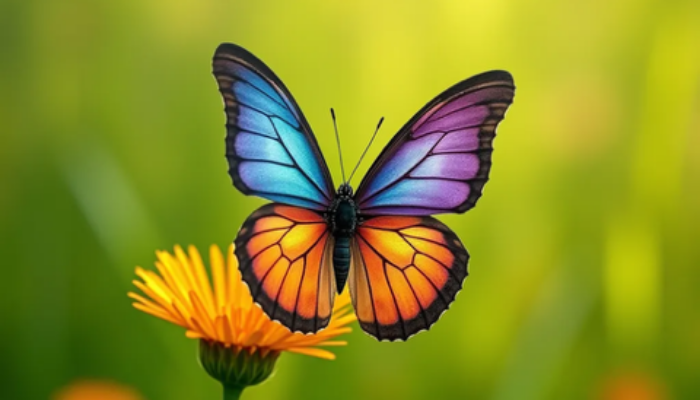
About the revolution of generating butterflies
Imagine the most vibrant butterfly you can conceive. Congratulations – you’ve just achieved what generative AI does! This technology can create images from simple text descriptions, revolutionising image generation. But as geoscientists, how can we use it to improve the prediction of chaotic system like our weather? How can it help us to discover properties crucial for weather prediction and climate projections? And what does it learn from data?
These are the questions, we explore in our recent publication, T. S. Finn et al., 2024. In this study, we trained a type of generative AI called denoising diffusion models, which are commonly used for image generation. Instead of creating realistic images, we tasked them to generate data from a simple chaotic system known as the Lorenz 1963 system—a mathematical model often used to describe the chaotic behaviour of weather. This system has three variables, and when we track its states over long periods, we can get butterfly-shaped patterns, as can be seen in the main picture.
We trained the model to produce data mimicking these states. After training, we found that it could generate realistic states without needing to track the system step-by-step over long periods. The model learns to efficiently sample possible states of the system, providing us with valuable information for climate-like systems. Hence, this technology can be particularly valuable for handling chaotic systems like the weather. These systems are notoriously difficult to predict especially in high dimensions, and this capability of generating realistic states could improve both short-term forecasts and long-term climate projections.
On the efficiency of denoising diffusion models for chaotic systems
To understand why the model performs so efficiently for chaotic systems like Lorenz 1963, we need to dive into how it works: it is trained to transform random noise into clean, structured data. It do this transformation iteratively similar to how chaotic systems evolve forward from initial conditions. We believe that, because of this similarity, the generative procedure mirrors the nature of weather-like systems, and this is why the AI can produce these states so efficiently.
A key question to further understand the efficiency is: what exactly does the AI learn? Surprisingly, we found that the model learns to extract features that can be combined to reconstruct the fundamental equations of the Lorenz 1963 system. This is remarkable because the model wasn’t shown how states evolve over time, it learned the key features of the system on its own. This ability to identify key features within denoising diffusion models could open us a door to improve our understanding of the system’s dynamics from pure data.
Learning the system’s inner workings is not only interesting on its own, but has also practical use beyond just generating nice samples. The model can be retrained to predict the evolution of the states or can be integrated into a data assimilation, where predictions are updated with observations to improve their accuracy. In our tests, both the forecasting and data assimilation models outperformed traditional methods, e.g., by exhibiting much more stable forecasts or improving the needed uncertainty estimates. Their application to forecasting and data assimilation provide us a glimpse of how we can use these models.
Applications of denoising diffusion models in geoscience
During the last two years, concurrently to our work, denoising diffusion models have shown to work on broad applications beyond chaotic systems like Lorenz 1963. In geoscience, and especially meteorology, they are increasingly being applied to tackle a range of challenges. For example, they are employed for precipitation nowcasting (Leinonen et al., 2023), slowly replacing older and more-brittle AI systems. Furthermore, they have been used to increase the resolution of meteorological data with direct applications to forecasting (Mardani et al., 2024), climate projections (Bassetti et al., 2024), and an increased use of satellite observations (Barth et al., 2024).
Perhaps most remarkably, denoising diffusion models can improve forecasts of climate components like the atmosphere (Price et al., 2024) and sea ice (Finn et al., 2024), and efficiently generate large forecasting ensembles (Li et al., 2024). By doing so, they can outperform traditional numerical weather prediction models, which have long been the gold standard for weather forecasting. These models can hence represent another significant shift in the application of AI for forecasting purposes.
In summary, generative AI provides us an efficient novel way to explore, understand, and predict complex phenomena like weather. Thereby, it can do more than just generating picturesque butterflies – it promises significant advances, offering us a unique opportunity into improving our understanding of such systems and their inner workings.
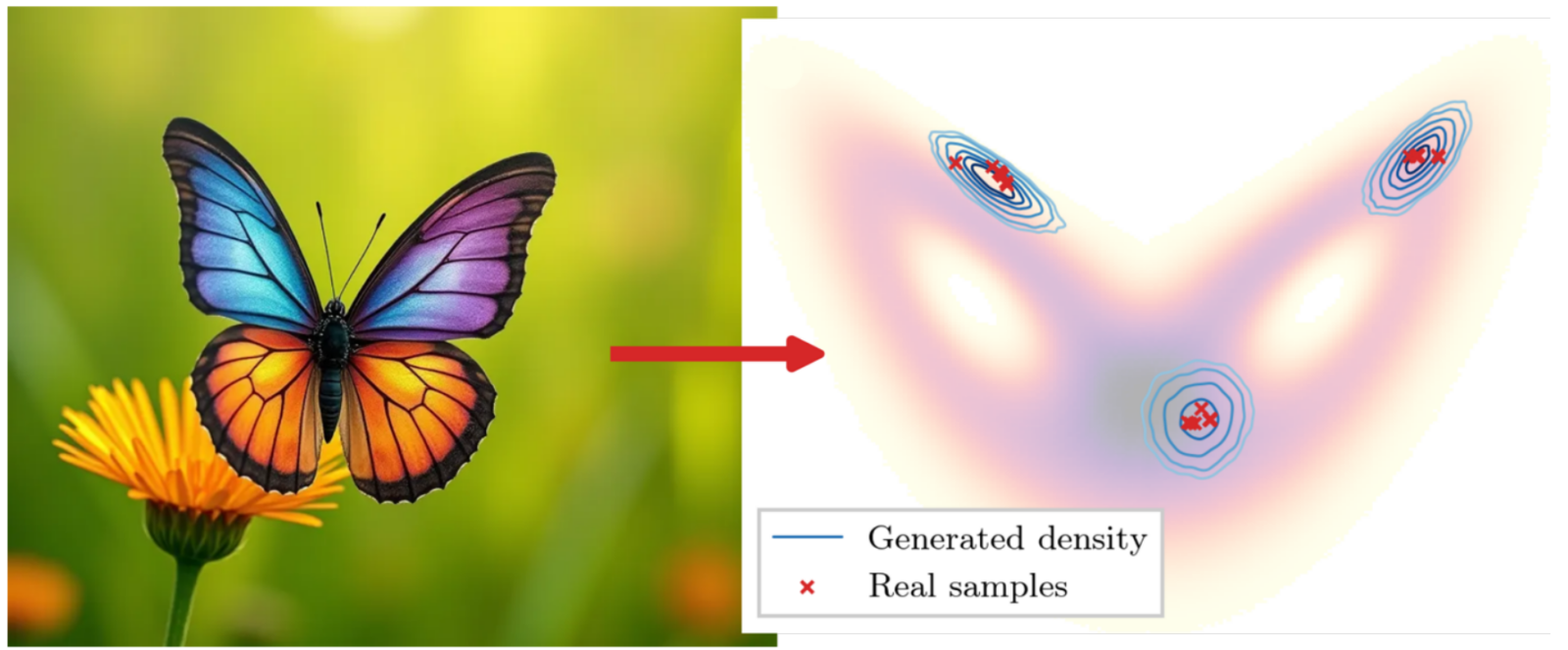
We investigate how denoising diffusion models, a popular type of AI to generate images, like the one shown on the left (generated with the FLUX.1 [dev] model from black forest labs), can be used to discover properties important for weather forecasting and climate projections. Here, as shown on the right, we trained such an AI to learn states from the Lorenz 1963 system, which, tracked over a long period, produces butterfly-shaped patterns. After the training, we used the AI to for example generate uncertainty estimates as they are needed to correct forecasts with observations.
References
Barth, A., Brajard, J., Alvera-Azcárate, A., Mohamed, B., Troupin, C., & Beckers, J.-M. (2024). Ensemble reconstruction of missing satellite data using a denoising diffusion model: Application to chlorophyll a concentration in the black sea. EGUsphere, 1–26. https://doi.org/10.5194/egusphere-2024-1075
Bassetti, S., Hutchinson, B., Tebaldi, C., & Kravitz, B. (2024). DiffESM: Conditional emulation of temperature and precipitation in earth system models with 3D diffusion models. Journal of Advances in Modeling Earth Systems, 16(10), e2023MS004194. https://doi.org/10.1029/2023MS004194
Finn, T. S., Durand, C., Farchi, A., Bocquet, M., Rampal, P., & Carrassi, A. (2024). Generative diffusion for regional surrogate models from sea-ice simulations. Accepted for Journal of Advances in Modeling Earth Systems, https://doi.org/10.22541/au.171386536.64344222/v1
Leinonen, J., Hamann, U., Nerini, D., Germann, U., & Franch, G. (2023). Latent diffusion models for generative precipitation nowcasting with accurate uncertainty quantification (No. arXiv:2304.12891). arXiv. https://doi.org/10.48550/arXiv.2304.12891
Li, L., Carver, R., Lopez-Gomez, I., Sha, F., & Anderson, J. (2024). Generative emulation of weather forecast ensembles with diffusion models. Science Advances, 10(13), eadk4489. https://doi.org/10.1126/sciadv.adk4489
Mardani, M., Brenowitz, N., Cohen, Y., Pathak, J., Chen, C.-Y., Liu, C.-C., Vahdat, A., Nabian, M. A., Ge, T., Subramaniam, A., Kashinath, K., Kautz, J., & Pritchard, M. (2024). Residual corrective diffusion modeling for km-scale atmospheric downscaling (No. arXiv:2309.15214). arXiv. https://doi.org/10.48550/arXiv.2309.15214
Price, I., Sanchez-Gonzalez, A., Alet, F., Andersson, T. R., El-Kadi, A., Masters, D., Ewalds, T., Stott, J., Mohamed, S., Battaglia, P., Lam, R., & Willson, M. (2024). GenCast: Diffusion-based ensemble forecasting for medium-range weather (No. arXiv:2312.15796; Version 2). arXiv. https://doi.org/10.48550/arXiv.2312.15796