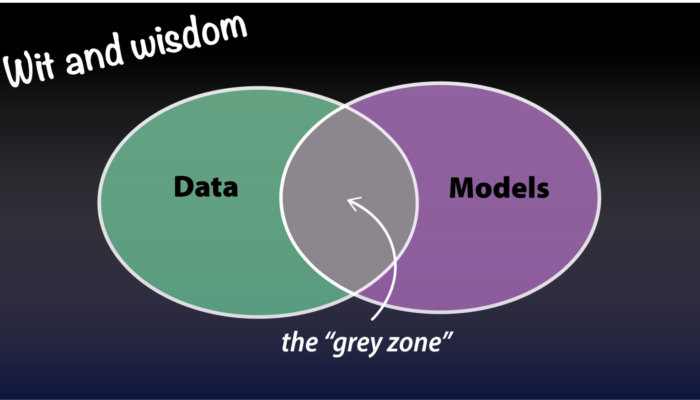
A privilege of being an academic is the freedom to muse, staying faithful to the title of a PhD which is, after all, a doctor of philosophy. In his latest reflection on a topic of importance to all scientific disciplines, Dan Bower (CSH and Ambizione Fellow at the University of Bern) discusses the ambiguity that comes with the separation of data and models.
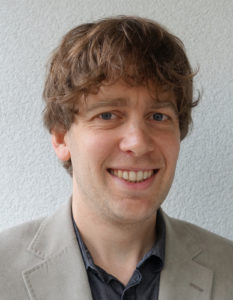
Dan Bower is a CSH and Ambizione Fellow at the University of Bern, Switzerland
What are data? What are models? You are probably wondering how I can write a blog post about this—but bear with me—because these under-appreciated questions affect all scientific disciplines. The tug-of-war between models and data has been viscously fought since the enlightenment to the mutual benefit of both. Modelling has undergone a recent explosion due to the growth of computing power that enables numerical techniques to model physical systems that were beforehand intractable. But the notion of data and models extend beyond their immediate scientific applications; the preference of a scientist to lean towards data or models is often a defining aspect of their character. We’ve all heard (at least anecdotally) of the modeller that confidentially exclaims “the data is wrong, the model is right”. The passion with which this statement is made in a packed room at an international conference demonstrates that—for the individual at least—the vested interest in modelling can be perhaps a little too strong. Clearly, the battle between data and models as mechanisms to advance scientific knowledge is punctuated by the seemingly innate preference of a particular scientist to “believe” in the usefulness of models or data.
Battle for the heart of science
I define an “observer” as a scientist with a preference for data— either directly observed or experimentally collected—and a “modeller” as a scientist that formulates models. In the modern scientific landscape, it’s increasingly difficult to be a true end-member of either definition. Science is the endeavour to uncover the truth of the natural world. Establishing truth was purely observational in the early days; we observed the stars, planets, mountains, animals, plants, the list goes on. Observations by Charles Darwin led to the theory of natural selection – jump-starting modern biology. Observations of planets and stars established the Copernican model of the solar system, and geological observations led to the theory of plate tectonics. In this regard, the observer may always claim the closest affiliation to the heart of what science is, in the sense that a (good) observation is (in the best case scenario) an irrefutable truth about the natural world. What information this truth provides in the context of the bigger picture is not necessarily apparent—this is where modellers pick up the story. A truth of nature cannot be established by simply the outcome of a model or theory without observational proof; recall that Peter Higgs waited almost 50 years to be awarded the Nobel Prize following experimental verification of the Higg’s boson.
A classical observer makes an observation and reports the observation to the scientific community. Following this approach led to the establishment of many scientific fields, including biology, astronomy, and geology. The discovery and classification of a plant species in a rainforest by the pioneering botanists is a clear example of a scientific advancement made exclusively by observation. Essentially, there are no extra steps necessary to take the visual observation of a plant and realise that it is a truth of nature—I’m necessarily avoiding philosophical discussion of how we perceive and understand the natural world from the human perspective. Whilst observations akin to those of the botanist remain present in science, increasingly observers are necessarily dragged into the underworld of modelling—whether willingly or otherwise. This is because our scientific observations have gone beyond what our primitive senses can detect – technological developments in sensors and microchips have opened up a new space of sensory perception that we could never previously explore.
Increasingly observers are necessarily dragged into the underworld of modelling –
whether willingly or otherwise
The guts of the Earth
Seismic tomography provides imagery of Earth’s interior, and for someone interested in the interior dynamics of Earth, seismic tomography is often regarded as data. Models of Earth’s dynamic interior are compared with seismic tomography to determine their validity. This is a perceived common interplay between models and data — independent data can be used to test a model. However, this interplay hides over-simplifications that are important to appreciate. The interior dynamics model is partly built on data. For example, flow parameters such as viscosity are based on a secondary model devised from fits to experimental data. Note that modellers refer to viscosity as a “parameter” rather than “data”. There is no problem with this approach, as long as we don’t then use the primary model to infer the viscosity, as this would be circular reasoning. This demonstrates an important point that is often buried when feedbacks become more complicated in non-linear systems; it can become non-trivial to reason what is truly independent data with which to test our model. Are we analysing what we put in, or are we probing a new or interesting phenomenon?
Furthermore, seismic tomography images are not strictly data, because seismic images have been created by a model using input data; the input data is the observation of surface motions. Furthermore, quantifying the accuracy and precision of these data are critical for determining their worth, yet error estimates are linked to an assumed noise model. Crucially, the input data itself is not “useful” information to non-seismologists, but visualising the 3-D wave speed of Earth’s interior is tremendously informative to fanatical geoscientists. Nowadays, tomographic models have converged to produce a coherent picture of the dominant features of Earth’s interior, such that we can argue that agreement between tomographic models has effectively established a truth of nature. This argument is bolstered by the fact that the interior is inaccessible, so there is not another approach to determine the interior wave speed structure. Nevertheless, we should not lose sight of the fact that any model outcome and any data is never immune from further scrutiny.
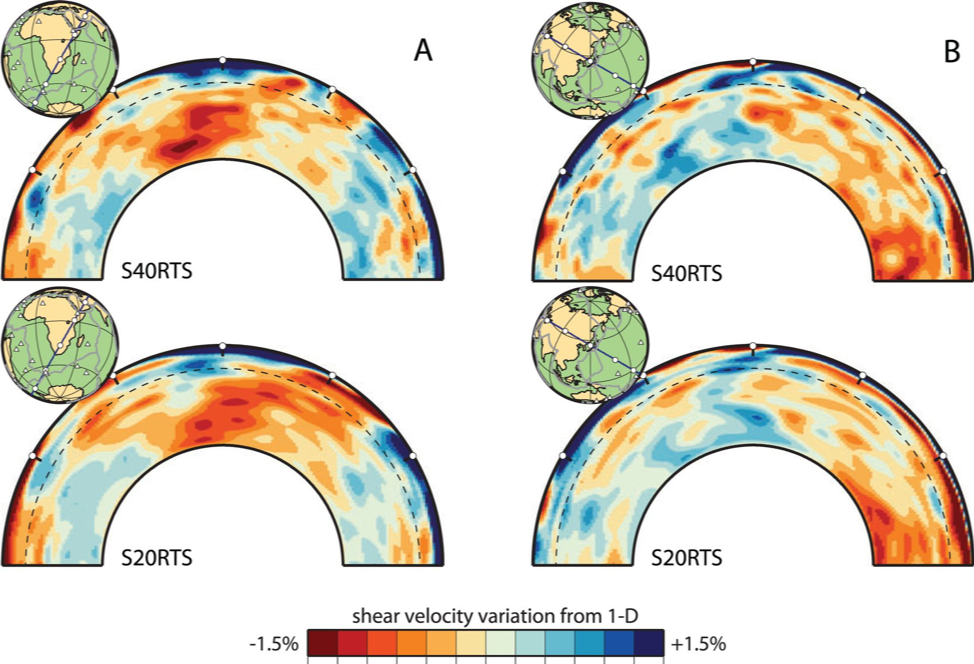
An example of the “grey zone” between models and data in Earth sciences is seismic tomography. Here shown are whole-mantle cross-sections for models S40RTS and S20RTS, from Ritsema et al. (2011).
Cultural sensitivity
Despite the fact that models and data are nowadays intimately intertwined in all fields of modern science, there is still a perceived cultural difference between a scientist who is an observer versus one who is a modeller. The cost and technology necessary to make observations—whether a mass spectrometer in a laboratory, space telescope, or particle accelerator—requires a high level of expertise, time, and collaboration. An observer is understandably motivated to b-line to a truth of a nature through careful observation of a particular system, but this may be at the expense of a more general understanding (i.e., one observation is one data point). There can also be the perception that all data is “useful” — after all, it’s a new truth of nature — even though it may not provide any further information that influences our understanding. By contrast, a modeller is motivated to connect the dots to provide meaning to what may initially appear as disparate data. A modeller is typically driven to generate a holistic view of a complex system; ground-truthing the model against independent data is, of course, critical to the eventual success of a model, but a modeller is usually less beholden to any one particular data point. This is an acknowledgement of the modeller that the model cannot ever capture all the complexity of nature and thus reproduce all data; the goal is to capture the key components to provide understanding and predictive power.
But not all hope is lost for ardent modellers to stamp their signature on the annals of scientific discovery. This is because the best models — those with unprecedented ability to explain observations and make predictions (which are later verified by more observations) — become scientific theories. And the most prominent scientific theories then establish new branches of science; natural selection is the tenet of modern biology and plate tectonics is the unifying framework of geology. Newton’s laws now permeate all branches of science, which at its core contains a beautifully elegant description of motion through a quantitative model, encapsulated in one of the most famous equations: F = ma. Now, of course, even the mighty Newton’s laws are only valid within a certain parameter space, but that parameter space is both extensive and relevant for scientific and engineering applications, such that the laws have stood the test of time. So modellers should remain optimistic that, at least in principle, they too can influence the course of science by effectively establishing new truths of nature (albeit with a little help from their observer colleagues). This is typically more likely for disciplines that are amiable to purely theory-based models, such as physics or astrophysics; by contrast, the lack of so-called “clean” physics in other disciplines renders an all-predictive quantitative model a formidable task (e.g., evolution in biology, plate tectonics in Earth science).
Life in the grey zone
So where does this leave us? Well, I think we must accept that we are in an era of data-dependent models and model-dependent data, and therefore the lines have been blurred between the classical notions of what are models and what are data. Remaining steadfast to what we perceive as either a “pure” model or “pure” data ignores the fact that one is increasingly inherent to the other. This point is emphasised by the growth of machine learning in all scientific disciplines, whereby a mathematical model is used to extract data from data, as it were. As we read and review papers and advance our own research projects, it is always a useful exercise to critically assess what is a model, and what are data. We must determine what is the truth of nature we are trying to probe, and how what we deem to be models or data affect our understanding of this truth. And whilst it is inevitable that there will be an increase of scientists equally apt to data collection as well as modelling, we should remember that history has ingrained the idea of being a modeller or an observer deep into an individual scientists psyche.
Ritsema, J., Deuss, A., van Heijst, H.J. and Woodhouse, J.H. (2011) S40RTS: a degree-40 shear-velocity model for the mantle from new Rayleigh wave dispersion, teleseismic traveltime and normal-mode splitting function measurements. Geophysical Journal International, vol. 184(3), pp. 1223-1236,https://doi.org/10.1111/j.1365-246X.2010.04884.x
geo89
It is a really good piece. But I think that there are some few stuff that must be addressed. For example, I think that it is not solely the data collection and observation that drives the evolution of scientific knowledge, but from a holistic perspective all the scientific community and social environment. For example, sometimes the same data set is interpreted in different ways, and old fashioned theory worked (although they were extremely complicated).
The observation scientist is not totally without prejudice, moreover, the way in which the data are handled may hide some unexpected observational bias. On the other hand, the modeller may hunt the perfect set of parameters that fits the observational data without asking if the collected data and the relative interpretation may be completely free of any unintentional bias.
Patrick Sanan
There’s a related narrative these days, that of the “third pillar” of computational science. This says that while the data vs. models / induction vs. deduction /experiment vs. theory / etc. dynamic has been playing out in science since its inception, only nowadays are we in the position to be working with a new type of beast: (computer) models which are so complicated that they themselves need to be examined with the tools of observational science. Blurry lines indeed!