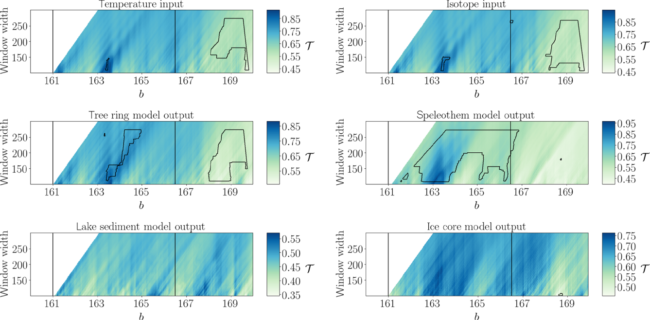
This month the NPG Paper of the Month award is achieved by Jaqueline Lekscha and Reik Donner for their paper “Detecting dynamical anomalies in time series from different palaeoclimate proxy archives using windowed recurrence network analysis” (https://npg.copernicus.org/articles/27/261/2020/).
Jaqueline Lekscha did her PhD in physics at the Potsdam Institute for Climate Impact Research and Humboldt University Berlin. Her main research focus was on further developing and applying windowed recurrence network analysis to palaeoclimate time series. She now works for Project Management Jülich in the field of Sustainable Development and Innovation.
Reik Donner currently works as a professor for mathematics (data science and stochastic modeling) at Magdeburg-Stendal University of Applied Sciences, Germany, in 2018. He has received the EGU NP Division Outstanding Young Scientist Award in 2011, and has been serving the NP division as scientific officer for time series analysis and big data since 2012. His main research interests comprise the development and application of complex systems methods for analyzing time series data especially from different geoscientific disciplines.
Paleoclimate proxy time series from archives such as, for example, trees, lakes, speleothems and ice cores offer the possibility of inferring information on past climates. Applying non-linear methods of time series analysis to such data sets can therefore yield valuable insights into past climate dynamics. Among others, the concept of windowed recurrence network analysis (wRNA) has been previously shown to provide a versatile tool in the palaeoclimate context. The basic idea of RNA is to transform a time series into a network based on proximity relations among sequences of observations representing state vectors in a reconstructed state space. The resulting network’s structural characteristics can then be related to the dynamics of the underlying system. Using a sliding windows approach, i.e., splitting the time series into multiple mutually overlapping windows and performing the analysis for each window separately, allows detecting changes in the system’s dynamics. Accordingly, analysing proxy time series using wRNA offers a new statistical-dynamical perspective on past climate variability. However, previous studies have also indicated that the robustness of the obtained results can differ substantially among proxies from different types of palaeoclimate archives.
In our article, we systematically test the suitability of wRNA for identifying temporal changes in the nonlinear dynamical characteristics of a selection of some of the most common types of palaeoclimate proxies. For this purpose, the framework of forward proxy modelling is used. Proxy system models are mathematical models that describe the physical processes underlying a proxy archive and aim at simulating the dynamics of a certain proxy variable given some climatic input variables. In particular, they can be interpreted as specific (non-linear) filter applied on the input variables. Note that common palaeoclimate research takes the inverse approach of inferring information on the (unknown) climate conditions based from the observed proxy values. Here, we address the question of how well changes in the nonlinear dynamics of the climate system can be identified based on corresponding analyses of the proxies.
We study four intermediate complexity models to simulate tree ring width, a lake proxy variable, and speleothem and ice core characteristic oxygen isotope ratios. In a first step, we create artificial input time series with different properties and analyse their properties using wRNA. To identify dynamical anomalies in the results, we use an areawise significance test developed in a previous study (Lekscha & Donner, Proc. R. Soc. A, 2019). In a second step, we process the input time series through the different proxy system models and apply the same analysis. Thereby, we can compare the respective areawise significant dynamical anomalies (detected using wRNA) between the input and the model output time series. Additionally, we compare the analysis results of different stochastic and deterministic time series with and without applying some general filters like moving averages or exponential smoothing.
We find that the variability of the network transitivity as a particularly relevant indicator (related to the effective degrees of freedom of the dynamics in the spirit of a generalized fractal dimension) is altered for stochastic input time series while being rather robust for deterministic input. More specifically, we observe that anomalies may be missed by proxies from tree and lake archives after the non-linear filtering by the corresponding proxy system models. For proxies from speleothems, we observe additional falsely identified significant anomalies that are not present in the underlying input time series. Finally, for proxies from ice cores, the wRNA results show the best correspondence to those for the input data. Our results help improving the interpretation of wRNA results obtained from real-world palaeoclimate time series and provide a general strategy for testing other types of nonlinear time series analysis approaches in a palaeoclimate context. Most notably, they highlight the importance of more systematically studying the effects of non-linear filtering on the results of wRNA (and likely also other approaches serving a similar purpose) on the one hand, and the relation between the autocorrelation of a time series and the nonlinear analysis results on the other hand.