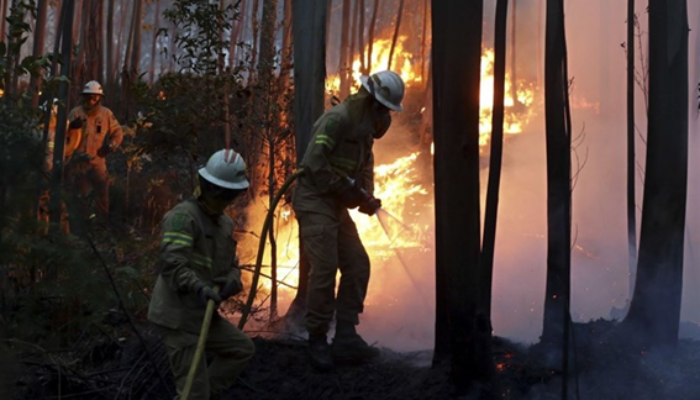
In the last decades, the strength of climate change has been evident across the globe in many weather and climate extremes occurrences, including heatwaves and droughts. Those events are involved in all fire stages and influence all aspects of the fire regime [1].
Climate change is driving unprecedented wildfire in the Mediterranean region
The Mediterranean region is a climate change hotspot characterised by a severe decrease in precipitation (40% less in winter) and significant warming with peaks in summer. Additionally, since the 50s, the frequency of droughts has shown notable increases in this region [2]. In addition, droughts are projected to increase their spatial extent between 10 to 60% in the future [3]! On the other hand, the magnitude of the warming is projected to increase year by year, which will contribute to the increase in the occurrence of extremely high-temperature events, such as heatwaves. This trend toward drier conditions has been explained as the result of the combined effect of two phenomena: a change in the dynamics of upper atmosphere circulation and a decrease in the temperature difference between land and sea [4]. In many ecosystems, the impacts of climate change act as a disturbance variable that, in the long term, may result in an increase in tree mortality, drastic changes in soil properties, and fine fuel dryness. These concomitant conditions will increase the likelihood of larger and more intense wildfires in the Mediterranean region!
Catastrophic wildfires in the Mediterranean
In the Mediterranean region, there has been an increase in large and untamed wildfires with huge socioeconomic and environmental impacts, including the potential loss of human lives [5]. For instance, in Portugal in 2017, there were catastrophic wildfires that occurred in only two months (Figure 1). During October, the biggest pyro-convective phenomenon of the year occurred worldwide, with an average of ten thousand hectares burnt per hour in only 13 hours [5].
Globally, the 2017 events caused a record of 500 thousand burnt hectares, largely consuming forest (90% occupied by Eucalyptus spp. and Pinus Pinaster), followed by agricultural areas, shrublands, and artificial surfaces. The greatest tragedy is that 112 people between 5 and 88 years old lost their lives!
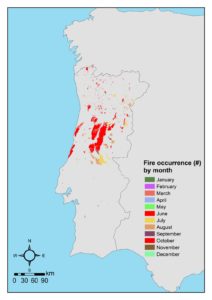
Figure 1 – Fire occurrence in Portugal in 2017 by month. Credit: Joana Parente
What we saw in Portugal in 2017 is what researchers in fire behaviour call a “plume dominated wildfire”, the most dangerous for Europe due to its unpredictability in terms of when the event will occur and where it will go [6]. Comparable with this fire season, but not so severe, were the wildfires of 1994 in Spain (burnt area of 146 thousand hectares), of 2003 in Portugal (burnt area of almost 100 thousand hectares), and of 2007 in Greece (burnt area of 63 thousand hectares that burnt during 6 days). Such events imply pressure upon forest management and firefighting: it is now a matter of national security and civil protection!
Machine Learning: a new tool for fire risk assessment
A key tool to undertake prevention and planning programs is certainly the implementation of accurate wildfire inventories, ideally including the spatial locations of wildfires, eventually as mapped burnt areas, and the starting and ending dates. The traditional approaches for burnt area delimitation, based on ground observations, have been progressively replaced by satellite products, ensuring a more consistent assessment over time [7].
In recent studies, machine learning (ML) techniques have proven to be successful in mapping and dating daily burned areas using high-resolution images [8]. The use of ML in fire research is rapidly advancing, not only for events detection and mapping but also for fire susceptibility and risk assessment, fuels characterisation, fire weather and climate change, fire impacts and fire management [9]. These techniques are generally emerging as a new paradigm in geosciences: they are capable of discovering the hidden relationships between the hazard events and the environmental, meteorological and anthropogenic complex drivers allowing us to make predictions, in terms of probabilistic outputs (Figure 2) [10].
The main benefit of using ML compared to expert and physical-based models is that the former is data-driven – meaning that it does not need a priori knowledge of the process – which can be useful in a rapidly changing world (for example, changes in land use/land cover, climate changes). Finally, predictions in ML are meaningful to elaborate wildfire susceptibility maps, indicating the potential to experience a particular hazard in the future based on past observations. This outcome is extremely useful for decision-makers in wildfire management and long-term land use planning. For example, ML-based susceptibility maps are adopted operationally by the Italian Civil Protection in early warning systems for wildfire danger.
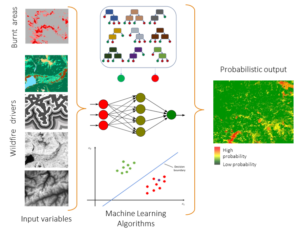
Figure 2 – Methodological flowchart for wildfire susceptibility mapping using Machine Learning (ML) based approaches. The three ML algorithms represented in this generic scheme are random forest, artificial neural network, and support vector machine (from top to down). Credit: Marj Tonini
Finally, we would point out that in fire risk assessment and predictive mapping, most of the literature focuses on the static probability of experiencing an event in a certain area as a function of the intrinsic characteristics of the territory, overlooking the dynamic effect of the weather conditions, such as wind spread and direction, precipitations, temperature, heatwaves, fuel moisture content, and, for long time series, climate changes. Clearly, this gap needs to be filled in further research that should also take into account the dynamic effect of local and global climate conditions.
Wildfires at #EGU23
In an era of climate change, wildfires need to be characterised and understood for future fire management, and #EGU23 wants to contribute to it with several dedicated thematic sessions: NH7.1 seeks to improve our knowledge of wildfire spatio-temporal dynamic, fire regime, and strategies to mitigate the disastrous effects of wildfire; NH7.2 explores the changing meteorological and climatological dynamics of extreme wildfires along with their impacts on societies and ecosystems; BG1.2 explores the role of fire in the Earth system at any temporal and spatial scale using modelling, field and laboratory observations; SSS9.11 aims at gathering researchers on the effects of wildfires on ecosystems, from fire prevention to post-fire mitigation.
Edited by Asimina Voskaki and Soheil Mohammadi
References
[1] Pereira, M. G., Parente, J., Amraoui, M., Oliveira, A., & Fernandes, P. M. (2020). The role of weather and climate conditions on extreme wildfires. In Extreme Wildfire Events and Disasters (pp. 55-72). Elsevier. https://doi.org/10.1016/B978-0-12-815721-3.00003-5.
[2] IPCC. (2021). Climate Change 2021: The Physical Science Basis. Contribution of Working Group I to the Sixth Assessment Report of the Intergovernmental Panel on Climate Change [Masson-Delmotte, V., P. Zhai, A. Pirani, S.L. Connors, C. Péan, S. Berger, N. Caud, Y. Chen, L. Goldfarb, M.I. Gomis, M. Huang, K. Leitzell, E. Lonnoy, J.B.R. Matthews, T.K. Maycock, T. Waterfield, O. Yelekçi, R. Yu, and B. Zhou (eds.)]. Cambridge University Press, Cambridge, United Kingdom and New York, NY, USA, 2391. Available from: https://www.ipcc.ch/report/ar6/wg1/.
[3] EEA. Climate change, impacts and vulnerability in Europe 2016: An indicator-based report [Internet]. 2017. Available from: https://www.eea.europa.eu/publications/climate-change-impacts-and-vulnerability-2016.
[4] Tuel, A., & Eltahir, E. A. (2020). Why is the Mediterranean a climate change hot spot? Journal of Climate, 33(14), 5829-5843. https://doi.org/10.1175/JCLI-D-19-0910.1.
[5] Comissão Técnica Independente, Guerreiro, J., Fonseca, C., Salgueiro, A., Fernandes, P., Lopez Iglésias, E., de Neufville, R., Mateus, F., Castellnou Ribau, M., Sande Silva, J., Moura, J., Castro Rego, F., & Caldeira DN. (2018). Avaliação dos incêndios ocorridos entre 14 e 16 de outubro de 2017 em Portugal Continental. Relatório Final. Comissão Técnica Independente. Assembleia Da República. Lisboa. Available from: Avaliação dos Incêndios ocorridos entre 14 e 16 de outubro de 2017 em Portugal Continental – XXI Governo – República Portuguesa.
[6]Castellnou, M., Guiomar, N., Rego, F., & Fernandes, P. M. (2018). Fire growth patterns in the 2017 mega fire episode of October 15, central Portugal. In Advances in forest fire research 2018 (pp. 447–453). Imprensa da Universidade de Coimbra. https://doi.org/10.14195/978-989-26-16-506_48.
[7] Chuvieco, E., Mouillot, F., van der Werf, G. R., San Miguel, J., Tanasse, M., Koutsias, N., García, M., Yebra, M., Padilla, M., Gitas, I., Heil, A., Hawbaker, T. J. and Giglio, L. (2019). Historical background and current developments for mapping burned area from satellite Earth observation. Remote Sens. Environ., 225, 45–64. https://doi.org/10.1016/j.rse.2019.02.013.
[8] Pinto M.M., Libonati R., Trigo R.M., Trigo I.F., DaCamara C.C. (2020): “A deep learning approach for mapping and dating burned areas using temporal sequences of satellite images”. ISPRS Journal of Photogrammetry and Remote Sensing, 160, 260-274. https://doi:10.1016/j.isprsjprs.2019.12.014.
[9] Piyush Jain, Sean C.P. Coogan, Sriram Ganapathi Subramanian, Mark Crowley, Steve Taylor, and Mike D. Flannigan (2020). A review of machine learning applications in wildfire science and management. Environmental Reviews. 28(4): 478-505. https://doi.org/10.1139/er-2020-0019.
[10] Trucchia, A.; Izadgoshasb, H.; Isnardi, S.; Fiorucci, P.; Tonini, M. Machine-Learning Applications in Geosciences: Comparison of Different Algorithms and Vegetation Classes’ Importance Ranking in Wildfire Susceptibility. Geosciences 2022, 12, 424. https://doi.org/10.3390/geosciences12110424.