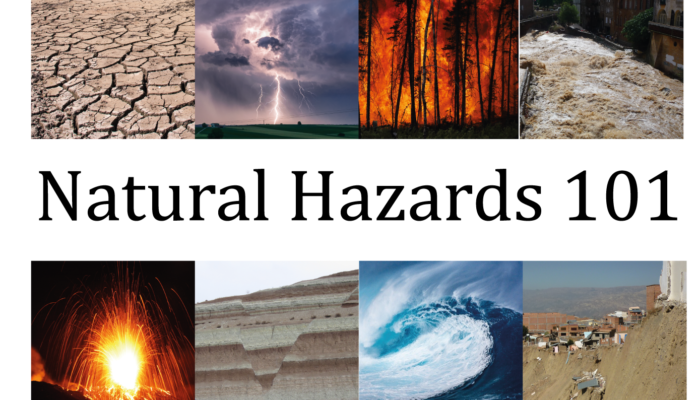
With the Natural Hazards 101 series, we mean to bring our readers closer to the terminology often used in the field of natural hazards, but that may not be so familiar.
In the first episode of the series, we focused on the definition of hazard and natural hazard. We moved then to the concept of risk, which brought us to define exposure and vulnerability. Later on, we digested the disaster terminology provided by the United Nations Office for Disaster Risk Reduction. In this episode, we will explore the meaning of “Forecasting and modelling” natural hazards.
It is hard to find an official or commonly accepted definition of “Natural hazard modelling” since models can be applied in different scientific fields besides natural hazards and geosciences. If you search on the web, it is easy to find that modelling (and simulating), in science, is defined as a scientific activity aimed to make a phenomenon easier to understand, define, quantify, visualize, or simulate by referencing it to existing and usually commonly accepted knowledge. All models are thus reflections of reality that, despite being approximations, can be extremely useful [1].
Models are typically used when it is either impossible or impractical to create experimental conditions in which scientists can directly measure outcomes. They are generally cheaper, safer and sometimes more ethical than conducting real-world experiments. Simulations can often be performed faster than the real-time phenomena they describe [2].
Scientific models can be divided in:
Physical models: smaller and simpler representations of the phenomenon or object being studied. A globe or a map is a physical model of a portion or all of Earth.
Mathematical models: sets of equations that take into account many factors to represent a phenomenon. Mathematical models are usually done on computers. Among them, statistical models based on Machine Learning algorithms are currently finding a growing application.
Concerning natural hazards, GNS Science provides a synthetic description of their geohazard modelling activity [3]. This activity can be representative of the typical approach adopted by scientists to model natural hazards.
- Earthquake Hazard Modelling: they model strong quakes to determine and design for the severity and characteristics of strong ground shaking.
- Landslide Hazard Modelling: they forecast the magnitude and frequency of new and pre-existing landslides in response to event scenarios (for example, rainstorms or earthquakes).
- Tsunami Hazard Modelling: they use hydrodynamic models that provide quantitative estimates of the propagation of tsunami waves away from their source and their impact when they reach the coast.
- Volcano Hazard Modelling: they provide a dynamic assessment of the likelihood of size, frequency and location of future eruptions, based on the current level of volcanic unrest, coupled with analysis of past historic and geologic data.
In the context of the disaster cycle, as described in the latest episode of the series, natural hazards modelling can be considered a non-structural measure that contributes to mitigating risk and developing preparedness. Natural hazards modelling is also at the basis of disaster risk assessment. The UN Office for Disaster Risk Reduction (UNDRR) describes modelling as a qualitative or quantitative approach to determine the nature and extent of disaster risk, including identifying potential hazards and their characteristics, such as their location, intensity, frequency and probability [4].
Finally, natural hazards modelling is propaedeutic to natural hazards forecasting. The latter is a complex science that aims to determine where, when, and how intensively the next natural hazard event will occur. Scientists attempt to predict natural hazards and their consequences using a variety of approaches, which can be loosely grouped into two broad categories: theoretical (mechanistic, deterministic, physically based, “hard”) and empirical (statistical, functional, “soft”) approaches. Unlike other natural phenomena, natural hazards are difficult to predict since they are typically chaotic or exhibit distinct nonlinear behaviours, complicating the possibility of using past occurrences to predict future events [5]. However, low predictability is different from unpredictability [6], and efforts should be made to improve our ability to forecast natural hazards and determine and communicate the uncertainties of such forecasts [5].
References
[1] https://en.wikipedia.org/wiki/Modeling_and_simulation
[2] https://en.wikipedia.org/wiki/Scientific_modelling
[4] https://www.undrr.org/terminology/disaster-risk-assessment
[5] Guzzetti, F. (2016). Forecasting natural hazards, performance of scientists, ethics, and the need for transparency. Toxicological & Environmental Chemistry, 98(9), 1043-1059. https://dx.doi.org/10.1080%2F02772248.2015.1030664
[6] Stein, J. L., & Stein, S. (2014). Gray swans: comparison of natural and financial hazard assessment and mitigation. Natural hazards, 72(3), 1279-1297. https://dx.doi.org/10.1007/s11069-012-0388-x
Read episode 1: What is a -natural- hazard?
Read episode 2: The concept of risk.
Read episode 3: The disaster cycle.