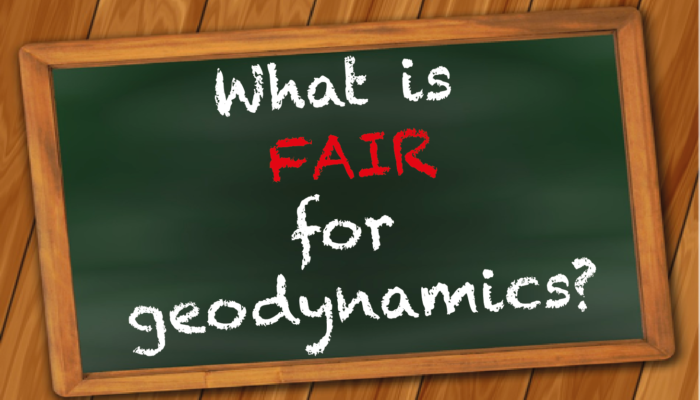
A very important part of science, which is getting more and more attention, is how to share and manage our data. This week, Adina E. Pusok, Postdoctoral Researcher at the Department of Earth Sciences, University of Oxford, shares thoughts and tips on how to make our science fairer. So, what is FAIR for Geodynamics?
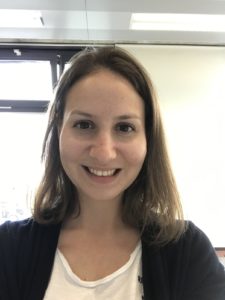
Adina Pusok. Postdoctoral Researcher in the Department of Earth Sciences, University of Oxford, UK.
This is a big question! I would say that what is fair for geodynamics is fair for academia and society in general. For example, equal opportunities for prospective students, diversity across age, gender, ethnicity, but also national borders, good work-life balance, healthy work environments, fair career opportunities and stability, etc. However, this post is about a different FAIRness, but which, as you will see later, still relates to the culture and practices of the scientific community.
I will talk about the FAIR principles regarding data management. The idea for this post came after exploring dedicated sessions and short courses during #shareEGU2020 and because last year many journals (AGU, Springer Nature)* adapted their author guidelines in line with FAIR data protocols.
But why is this important? Well, a large part of geodynamic research work involves software and producing numerical data. Over the last decades, the rate and volume at which research data are created, and the potential to make data available for analysis and reuse has increased exponentially (Wilkinson et al. 2016, Collins et al. 2018). However, credit is traditionally given only in the form of citations to published papers, rather than to the quality of the code or the data. This often leads scientists not to share data or code. And in cases when the software is open source and data is available, there is no (or not enough) information on how to handle them. This is bad and inefficient for science progression, as a researcher can spend about 80% of their time on discovering, creating, and preparing data for research (sometimes even reproducing the same data) (Stall et al. 2019). This can and should be improved (Figure 1).
Scientists do generally agree that more effort should be put into making data available. However, it is not sufficient to simply post data and other research-related materials on the internet and hope that the motivation and skill of the potential user are sufficient to enable reuse. There is a need for an infrastructure that allows data to be discovered, understood and used (Collins et al. 2018).
The main questions I will discuss in this blog post are: Can we make geodynamic data FAIR? What can we gain out of FAIR data? As we will see, these questions do not have simple, straightforward answers, but there are guidelines and examples from the wider scientific community.
What is FAIR?
The FAIR Guiding Principles for scientific data management and stewardship were published in Scientific Data (Wilkinson et al. 2016) with the intention to provide guidelines to improve the Findability, Accessibility, Interoperability, and Reuse of digital assets and the reuse of scholarly data (Figure 2).
There are detailed online resources of what FAIR means (i.e., Wikipedia), so I will just briefly mention the core features:
- Data should be easily found by humans and computers using a persistent identifier (PID) like the Digital Object Identifier (DOI). PIDs prevent link rot, which happens when hyperlinks stop referring to the original source through time because they are moved or deleted. Without a PID, the data object simply will not be findable let alone reusable in the long run.
- Data should have a sufficient set of metadata that provide information on what the data contains. The quality of the descriptive information regarding the data has a profound impact on their reusability. It should contain enough details to make the data findable but also understandable and reusable by other researchers.
- Data should be stored in repositories. Repositories can be general-purpose or discipline-related, institutional or globally open. Those that provide PIDs are even better. Examples: Dataverse, FigShare, Dryad, Mendeley Data, Zenodo, DataHub, or EUDat. More discipline related repositories can be found on re3data.org, including Geophysics.
- Data or software should have a clear license. Researchers (and computers) who find a dataset should immediately know what they are allowed to do with it. Important to understand that not all data has to be made open. Data can be restricted and still be FAIR.
- Data should also be accompanied by related materials, which may include the code used to process and analyse the data, research literature that provides further insights into the creation and interpretation of the data and other related information.
- Data should be well documented. README files help others to interpret and reuse data. A README plain text file should contain the following information: a description of data it includes, describing the relationships to the tables, figures, or sections within the accompanying publication, data processing steps and associated datasets stored elsewhere. One can write README style metadata and combine the two concepts.
- Use common formats and standards (i.e., controlled vocabularies) to ensure interoperability of data.
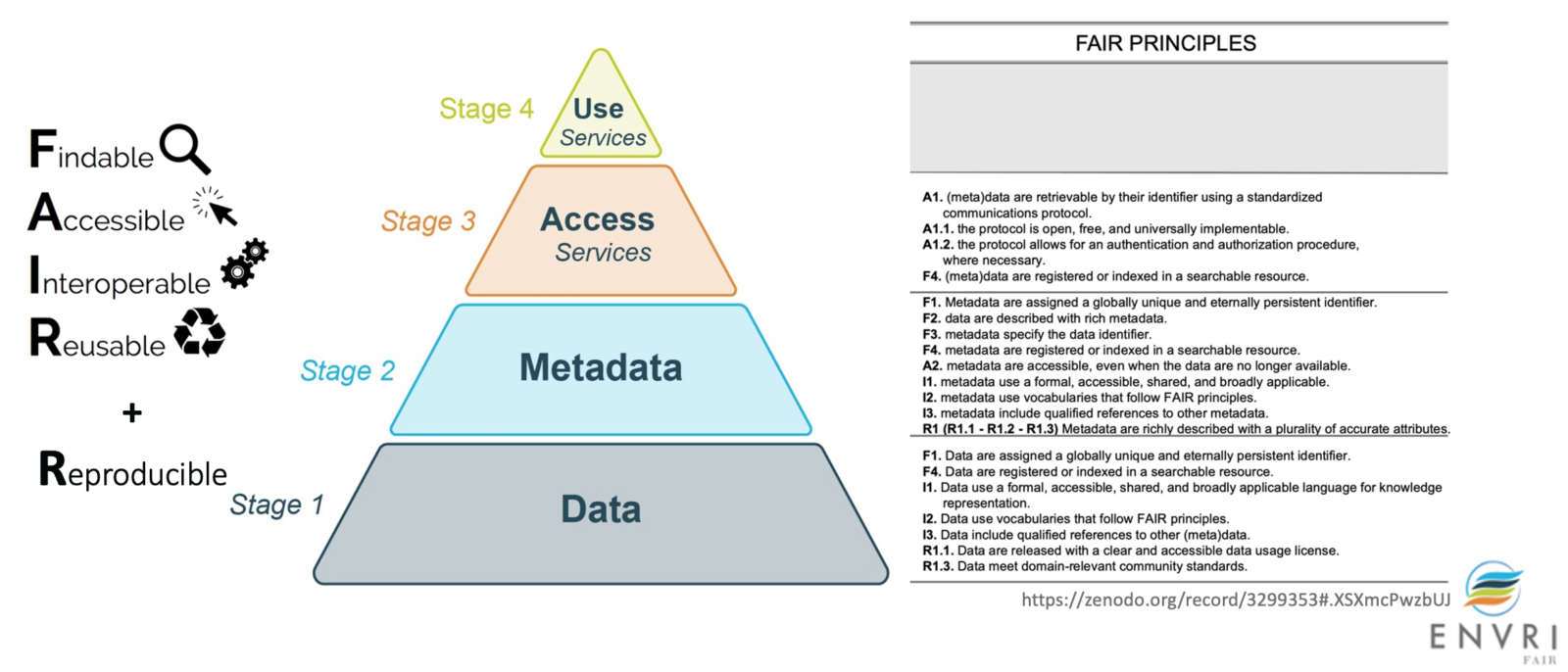
Figure 2. FAIR principles refer to data, metadata, software and infrastructure (adapted from Bailo et al., 2019, Asmi et al., 2020).
Why is FAIR important?
Publication, outreach and impact are increased by making data FAIR and the possibility of researchers and machines discovering data relevant to their research. For example, there is evidence that articles with Open and FAIR data available receive more citations (SPARC Europe report)**. Moreover, FAIR practices also have a high economic return in the long term and open the door to citizen science, which is an increasingly important policy objective (Collins et al. 2018).
How to make data FAIR?
First, how FAIR are your data? Take this test to find out (Jones and Grootvelt, 2017).
To make data FAIR requires good data management and stewardship. But what constitutes ‘good data management’ is, however, largely undefined, and is generally left as a decision for the data or repository owner. Making data FAIR is a joint responsibility of all stakeholders (funders, researchers, publishers, third parties). In response to this, funders and publishers are beginning to require data management and stewardship plans for data generated in publicly funded experiments. They also understand that investment into a FAIR ecosystem is necessary. A FAIR ecosystem would comprise of key data services such as providing PIDs, metadata specifications, stewardship and repositories, actionable policies, training and Data Management Plans (Collins et al. 2018).
But mostly, FAIR requires key changes in the practice and culture of scientific communities. This can be done only by recognising that data are a key research output type. To formally encourage this, two main things can be done:
- Professionalize the roles of people specializing in data science and data stewardship. New job profiles need to be created at institution/community level in order to support the transition to FAIR. Education programmes are also needed to train data scientists and data stewards and support researchers.
- Effective recognition and rewards to include efforts of FAIR data. The current reward system based on academic publications is considered a major obstacle to FAIR data (European Open Science Cloud report), so a range of new metrics and incentives for FAIR data are needed. Career rewards need to evolve to reflect the value of data sharing, curation, stewardship and reuse. Scientists too, need to break with existing metrics, which are embedded in longstanding academic culture. It is important that metrics should not encourage quantity over quality.
One other challenge to making data FAIR is that the requirements and resources are not static, they evolve over time based on the data lifecycle, changes within the designated user needs and transition to new technology such as cloud. However, the best FAIR initiatives are generally research-driven and backed by communities or large infrastructures, making sure the data are relevant and used in time.
Adoption by scientific communities
The strong policy push towards FAIR (and Open) data comes primarily from governmental agencies. For example, since 2016, G20 leaders issued a statement endorsing the application of FAIR principles to research and have taken steps to establish infrastructures (i.e., Go Fair). FAIR principles are important for creating high-quality reports regarding societally important issues (i.e., IPCC report, Juckes et al., 2020).
Therefore, some scientific disciplines have already made great progress in the sharing and reuse of FAIR data, and important lessons on diversity and obstacles can be learnt from these examples. Other communities established a way to share data that satisfies their needs without explicitly invoking the FAIR principles (i.e., particle physics shares its data inside the large consortia attached to its experiments, while crystallography developed their own data management system and vocabulary out of need). Some others are beginning to implement part of or all the principles, and others are simply not aware or interested.
Within Earth, space and environmental sciences, a large number of stakeholders signed onto the Enabling FAIR Data Commitment Statement for handling data based on these principles. The responsibilities outlined in the Enabling FAIR Data Commitment Statement include:
- Publishers will strive to ensure that data and other research outputs supporting publications are openly accessible at the time of publication in a FAIR-aligned repository. This means that journals such as AGU or Springer Nature will no longer accept article supplements as the primary archive for data [Stall et al., 2018].
- Repositories will strive to support researchers with open and FAIR data services for data citation, persistent identifiers, and human- and machine-readable descriptions.
- Societies, communities, and institutions will strive to support open and FAIR Data Principles in their activities and policies and promote FAIR initiatives as important criteria in promotion and tenure, awards, or honors.
- Funding agencies and organizations will strive to promote open and FAIR Data Principles in their activities and policies, and implementation of data management plans with FAIR principles.
- Researchers will strive to make all research products FAIR and open by depositing them in FAIR-aligned repositories, cite all research products created or reused in publications, and include a data availability statement in publications to specify where the research products that support the paper can be accessed.
Some notable examples of FAIR initiatives in solid Earth geosciences include:
- EarthChem Library for geochemical, petrological and geochronological data. A recent EGU presentation showed that such an initiative has grown extensively, but this rapid growth also created new challenges dealing with heterogenous data or expectations for immediate data publication (Lehnert et al., 2020).
- EPOS (European Plate Observing System) within the ESFRI Landmark and European Research Infrastructure Consortium. EPOS represents a community of solid Earth sciences laboratories including high temperature and pressure experimental facilities, electron microscopy, micro-beam analysis, analogue tectonic and geodynamic modelling, paleomagnetism, and analytical laboratories.
- SAGE (National Science Foundation’s Seismological Facility for the Advancement of Geoscience) operated by IRIS Data services. SAGE is US national facility for the development, deployment, and operational support of seismic instrumentation and data.
Geodynamics
It is clear that each field of research needs to define what it means to be FAIR and decide appropriate measures to assess this. Within Geodynamics, there have been ongoing efforts to improve community practices. Examples include individual (i.e, Crameri, 2019), community-wide (i.e, CIG), or outreach (i.e., EGU Geodynamics blog) efforts. But in terms of FAIRness, the geodynamics community is behind other disciplines. For example, few studies publish numerical data that fit the FAIR principles because there is no good recipe on where or how to store numerical data, which data to make available, or that there is no database of numerical models performed. The common practice is to provide ‘enough’ information to reproduce the numerical data in publication. But that logic has major flaws, as important details can be left out, which may lead to different interpretation of the data, difficulties in reproducing results and to a fragmented science progression.
There are, however, some small (yet big) steps that could be easily adopted by geodynamicists to improve FAIRness:
- Use of repositories that have PIDs for software and reproducible data files (see this article). Git repositories such as Bitbucket, Github, Gitlab are also a good alternative (especially for version control) and there is a push for these repositories to adopt stronger PIDs.
- Consider publishing numerical data results for outreach and teaching. Students can learn while analysing published data.
- Practice citing reused software or data (generally happening), but also including reproduced models or published numerical data.
- Consider publishing in alternative journals such as JOSS, which allow you to link git repositories/software with your publication and gain academic merit (citations) for your software.
- Practice use of metadata or README files. Metadata for a numerical study should include: information on code and dependencies, how to install and run it, parameter list and info (input parameter files), machine information (simulations can be run on desktop, or require high-performance computing resources), information on output and post-processing, and link to other relevant data, repositories. Here’s an example of data git repository: https://bitbucket.org/adina/rep-mconvindia/src/master/.
- Suggest that you and your colleagues improve FAIR practices. More importantly, reviewers should be critical to submissions that do not contain the underlying materials without good reason.
- Use of reproducible manuscripts, for example with Jupyter notebooks (Konkol et al., 2020). It can be difficult and time-consuming but could solve the problem of publishing executable computational research results.
There are other aspects that geodynamicists will have to decide what is appropriate to do. For example, how to implement/enforce data availability and reproducibility and where to draw the practical limits to it, what are best PIDs and license practices, and how to ensure that the efforts are rewarded and best practices followed. But there is no doubt that FAIR data could bring considerable advantages in Geodynamics, such as reproducibility, reuse of data, training of students, teaching, outreach, and, potentially, citizen science. So let’s strive to make Geodynamics data FAIR!***
Footnotes: * EGU journals have not yet adopted FAIR guidelines, but are part of COPE and DOAJ (ethics and open access). ** The author considers that reports commissioned by the EU or other public institutions should also have PIDs for easy reference. *** In addition, the CARE principles extend principles outlined in FAIR data to include Collective benefit, Authority to control, Responsibility, and Ethics to ensure data guidelines address historical contexts and power differentials.
References: Asmi, A., Franz, D., and Petzold, A.: Building the Foundations for Open Applied Earth System Science in ENVRI-FAIR, EGU General Assembly 2020, Online, 4–8 May 2020, EGU2020-13291, https://doi.org/10.5194/egusphere-egu2020-13291 Bailo, D. et al. (2019, July 10). Four-stages FAIR Roadmap - FAIR "Pyramid". Zenodo. http://doi.org/10.5281/zenodo.3299353 Collins, S., Genova, F., Harrower, N. et al. Turning FAIR into reality – Final Report and Action Plan from the European Commision Expert Group on FAIR Data, European Commision (2018), https://ec.europa.eu/info/sites/info/files/turning_fair_into_reality_0.pdf Crameri, Fabio. (2019, May 17). Scientific Colour Maps (Version 5.0.0). Zenodo. http://doi.org/10.5281/zenodo.3596401 Jones, Sarah, and Grootveld, Marjan. (2017, November). How FAIR are your data?. Zenodo. http://doi.org/10.5281/zenodo.1065991 Juckes, M., Pirani, A., Pascoe, C., Matthews, R., Stockhause, M., Chen, B., and Xiaoshi, X.: Implementing FAIR Principles in the IPCC Assessment Process, EGU General Assembly 2020, Online, 4–8 May 2020, EGU2020-10778, https://doi.org/10.5194/egusphere-egu2020-10778 Konkol, M., Nüst, D., and Goulier, L.: Publishing computational research – A review of infrastructures for reproducible and transparent scholarly communication, EGU General Assembly 2020, Online, 4–8 May 2020, EGU2020-17013, https://doi.org/10.5194/egusphere-egu2020-17013 Lehnert, K., Profeta, L., Johansson, A., and Song, L.: Best Practices: The Value and Dilemma of Domain Repositories, EGU General Assembly 2020, Online, 4–8 May 2020, EGU2020-22533, https://doi.org/10.5194/egusphere-egu2020-22533 Stall, S., Yarmey, L., Cutcher-Gershenfeld, J., Hanson, B., Lehnert, K., Nosek, B., Parsons, M., Robinson, M., Wyborn, L. Make scientific data FAIR. Nature 570, 27-29 (2019), doi: 10.1038/d41586-019-01720-7, https://www.nature.com/articles/d41586-019-01720-7 Wilkinson, M., Dumontier, M., Aalbersberg, I. et al. The FAIR Guiding Principles for scientific data management and stewardship. Sci Data 3, 160018 (2016). https://doi.org/10.1038/sdata.2016.18 SPARC-Europe report, ‘The Open Data Citation Advantage’, http://sparceurope.org/open-data-citation-advantage European Open Science Cloud report, ‘Realising the European Open Science Cloud’, https://ec.europa.eu/research/openscience/index.cfm?pg=open-science-cloud-hleg