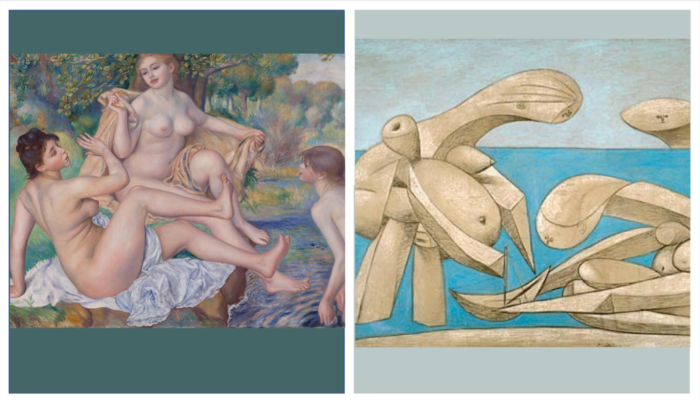
“All models are wrong but some are useful” is a quote you probably have heard if you work in the field of computational hydrology – or ‘hydroinformatics’ – the science (or craft?) of building computer models of hydrological systems. The idea is that, even if these models cannot (by definition!) be a 1:1 representation of reality, their erroneous predictions can still be useful to support decision-making – for flood protection agencies, urban planners, farmers, water resource managers, etc.
From a modeller perspective, however, the question remains open: how do we decide whether a particular model (the one we are developing!) is ‘wrong within reasonable limits’ and thus suitable for use? How do we decide whether a certain simplifying assumption is acceptable? Or whether adding details is really beneficial? Ironically, one of the most thoughtful – and enjoyable – articles about the matter was published the year I started primary school… and here I am writing about it 30+ years later… (… so maybe it was ‘destiny’ after all?!).
MO’ DATA, MO’ PROBLEMS
One way to test (or ‘validate’) a computer model is by comparing its predictions against observations. If the model is able to reproduce the data collected in past circumstances, then it can be used to make predictions in other circumstances: those we are observing now (forecasting) or some that may occur in the more distant future and are of interest to us (simulation). Underpinning this approach is an implicit assumption: we trust the data – the ones we use for ‘validation’ and those we will feed into the validated model when making new predictions. However, this is a strong assumption in hydrology where, differently from some other sciences, data are not generated through controlled lab experiments but collected ‘out there’ – on hillslopes, in rivers, etc. – where the ‘observed’ processes are affected by myriads of factors, mostly out of our control, often non stationary, sometimes simply unknown (a crucial point I first came across in one of the very first papers I read for my master thesis – and clearly stuck to mind!).
One may think the problem will go away as we get new data products from intelligent sensors, satellites, drones, etc. And yet even these data will not cover all components of the water cycle (think of groundwater); they often require complex pre-processing, which introduces a whole new set of uncertainties; and they cannot completely close the spatial, temporal or conceptual mismatches between the quantities represented in our models and those we actually measure. So, it seems to me that the availability of new data products, rather than solving our problems with computer model development, is mainly giving us a deeper understanding of their deficiencies and limitations.
CODE HARD BUT TEST HARDER
If we cannot simply rely on data for validation, where else should model credibility come from? As pointed out in another great paper on model validation in earth sciences, often the most we can ask of a computer model is, simply, that it “does not contain known or detectable flaws and is internally consistent” – in other words, that it behaves as we expect it to.
Such a validation criterion may sound weak: so models are not meant to reproduce reality but only our understanding of it? Well, I’d say… yes: we use models to reveal how complex systems may behave, given the behaviour we have defined for their individual components. But computer models do not possess more knowledge than we do; they only do the calculations for us – more accurately and more quickly than we would be able to do otherwise (unless we have human computers!).
The criterion may sound obvious: wouldn’t all computer models pass it? Here I’d like to say ‘yes’ but… I am afraid not! In my experience, hydrological models can quickly go ‘out of hand’ if only we combine a few (non-linear) processes and parameters. And when we scrutinise them a bit deeper, we often find that, be it because of plain bugs in the code or more subtle numerical interactions, they may behave in ways that we would have not anticipated, and are inconsistent with what we know about the real system.
So, I think we have to accept that adding complexity to a model does not guarantee, per se, that it will give us a better representation of the real system. Actually, there may even be a trade-off whereby adding complexity reduces our ability to carry out a comprehensive testing of the model behaviour, paradoxically undermining its credibility instead of enhancing it. Ultimately, what we need is not only more sophisticate models but also more sophisticate evaluation tests – using formal, structured approach to analyse propagation of uncertainties and sensitivities. And to accept that sometimes a simpler model with known behaviour and limitations is probably more useful than a more complex one that cannot be comprehensively tested.
Edited by Maria-Helena Ramos
________________________________________
Guest author Dr Francesca Pianosi is a lecturer in Water and Environmental Engineering at the Department of Civil Engineering of the University of Bristol, and an EPSRC Fellow. Her main research interest is the application of mathematical modelling to advance the understanding and support the sustainable management of human-environment systems, and in particular water resource systems. She is also interested in open-source software development and is the lead author of the SAFE Toolbox (safetoolbox.info).
Warner Marzocchi
Thank you for this post. This topic is of great interest, and too often completely overlooked by many researchers.
In my experience I struggled to give an answer to questions like
“if the cornerstone of science is to test (validate) one model against the data, what about the Earth system in which all models are wrong? Is it still Science if I know since the beginning that all models are wrong?”
Together with Tom Jordan from USC we published a paper on this topic describing our view of the problem, and our answers to these questions.
Marzocchi, Jordan (2014). Testing for ontological errors in probabilistic forecasting models of natural systems. Proc. Natl. Acad. Sci., 111(33), 11973-11978.
https://www.dropbox.com/s/ei0qnnv3gsxb5d5/PNAS_Marzocchi_Jordan_2014.pdf?dl=0
Hopefully this might stimulate more thoughts on this important topic
Francesca Pianosi
Thank you for your comment and for sharing your PNAS paper, it is indeed very relevant!
David A Sampson
Thanks for your post. I did enjoy it. I have to admit, I extremely dislike the quote “All models are wrong, but some are useful.” I have been “modelling since 1987, so I have been around the block a few times. I have created several published models; I write raw code to produce the models that I use. Sometimes I borrow good code when appropriate (e.g., Farquhar photosynthesis, Thornthwaite evaporation, etc.). A model is nothing more than a collection of algorithms framed as hypotheses regarding how a system works. If the model is wrong, then the hypotheses are wrong (I guess the driving data could also be “wrong”). So, it cannot be that all models are wrong. Rather, I would suggest that those that are not modelers learn to read code. It reminds me of the saying “Those that teach do not know; those that know do not teach.” I suspect that I am being naive. It also takes a systems (thinking) “mind” to understand system behavior.
Francesca Pianosi
I like the quote maybe because I tend to focus on the “useful” attribute more than the “wrong” one 🙂
I suppose the same hypothesis (model) can be “wrong” or “right” depending on the temporal/spatial scale it is applied to, or the purpose that the application is meant to serve – a reminder that we should know our models/codes well in order to apply them usefully, as you also say. Hope I made you a bit more favourable to Box’s quote… Thanks for your comment anyway!