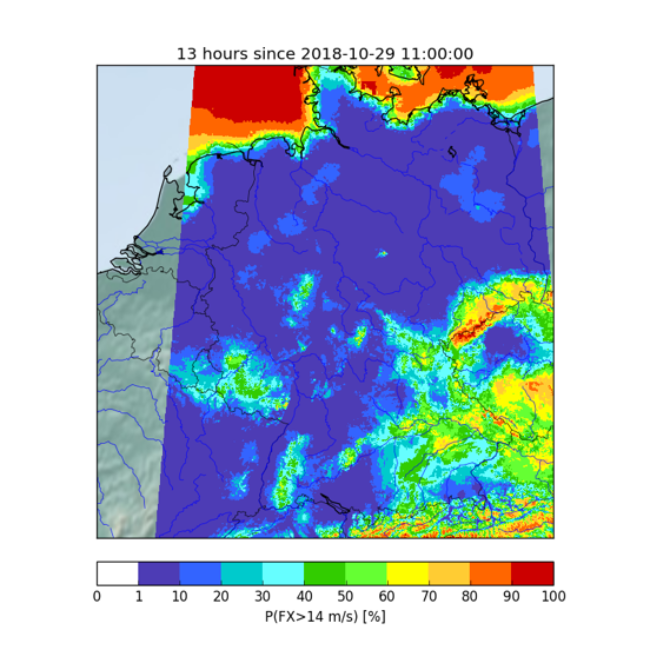
The October 2020 NPG Paper of the Month award goes to Reinhold Hess for the paper “Statistical postprocessing of ensemble forecasts for severe weather at Deutscher Wetterdienst“.
Ensemble Forecasting rose with the understanding of the limited predictability of weather. In a perfect ensemble system, the obtained ensemble of forecasts expresses the distribution of possible weather scenarios to be expected. However, operational forecasts of near-surface weather elements are often underdispersive and underestimate forecast errors.
At Deutscher Wetterdienst (DWD) a MOS system has been developed, that corrects for systematic errors of the numerical ensemble systems ECMWF-ENS and COSMO-D2-EPS. It calibrates probability forecasts to observed relative frequencies with a focus on severe weather. The calibrated event probabilities can be used for qualified decisions in terms of cost-loss evaluations that relate forecasts of harmful weather to economic value.
The basic concept of the MOS system presented in the paper is to use ensemble mean und spread as predictors in multiple linear and logistic regressions. The use of ensemble products as predictors instead of processing each ensemble member individually prevents difficulties with underdispersive statistical results and underestimated errors especially for longer forecast horizons. During multiple regressions, the system selects most relevant predictors based on statistical tests and is able to correct even for conditional biases, therefore. It is possible to use the latest available observations as predictors for short-term forecasts or to use the previous statistical forecasts as predictor for the next time step in order to exploit the persistency of the weather.
Extreme events are most relevant for meteorological warnings. They are (fortunately) rare, however, and long time series are required to capture a sufficiently large number of observed events in order to derive statistically significant estimations. Currently, time series of 8 years of ensemble and observation data are used for training. Model changes have been found less harmful than insufficient data. Extreme events with 40 mm or 50 mm precipitation per hour rarely appear at certain stations, which may result in statistical models that permanently predict 0% probability. Clusters of stations are gathered for a combined modelling of rare events, therefore. The clusters are defined according to the climatology of the stations. In order to compute statistical forecasts on a regular grid, the MOS-equations of the clusters are evaluated for locations apart from the training and observation sites. Figure 1 shows resulting gridded forecasts of wind gust probabilities as an example. Strong gusts appear most probably over sea and at higher elevations according to this forecast.