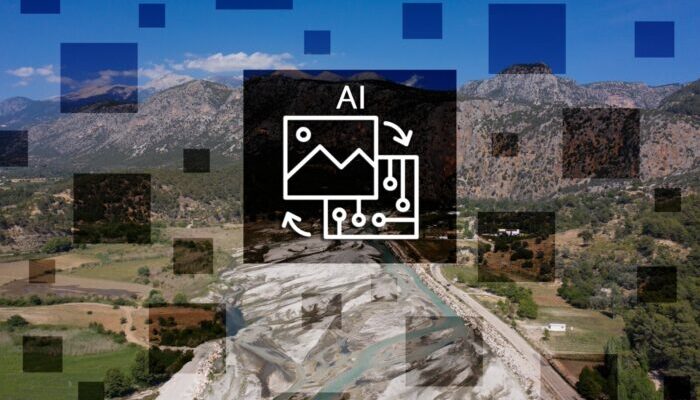
In recent decades, AI-based methods have increasingly been adopted to tackle various problems in the field of natural hazards. The escalation of climate change has fuelled the complexity of tasks within the field of disaster risk reduction, such as capturing the formation of an extreme event timely to evacuate an area at risk. In this context, with the greater availability of data and computerised methods, we have had the application of mostly machine learning and deep learning methodologies as great allies for obtaining rapid responses to better understand the dynamics of natural phenomena. However, the emergence of foundation models could represent an even more significant leap forward.
As a first note, AI-based methods and their applications for disaster risk management were the focus of a 2021 blog post titled ”Artificial intelligence for disaster management: that’s how we stand”; here, we will dive into the advances. Foundation models represent a pivotal advancement in Artificial Intelligence (AI), embodying large-scale neural networks trained on extensive datasets. These models serve as the bedrock of contemporary deep learning, encompassing a wealth of knowledge and offering an adaptable and scalable AI solution. The foundation is established by integrating diverse and huge data sources into these neural networks, allowing for a broad understanding of various domains. Types of foundation models include Large Language Models (LLM), Visual Models for interpreting and generating images, Scientific Models for tasks, such as predicting proteins in biological processes, and Audio Models [1].
How can we adapt this vast knowledge of a foundation model for specific domains?
Now, visualise these models as the building blocks for understanding complex tasks like predicting climate change. But how is that possible? One notable manifestation of this paradigm is the Transformer architecture, which forms the base – for example – the geospatial climate models. These foundation models are then adapted to create specialised models for specific applications (i.e, creating maps for natural hazard propensity), a process named fine-tuning. The fine-tuning process involves supplementing the model with examples of specific use cases, creating a bridge between vast datasets and real-world challenges. Another interesting technique is prompt tuning, in which you can tailor the massive data trained into a specific task. Prompt tuning guides the model towards the desired specific output, exemplified by generating a “landslide hazard map for the German Alps”. Nevertheless, foundation models are just the basis, and the idea here is to create content. Thus, Generative AI enters as a tool that takes this knowledge and crafts entirely new content.
Summing up, whilst foundation models provide structure and understanding, Generative AI produces new content that emerges from the vast knowledge coming from Foundation models. For instance, by training climate-related variables through Generative AI, it is possible to generate maps illustrating climate change projections for specific time periods and locations.
Advantages for disaster risk reduction
In the sphere of natural hazards, machine learning and deep learning methods have been widely employed to develop predictive models [2]. Nevertheless, challenges arise in acquiring comprehensive data, such as a complete landslide inventory or suitable spatial products, hindering the use of more sophisticated methods and impacting the quality of predictions.
Within the disaster risk reduction framework, foundation models confer an advantage by surmounting these data limitations and labour-intensive tasks. They streamline the handling of extensive datasets essential for predictive modelling, thereby enhancing the precision of hazard assessments. Jakubik et al. [3] demonstrated the effectiveness of unsupervised domain adaptation in improving U-Net models for natural hazard segmentation, enhancing generalisability across diverse regions and hazards. This approach supports near real-time mapping, addressing the challenges of deep learning models designed for specific tasks in single regions [3].
Regarding applicability, a good example is that a foundation model can be fine-tuned to predict future flood risks (Fig 1) by analysing past flood-prone areas. Therefore, generating a natural hazard susceptibility map would be possible by instructing a prompt to do all this work. In addition, the vast knowledge base and amount of data are also advantages for dealing with problems that computerised methods struggle with. While traditional machine learning models are limited in dealing with climatic variables and their interactions, the difficulty of capturing extreme events with rapidly forming characteristics is made more feasible by foundation models. In this context, achieving the ability to foresee an extreme event to the extent that we can make decisions to prevent fatalities represents a significant milestone in boosting disaster risk reduction strategies, such as identifying climate-proof spaces, reducing vulnerabilities and increasing resilience. This iterative process enhances the adaptability and effectiveness of foundation models in addressing diverse challenges.
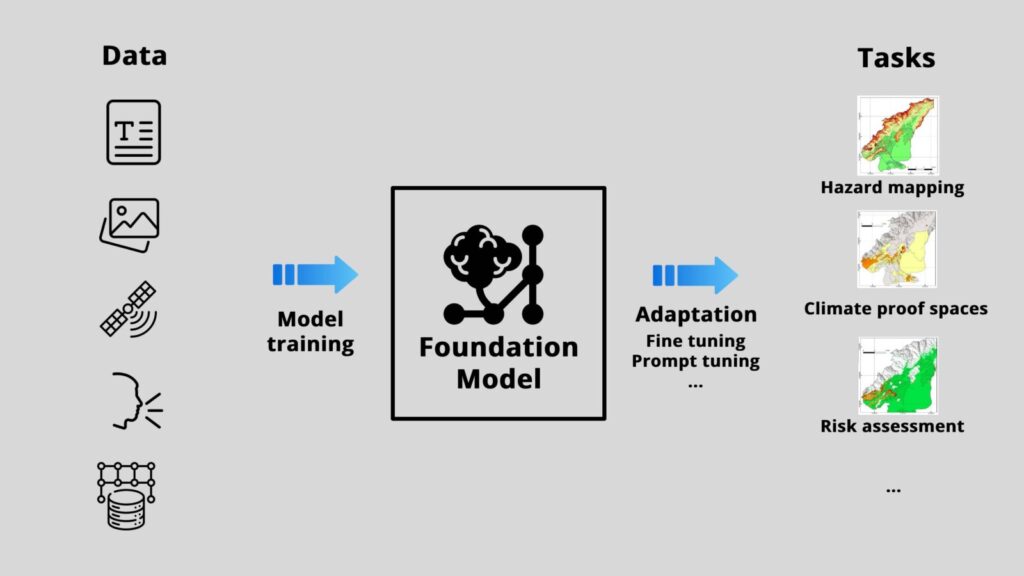
Fig. 1 – Workflow of a foundation model with outputs for geospatial/climate data (photo credit: Paulo Hader, source of icons: https://www.flaticon.com)
Scientific Rigour with Explainable AI
To ensure transparency and scientific rigour, exploring Explainable AI is crucial. This allows us to scrutinise the interactions behind decisions made by foundation models and Generative AI, providing a deeper understanding of the processes driving predictions and content generation. As foundation models become the cornerstone of a new era in AI, their integration with Explainable AI ensures a reliable and accountable approach to addressing the impact of natural hazards.
EGU General Assembly Session about Generative AI in April 2024
Notably, the topic of Generative AI has already garnered significant interest within the natural hazard scientific community, as evidenced by its inclusion as a focal point in the “Towards AI enhanced modelling of Natural Hazards: Exploring the Power of Generative AI Chatbots“ at the EGU General Assembly in 2024. This notable presence in a dedicated session underscores the growing recognition of the importance and potential impact of Generative AI in advancing the field of natural hazard modelling.
References
[1] Paaß, G., Giesselbach, S. (2023). Foundation Models for Speech, Images, Videos, and Control. In: Foundation Models for Natural Language Processing. Artificial Intelligence: Foundations, Theory, and Algorithms. Springer, Cham. https://doi.org/10.1007/978-3-031-23190-2_7
[2] Abdelaziz Merghadi, Ali P. Yunus, Jie Dou, Jim Whiteley, Binh ThaiPham, Dieu Tien Bui, Ram Avtar, Boumezbeur Abderrahmane (2020). Machine learning methods for landslide susceptibility studies: A comparative overview of algorithm performance, Earth-Science Reviews. https://doi.org/10.1016/j.earscirev.2020.103225
[3] Jakubik, J., Muszynski, M., Vössing, M., Kühl, N., & Brunschwiler, T. (2023). Toward Foundation Models for Earth Monitoring: Generalizable Deep Learning Models for Natural Hazard Segmentation. https://doi.org/10.48550/arXiv.2301.09318
Post edited by Asimina Voskaki and Hedieh Soltanpour