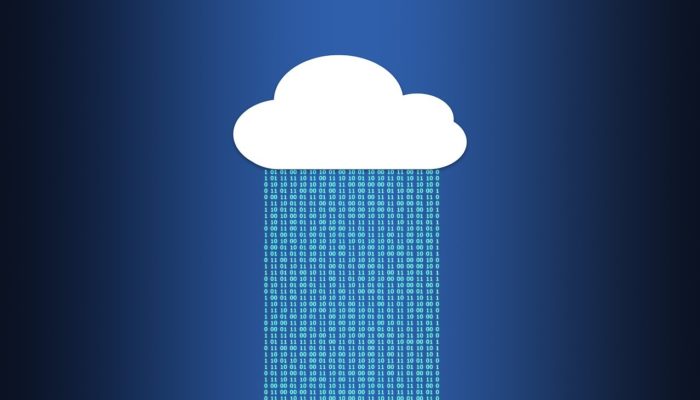
If I asked you about what your carbon footprint is, your mind might jump to the food choices you make when at the supermarket, or how many conferences you fly to when you could get a train (well, not now, but you know, back in ye olden days).
In this week’s post, Eoghan Totten, a PhD student at the University of Oxford, discusses the potential “hidden” impacts on your contribution to CO2 emissions from something rather mundane that you might be overlooking…computing!
Geoscience is rooted in observation. Quite often, a great deal can be learned about solid Earth processes from a rather modest dataset. It could be argued that, in the current era of high performance computing and big data, geoscience has never been better positioned to evolve as a discipline. My own PhD research (which focuses on creating seismic velocity models of the mantle) directly benefits from being able to process large datasets on high performance computing resources. However, I am aware that this takes place against the general global backdrop of ever-increasing CO2 emissions from cloud computing and data warehousing.
Recently, I read an astonishing figure that it is predicted that up to 29% of electricity consumption in Ireland (my country of origin) will be used by data centres by 2028 (Boran, 2020). This reflects the exponential and global growth of data science and artificial intelligence, which will likely play an integral role in post-COVID economic recovery. While the associated energy consumption will be primarily driven by the commercial sector, research will undoubtedly play a role. This made me wonder, how can we mitigate research-related CO2 production?
Before I move on to telling you what steps we [the geoscience community] might take to reduce our carbon footprint, I will hold my hands up and say that I am very far from being an expert in this area. Perhaps that is why I have written this article in the first place. I want to learn what is the right thing to do as a researcher, going forward. While I think that it is advisable to be carbon-conscious as scientists, I am also aware that each research project is unique. CO2 emissions incurred are often warranted if significant results are obtained, in which case the latter should always take precedence.
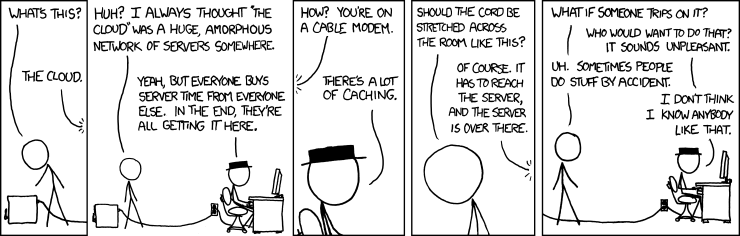
Image credit: xkcd.com
Carbon-concious coding
Programming is no longer incidental to the day-to-day work of most geoscience research projects. In many cases, it now plays a central role. As highlighted in a previous article, many PhD students already undergo an initial steep learning curve in order to upskill in programming. One possible option going forward is that future generations of PhD students and PDRAs undertaking computationally-intensive projects could be given the opportunity to upskill in software engineering, prior to beginning their research. This could kickstart a butterfly effect i.e. if the general body of new codes being written are on average more efficient, research computing CO2 emissions are likely to be lower.
Encouraging researchers to understand and make decisions regarding their CO2 emissions would, in the medium to long term, promote a culture of carbon-conscious coding, where researchers are mindful of questions such as:
- What is the most efficient programming language available to me, in order to achieve my research goals, based on my current skill set? If there is a more efficient programming language available, with which I have no experience, should I consider learning to use it?
- How much data am I likely to produce over the life cycle of my project?
- What are my options for storing this data securely and what is the least carbon-intensive means of doing so?
- If I am using cloud storage, am I able to find out how much brown energy (non-renewable) is used to power those resources? Are there alternatives?
- If I am running simulations, is it possible to run these at coarser resolutions (using reduced computing resources) and still achieve the desired outcome?
- If my jobs are set to be run on a supercomputer, what is the optimal trade-off between the number of processors used and time to solution (and how can I find out this information)?
Learning from other communities
Discussion around the environmental impact of research-based computing already appears to be taking place in other research communities. For example, Zwart (2020) notes that astrophysicists can significantly reduce their carbon footprint by increasing the use of GPU processors in the coming years, wherever possible.
Arguably, the particle physics community has achieved the gold standard when it comes to large-scale collaboration. For example, the ATLAS experiment, entails merging the research efforts of 1200 doctoral students across 38 countries. The tiered sharing and warehousing of data across the collaboration likely saves on CO2 emissions, compared to 1200 research students working in isolation.
Part of the success of the machine learning and AI research communities is due to its streamlined data processing, sometimes referred to as the Data Science Lifecycle (e.g. Siva, 2020). This guides workflow development and promotes development efficiency which, in itself, is likely to make a positive impact. Arguably, the rapid rise of AI/ML is already percolating into how the general research community processes its data e.g. the widespread use of Pandas data frames in Python. Perhaps this bodes well for the future.
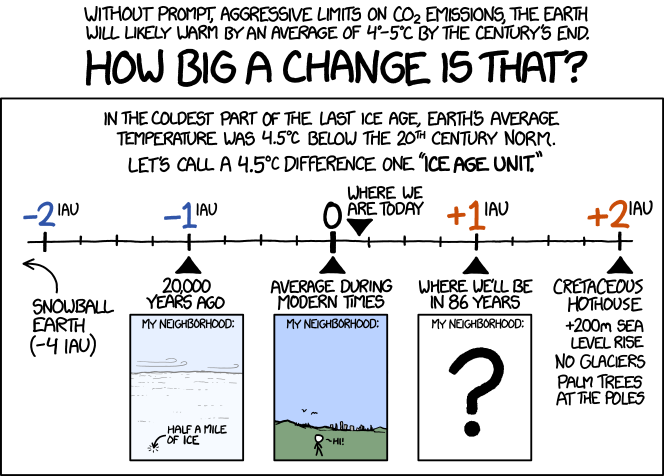
Image credit: xvdf.com
Growing community knowledge and incentivising CO2 reduction
This blog barely scratches the surface of the many possible ways to reduce the carbon footprint of research-related computing. In my opinion, there is a unique opportunity for the geoscience community to collaborate on this issue. A significant portion of its research already contributes directly or indirectly to climate change and sustainability efforts (e.g. oceanography/carbon sequestration). Formalising such a collaboration would be in harmony with these efforts.
Significant and measurable change often comes about by realignment of habits in a community (if a global pandemic has taught me anything, it has taught me that this is possible and in a short space of time). One way to move forward as a community is to create a blueprint or set of guidelines, for research-based computing and data storage, with sufficient visibility and outreach to make a measurable impact. Furthermore, carbon-conscious computing could be incentivised at the publication stage, by using a new set of carbon-conscious metrics or internationally recognised seals of approval.
Further Reading: Boran, M. (2020). ‘The true carbon cost of feeding the data centre monster’, Irish Times, 30 April, 2020. Available at: https://www.irishtimes.com/business/technology/the-true-carbon-cost-of-feeding-the-data-centre-monster-1.4236923 Siva, S. (2020). The Generic Data Science Life Cycle. Available at: https://towardsdatascience.com/stoend-to-end-data-science-life-cycle-6387523b5afc Zwart, S.P. (2020). The ecological impact of high-performance computing in astrophysics. Nature Astronomy, 4(9), pp.819-822.
brahim d staps
Thank you for sharing
ferahtia-fs
thanks I read the post
ferahtia.FS
Very interesting post. This is my first time visit here. I found so many interesting stuff in your blog especially its discussion. Thanks for the post!
aree
Believe it or not, it is the type of information I’ve long been trying to find. It matches to my requirements a lot. Thank you for writing this information.
ahmed staps
The work you are doing is very distinguished, as long as you are successful