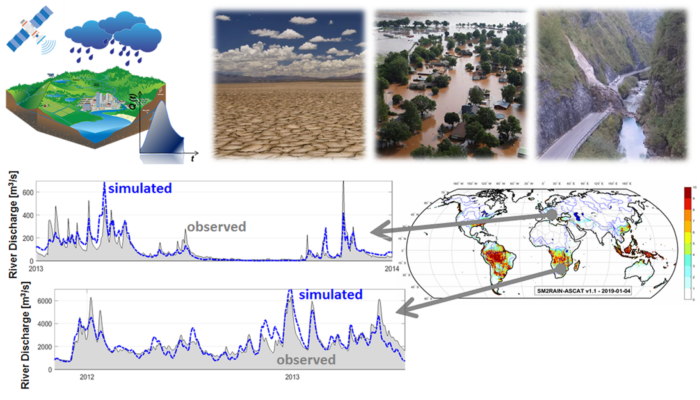
Soil moisture and rainfall are the two fundamental variables in the water and energy cycle and their knowledge in many applications is crucial. For instance, for predicting the occurrence and the magnitude of flood and landslide events the knowledge of the initial soil moisture condition and of rainfall amount is mandatory.
In the last decade, some authors have proposed a completely new approach, i.e., the use of satellite soil moisture for estimating and improving rainfall prediction, doing hydrology backwards. In 2014, we have published a study showing, for the first time, that we can estimate rainfall on a global scale using satellite soil moisture observations [1], i.e., using the soil as a natural rain gauge. The algorithm used for estimating rainfall is called SM2RAIN, i.e., Soil Moisture to RAINfall, and in the last 7 years, we have been performing a number of studies and research projects on this topic.
Currently, we, i.e., the Hydrology group of the Research Institute for Geo-Hydrological Protection at Italian National Research Council (CNR-IRPI), have made available three SM2RAIN-derived satellite rainfall products exploiting different satellite soil moisture products: SM2RAIN-CCI [4] from 1998 to 2015, SM2RAIN-ASCAT [2] from 2007 to 2019, and GPM+SM2RAIN [8] from 2015 to 2018. The three products have been developed under research projects funded by the European Space Agency (ESA) and the European Organisation for the Exploitation of Meteorological Satellites (EUMETSAT), they are freely available on Zenodo and characterized by different characteristics and quality level.
The assessment of SM2RAIN-based products on a global scale has revealed an important result [2], [8] i.e., satellite soil moisture improves state of the art rainfall products exactly over regions where the data are needed most: Africa and South America. Indeed, in these regions ground observations are lacking and hardly available; satellite rainfall datasets are crucial for flood and landslide prediction.
Animation of global rainfall for the year 2019 obtained with SM2RAIN-ASCAT dataset.
Animation of monthly rainfall from June 2016 to July 2018 with SM2RAIN-ASCAT dataset. Credit: Luca Brocca.
Why do we need a new satellite rainfall product?
You are probably wondering why a new satellite rainfall product? Do the new products based on the SM2RAIN concept add value (if any) to hydrological (or others) applications? Which gap can be filled with SM2RAIN-based rainfall products? For answering these questions, we have started using such products for river flood prediction in Italy [4], in the Mediterranean [5], [7], in Europe (Camici et al., 2020, under review) and in Africa, also with the work of colleagues who tested the products in other regions and globally, e.g. [6], [9].
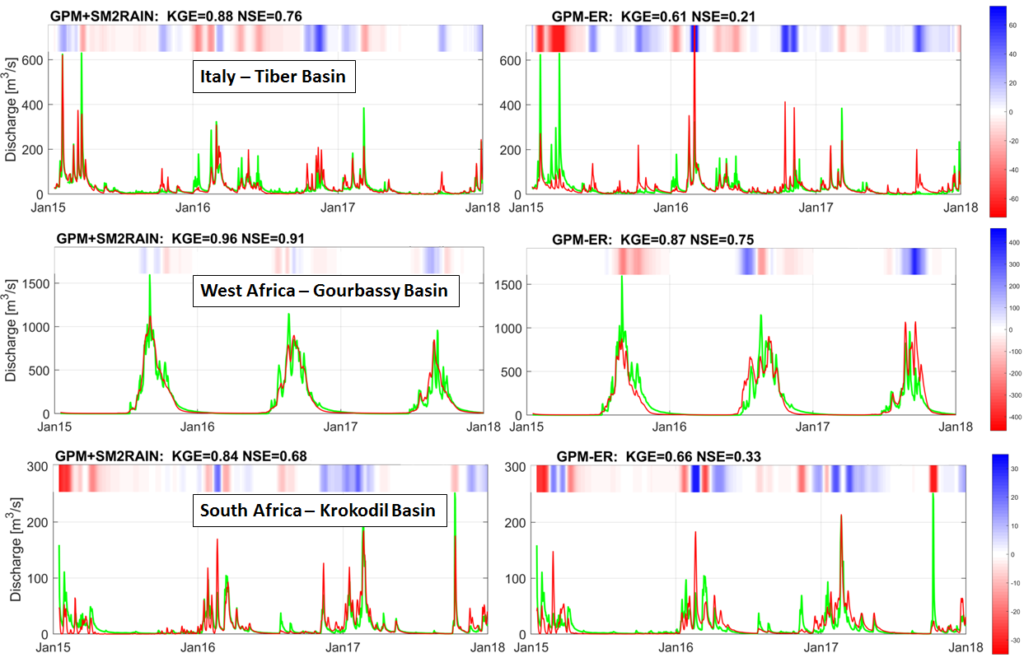
Testing satellite rainfall products for daily river flow prediction in three basins in Italy (top), West Africa (middle) and South Africa (bottom) by using MISDc rainfall-runoff model. The two satellite rainfall products are GPM+SM2RAIN (left) and GPM (Global Precipitation Measurement) Early Run, GPM-ER (right) [KGE= Kling-Gupta Efficiency; NSE=Nash-Sutcliffe Efficiency] The optimal value for KGE and NSE is 1. Therefore, the closer KGE (NSE) to 1, the better is the satellite rainfall product for river flow prediction.
Results indicate that SM2RAIN-based products perform better than classical satellite rainfall products (e.g., the Early Run version of the Integrated Multi-Satellite Retrievals for Global Precipitation Measurement, GPM, mission, IMERG-ER) for river flow prediction in most of the investigated basins. Why? It should be attributed to the different nature of the measurement provided by satellite soil moisture data; with SM2RAIN, we estimate the accumulated rainfall between two satellite overpasses without losing water (with accurate soil moisture data, of course). Differently, classical microwave-based products provide instantaneous measurements and hence errors might occur if satellite overpasses do not coincide with the time of rainfall occurrence. Indeed, such systems require a constellation of sensors to provide frequent measurements while with SM2RAIN a single satellite, if accurate, is able to provide reliable measurements of accumulated rainfall.
Hydrology has memory, for predicting a flood today you need to accurately know the history of rainfall in the last weeks or months depending on the size of the investigated basin, not only today rainfall. Therefore, satellite measurements of rainfall should be accurate in estimating the “history” of rainfall, not only during flood events, and SM2RAIN-based products do a good job to address this target!
Promising results for river flow prediction…and for landslides?
And…what is the reliability of SM2RAIN-based products for landslide forecasting? Our colleagues from the Geomorphology group at CNR-IRPI have collected a catalogue of 1400+ landslides occurred in Italy in the last 10 years. Such dataset is crucial for assessing the quality of different satellite rainfall products in predicting landslide occurrence, and we have published the results of this interesting analysis in 2018 [3]. Results confirmed what we have found for river flow prediction, i.e., SM2RAIN-based product is performing the best among the different products with results slightly worse than using in situ observations.
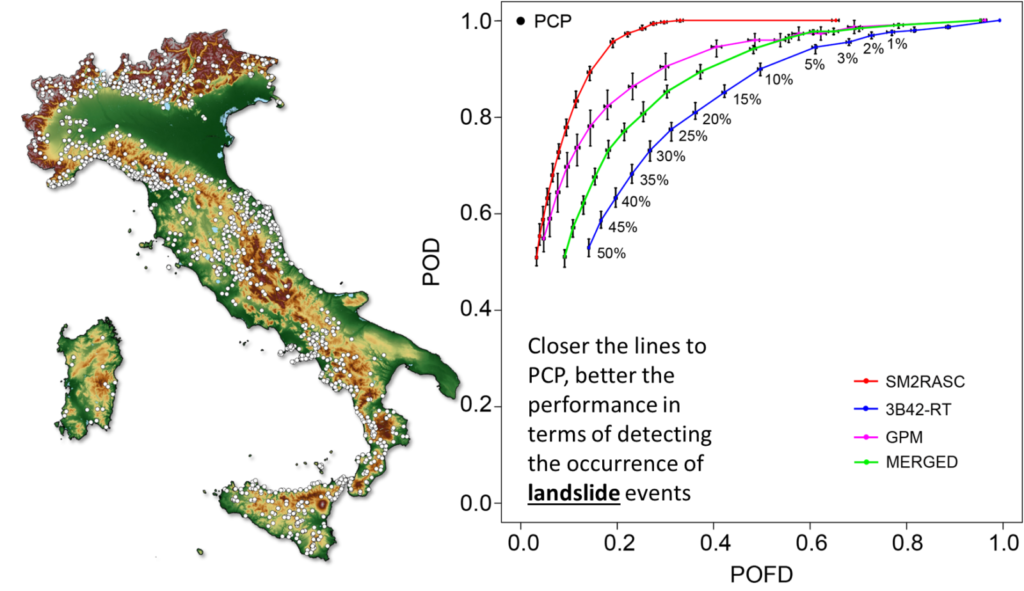
Italian catalogue of landslide events (left) and Receiver Operating Characteristic (ROC) curve (right) for assessing the capability of different satellite rainfall products in predicting landslide occurrence in Italy [POFD: Probability Of False Detection, POD: Probability Of Detection]. Closer the lines to PCP (Perfect Classification Point), the better the satellite rainfall product is (adapted from Brunetti et al., 2018).
Maybe, the current spatial (12 km) and temporal (1-day) resolution are not considered adequate for hydrological applications from many users, indeed we are moving forward to improve them. In the FFG (the Austrian Research Promotion Agency) funded DWC-Radar project, we intend to develop a daily and 500 m rainfall product through the integration of Sentinel-1 and ASCAT soil moisture data throughout the Mediterranean basin. Interesting results could also derive from using new technologies, such as Global Navigation Satellite Systems – Reflectometry (GNSS-R) that will provide higher spatial and temporal resolution in our satellite products. A particular interest is devoted to the ESA Earth Explorer 10 Candidate Mission Hydroterra (previously named G-Class) which is a radar satellite to be placed in geosynchronous orbit with a continuous view of Europe and Africa. Through Hydroterra, high resolution in space (<1 km) and time (<1 hour) will be obtained thus transforming and further improving how we understand the water cycle and how we will estimate rainfall through SM2RAIN.
To conclude, we would like to underline that “an exciting activity in the last 7 years have characterized the development of the SM2RAIN algorithm and its exploitation for natural hazard applications, but a more exciting future is expected to come.”
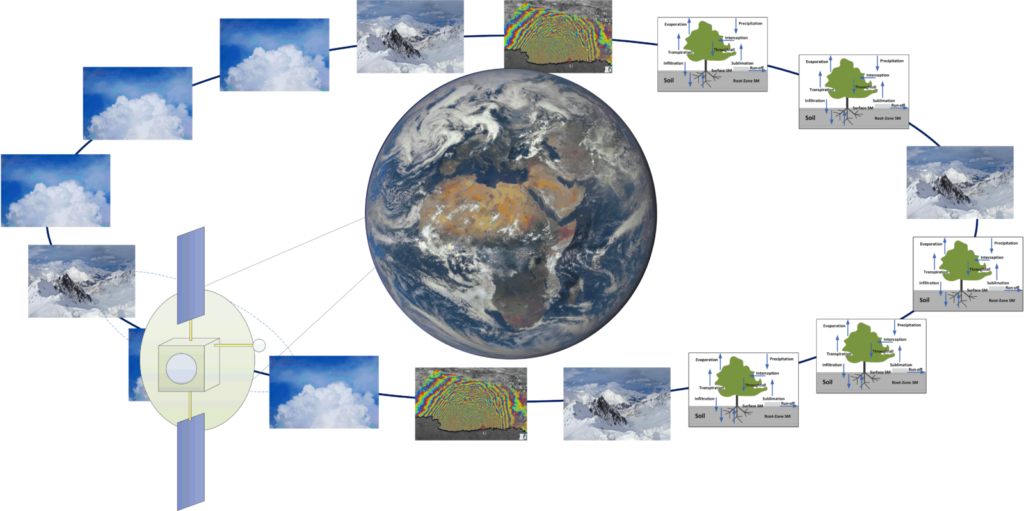
An overview of the Hydroterra mission concept: a satellite in geosynchronous orbit has a permanent view of Earth’s disk and can image when and wherever desired over Europe and Africa, thus making repeated observations of a range of phenomena and at different temporal sampling – as appropriate to each application.
References
- Brocca, L., Ciabatta, L., Massari, C., Moramarco, T., Hahn, S., Hasenauer, S., Kidd, R., Dorigo, W., Wagner, W., Levizzani, V. (2014). Soil as a natural rain gauge: estimating global rainfall from satellite soil moisture data. Journal of Geophysical Research, 119(9), 5128-5141, doi:10.1002/2014JD021489.
- Brocca, L., Filippucci, P., Hahn, S., Ciabatta, L., Massari, C., Camici, S., Schüller, L., Bojkov, B., Wagner, W. (2019). SM2RAIN-ASCAT (2007-2018): global daily satellite rainfall from ASCAT soil moisture. Earth System Science Data, 11, 1583–1601, doi:10.5194/essd-11-1583-2019.
- Brunetti, M.T., Melillo, M., Peruccacci, S., Ciabatta, L., Brocca, L. (2018). How far are we from the use of satellite data in landslide forecasting? Remote Sensing of Environment, 210, 65-75, doi:10.1016/j.rse.2018.03.016.
- Ciabatta, L., Massari, C., Brocca, L., Gruber, A., Reimer, C., Hahn, S., Paulik, C., Dorigo, W., Kidd, R., Wagner, W. (2018). SM2RAIN-CCI: a new global long-term rainfall data set derived from ESA CCI soil moisture. Earth System Science Data, 10, 267-280, doi:10.5194/essd-10-267-2018.
- Camici, S., Ciabatta, L., Massari, C., Brocca, L. (2018). How reliable are satellite precipitation estimates for driving hydrological models: a verification study over the Mediterranean area. Journal of Hydrology, 563, 950-961, doi:10.1016/j.jhydrol.2018.06.067.
- Fereidoon, M., Koch, M., Brocca, L. (2019). Predicting rainfall and runoff through satellite soil moisture data and SWAT modelling for a poorly gauged basin in Iran. Water, 11(3), 594, doi:10.3390/w11030594.
- Massari, C., Camici, S., Ciabatta, L., Brocca, L. (2018). Exploiting satellite-based surface soil moisture for flood forecasting in the Mediterranean area: state update versus rainfall correction. Remote Sensing, 10(2), 292, doi: 10.3390/rs10020292.
- Massari, C., Brocca, L., Pellarin, T., Abramowitz, G., Filippucci, P., Ciabatta, L., Maggioni, V., Kerr, Y., Fernández-Prieto, D. (2019). A daily/25km short-latency rainfall product for data scarce regions based on the integration of the GPM IMERG Early Run with multiple satellite soil moisture products. Hydrology and Earth System Sciences Discussion, doi:10.5194/hess-2019-387.
- Mazzoleni, M., Brandimarte, L., Amaranto, A. (2019). Evaluating precipitation datasets for large-scale distributed hydrological modelling. Journal of Hydrology, 578, 124076.
About the authors
Luca Brocca received his Master’s degree in Environmental Engineering in 2003 and his PhD in Civil Engineering in 2008. Since (2009) 2019 he is a (Researcher) Director of Research at the Research Institute for Geo-Hydrological Protection (IRPI) of the National Research Council (CNR) of Perugia (Italy). He has authored and co-authored 130+ journal papers and has been cited 7,000+ times. Luca develops innovative methods for exploiting satellite observations in hydrological applications, such as floods and landslides, drought and agriculture. He actively participates as the Principal Investigator (PI) and co-PI on several research projects within the frame of Italian, European and International programmes. Recent innovations are the SM2RAIN algorithm for estimating precipitation and the irrigation algorithm for which he won the BayWa Smart Farming Challenge of the Copernicus Masters 2018.
Luca Ciabatta received his Master’s degree in Geological Science and Technologies in 2012 at the University of Perugia and his PhD in Civil and Environmental Engineering in 2018 jointly at the University of Florence and at Vienna University of Technology. Since 2013 he is working at the Research Institute for Geo-Hydrological Protection (IRPI) of the National Research Council (CNR). His research interests regard the use of satellite data for hydrological applications and natural hazards mitigation. He is involved in satellite data validation, in the development of satellite-derived rainfall datasets (through SM2RAIN) and in the development of hydrological models. He actively participates in several research projects within the fame of Italian, European and International programmes. He has been author and co-author of 30+ journal referred papers.
Christian Massari received his Master’s degree in Environmental Engineering in 2008 and his PhD in Hydraulic Engineering at the University of Perugia (Italy) in 2011. Since 2017 he is permanent researcher at the Research Institute for Geo-Hydrological Protection (IRPI) of the National Research Council (CNR). His research interests include: hydrological and land surface water balance modelling, development of land data assimilation systems to ingest remote sensing retrievals, microwave and radar remote sensing of soil moisture and precipitation, supply pipe system diagnosis for the reduction of energy and water waste, floods and drought hazards assessment through remote sensing information and food security. He actively participated as Principal Investigator and co-Investigator to several research projects in the frame of Italian and European programs and has been the author and co-author of 50+ journal refereed papers with 1500+ citations. Among others, he is a member of the American Geophysical Union Technical Committee on Precipitation.
Stefania Camici received her Master’s degree in Environmental Engineering in 2008 and her PhD in Environmental Engineering at the University of Perugia (Italy) in 2016. Since 2008 she is working at Research Institute of Geo-Hydrological Protection (IRPI) of the National Research Council (CNR) in Perugia in the field of flooding risk assessment, also in the context of climate change. Since 2012 she has been a research scientist with CNR-IRPI. Her research interest is the flood frequency estimation also in a context of climate change and her research experience encompasses hydrological modelling for flood and flash flood prediction. Recently, her work has been focused on the exploitation of satellite data for hydrological applications and specifically for monitoring extreme events (i.e. flood and drought). She actively participated as Principal Investigator and co-Investigator to several research projects in the frame of Italian and European programs and has been author and co-author of 30+ journal referred.
Paolo Filippucci received his Master’s degree in Mechanical Engineering with excellence, from the University of Perugia (Perugia), Italy, in 2015. In November 2018 he started a doctorate course in Civil and Environmental Engineering jointly at the University of Florence and at Vienna University of Technology (TUWIEN). From August to December 2016, he carried out an Internship with the International Water Management Institute (IWMI). Since 2017 he is working at the Research Institute of Geo-Hydrological Protection (IRPI) of the National Research Council (CNR) in Perugia. His research interests include: Microwave remote sensing for soil moisture and precipitation, with the development of satellite-derived rainfall datasets (through SM2RAIN), Irrigation estimation from microwave remote sensing, River Discharge estimation from remote sensing. He actively participates in several research projects within the fame of Italian, European and International programmes and he has been author and co-author of several journals referred papers. He was one of the winners of BayWa Smart Farming Challenge of the Copernicus Masters 2018.
pushpendra
SM2RAIN has been a highly innovative idea in hydrological modelling. An inverse approach would be extremely useful in data scars regions.
Luca Brocca
Many thanks for the comment, yes data scarce regions is our target!
Luca Brocca
Happy to announce that the paper describing and showing the results for river flow prediction in Africa has been just published on Scientific Reports: https://www.nature.com/articles/s41598-020-69343-x
Vale Cigala
Thank you, Luca, for the update and congratulations on the publication!
zbiri asmae
Many researchers have told me that it is impossible to switch from soil moisture to precipitation!!!
And that there is no evidence that the SWI soil moisture data is better than the precipitation data.
It was suggested to me that we could try to correct the SWI data with precipitation.
But I was sure that this can be done because it depends on science and experiments. The algorithm only does its job…
I can confirm that the results are better especially in Africa.
Thank you Mr. Brocca and well done.
Zbiri Asmae.
Morocco.
Luca Brocca
Thanks! Read the comment just now.
Asmae Zbiri
Dear colleagues,
I hope you are well and taking precautions in this global pandemic.
I’d like to bring your attention to our experiences with remote sensing data that may be useful in this kind of report.
We want to thank all organisations who inspired and helped to do this work.
So, we are trying to use this index in drought monitoring, Yield monitoring…
Now I would like to share with you our latest publication “New investigation and challenge for spatiotemporal drought monitoring using bottom-up precipitation dataset (SM2RAIN-ASCAT) and NDVI in Moroccan arid rangelands” submited to Ekológia (Bratislava) journal indexed at Scopus.
https://lnkd.in/e9RMZ5Uu
Regards.