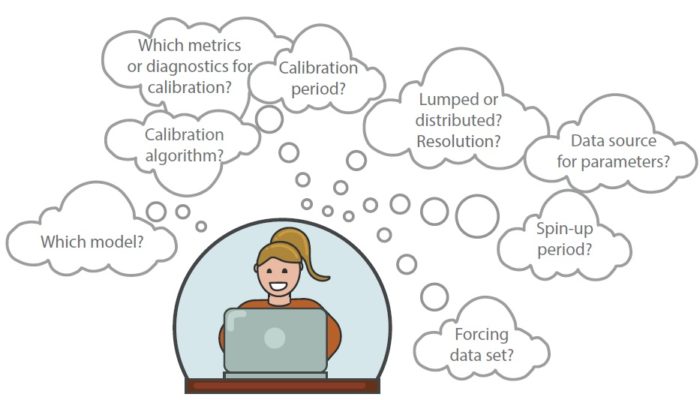
Several studies were conducted and are ongoing where we investigate modelers, modeling decisions and modeling perceptions. Below I discuss the rationale and a summary of the (preliminary) results.
Simulation models, conceptualizations of processes into a system of mathematical equations (hereafter simply referred to as models), are frequently used tools in the hydrological sciences. The literature review of Burt & McDonnell (2015)1 demonstrates the decline in fieldwork papers, and a strong increase in modelling papers in the field of runoff. So even in the geosciences, a science that seems to have a clear invitation to go outside and explore, computer models are increasingly popular for scientific research.
Don’t most hydrologists consider themselves a modeler in one way or another?
We develop and use models to enhance process understanding, to support decision making, to make forecasts and predictions, to investigate relations. Even when we don’t always consider ourselves a modeler directly, there is a big chance that somewhere in our career we encounter(ed) models.
- But how do modelers model?
- And is there a difference in how modelers model?
- And does this have consequences for the results?
It’s these questions that keep me busy lately, and that I try to understand and comprehend.
This is sometimes challenging, because many people consider physics and mathematics as something ‘objective’, and as a consequence, models that are based on physics and mathematics should be objective tools too.
But we are still faced with many uncertainties in our field, due to the lack of observations, their limited spatial and temporal coverage, the problem of not being able to conduct controlled experiments, scaling issues in general, computer constraints, et cetera. This forces us to make simplifications and conceptualizations when we translate the real world into a model. And the trade-offs we consider when making these decisions, might differ from person to person. As such, models should be considered social constructs2, which provides a strong rationale to investigate the modelers themselves.
Model development and model selection
Let’s have a look at the very first step of a modeling exercise: selecting or defining the mathematical equations in a model.
Clark et al. (2011)3 already discusses the risk of developing parental affection towards your own hypothesis (as inspired by Chamberlin, 1890). Babel et al. (2019)4 conducted interviews with several model developers in various fields of the geosciences. With these interviews, she revealed the large role of habit in model development, related to the education and background of the modeler. The role of the modeler is discussed from a gender-perspective in Packett et al. (2020)5, highlighting that gender considerations could lead to different modelling choices.
Rather than developing a model, one can also select an off-the-shelve model. In a bibliometric study6, we analyzed publications from seven frequently used hydrological models, and searched for predictors for model selection. It turns out that the institute of the first author was most strongly related to model selection, more than spatial or temporal application scale of the model, goal of the study, or landscape descriptors. We hypothesize that this relates to the role of legacy; within an institute, experience with a model is present which is helpful in identifying spurious model results or solving errors or bugs, and there might be infrastructure available that makes setting up and working with the model more efficient. We consider these valid reasons for model choice, but the results do demonstrate that model choice is not something “objective”.
Model complexity
So, model development and model selection relate to the environment and the background of the modeler, but there are many more facets of modeling that are exposed to personal judgement and background.
A questionnaire on the perception of model complexity in geosciences was distributed among geoscientists both in and outside academia7. We received over 600 responses. The questionnaire revealed that definitions and perceptions of model complexity were very heterogeneous. Confidence was not per se higher in the simulations of a complex model compared to a simple one, and neither gender, the discipline within the geosciences, nor career stage or work sector, explained the characterization of model complexity.
The results show that there are no generally accepted definitions for modeling terms such as complexity, even though many people might assume their own definition as being generally accepted. This not only hampers collaboration, but also, again, demonstrates the role of personal judgement in modeling.
Interested to read what your fellow modelers answered to our questions? You can find the paper here.
Model configuration
After selecting a model or developing a model, the modeler still has many more decisions to make related to the configuration of the model. The decisions in the configuration demonstrate a clear path dependency, as described by Lahtinen et al. (2017)8. Decisions related to model configuration depend on decisions related to the model. If a distributed model is used, it requires, for instance, a decision on how to spatially interpolate the forcing data.
I am currently conducting interviews with hydrological modelers from different institutes and at different career stages, to investigate how and why they made certain decisions. From the interviews I learned, for instance, that in one research group, many people used the same spatial interpolation method for downscaling of forcing data, because there is a script available that does the job. When I asked whether other interpolation methods were considered, some people answered that they did not have time to consider any other method, or that they trusted their colleague’s recommendation on using this method. At the same time, everyone acknowledged that this choice influences the final model results.
Preliminary results also suggest that scoping by funding agencies is a really important step in the modelling chain; modelers are for instance imposed to use the datasets that were developed by the funding agencies, rather than to make their own consideration on which dataset to use.
This shows that modeling is definitely not only about objective physics or mathematics – many social processes play an important role in the modeling process.
On modelers and modeling
With these studies in mind, we can come back to the questions that I aim to address:
- How do modelers model? This cannot unambiguously be answered, but at least we can conclude that it is a social process that requires closer scrutiny.
- Is there a difference in how modelers model? Yes, because this seems to relate to the environment and the background of the modeler, and that differs from modeler to modeler.
- Does this have consequences for the results?
The impact of some modeling decisions have been quantified and demonstrate significant impact on the model results9. Some modeling decisions will have more impact on the results than others – the next step is to quantify the impact of all decisions that come forward from the interviews.
An important consideration here, though, is that results can be considered twofold; if the model is used to support decision making, the model results themselves might change, but this might not impact the final decision. To what extent the impact of subjective modeling decisions propagate into the policy decision making process is an area that requires further exploration.
There is no right or wrong – we need modeler ensembles
Science is often considered an activity that strives for ‘objective knowledge’. Acknowledging models as social constructs seems to contradict this strive. This is a delicate discussion; perhaps objective knowledge is an ideal that can never be achieved. I believe we can get close to this ideal on the one hand by carefully structuring our methods (for model studies through good modelling practice, step 1: keeping a logbook), and on the other hand by having many people working on the same topic.
This works in the same way as a model ensemble. In a model ensemble each model has its own drawbacks, strengths, bugs, and assumptions, but these are believed to cancel each other out in model ensembles, explaining why the ensemble mean generally performs best.
Modelers also have their own strengths, weaknesses, implicit assumptions and world views. These two factors (modeler and model) are now always combined in model intercomparison studies. More attention to the ensemble of the modelers – e.g. by paying more attention to diversity in the modeler team on several aspects, such as experience or background – might even further improve the validity of the ensemble mean. We need more carefully designed modeler ensembles!
Anything to share related to this topic?
These are my casual and short thoughts about modelers and modeling. I am happy to hear your opinion or insights, and to discuss (ongoing) work. Please feel free to contact me.
References
- Burt, T. P., & McDonnell, J. J. (2015). Whither field hydrology? The need for discovery science and outrageous hydrological hypotheses. Water Resources Research, 51, 5919–5928. https://doi.org/10.1002/2014WR016839
- Melsen, , J. Vos, R. Boelens (2018), What is the role of the model in socio-hydrology? Discussion of ‘Prediction in a socio- hydrological world’, Hydr. Sci. J. 63. doi: 10.1080/02626667.2018.1499025
- Clark, M.P., D. Kavetski, F. Fenicia (2011) Pursuing the method of multiple working hypotheses for hydrological modeling, Water Resour. Res. 47, W09301, doi: 10.1029/2010WR009827
- Babel, L., D. Vinck, D. Karssenberg (2019), Decision-making in model construction: Unveiling habits, Env. Mod. Softw. 120, 104490, doi: 1016/j.envsoft.2019.07.015
- Packett, E., N.J. Grigg, J. Wu, S.M. Cuddy, P.J. Wallbrink, A.J. Jakeman (2020), Mainstreaming gender into water management modelling processes, Env. Mod. Softw. 127, 104683, doi: 1016/j.envsoft.2020.104683
- Addor, N., and Melsen, L.A. (2019), Legacy, rather than adequacy, drives the selection of hydrological models. Water Resour. Res. 55, doi: 10.1029/2018WR022958
- Baartman J., L. Melsen, D. Moore, M. Van der Ploeg (2020) On the complexity of model complexity: Viewpoints across the geosciences, Catena 186: 104261. doi:10.1016/j.catena.2019.104261
- J.Lahtinen, J.H.A. Guillaume and R.P. Hämäläinen (2017), Why pay attention to paths in the practice of environmental modelling? Env. Mod. Softw. 92, 74-81, doi:10.1016/j.envsoft.2017.02.019
- Melsen, L., Teuling, A., Torfs, P., Zappa, M., Mizukami, N., Mendoza, P., Clark, M., Uijlenhoet, R. (2019), Subjective modeling decisions can significantly impact the simulation of flood and drought events, J. Hydrol. 568, p. 1093-1104. doi; 10.1016/j.jhydrol.2018.11.046
Guest author Lieke Melsen is a researcher and lecturer at the Hydrology and Quantitative Water Management group on the topic of Computational Hydrology at Wageningen University and Research in The Netherlands. Her research focuses on hydrological modelling practices to identify model uncertainty, hydrological model selection for modelling studies, configuration of models and influence on the model output, model intercomparison studies, (Bayesian) statistics and sensitivity analysis.
Edited by Maria-Helena Ramos