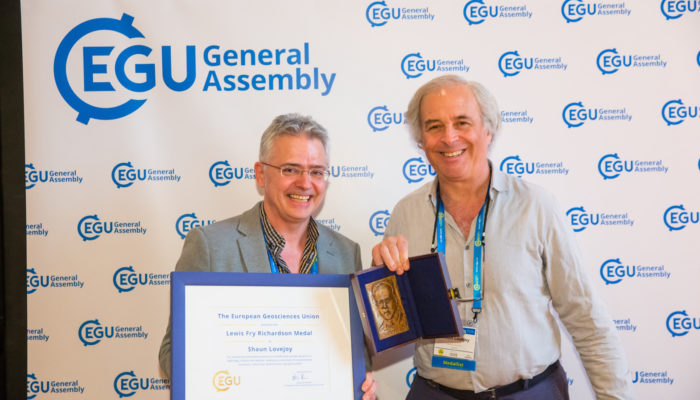
Today’s NP Interviews hosts the Lewis Fry Richardson Medallist Shaun Lovejoy.
Shaun has degrees in physics from Cambridge and McGill University; he has been a McGill professor since 1985. For four decades, he has developed fractal, scaling ideas in the geosciences, contributing to advances in cascade processes, multifractals, anisotropic scale invariance, space-time multifractal modeling as well as to the empirical establishment of wide range atmospheric scaling. He co-founded the Nonlinear Processes in Geosciences Division at the EGU, and the Nonlinear Processes in Geophysics journal. He was president of the AGU Nonlinear Geophysics focus group (2008-2012) and of the EGU NP Division (2013-2016).
Firstly, what were your feelings when you received the news that you received the Richardson medal?
Of course, I was happy: in fundamental science, it can be very lonely and this brought me attention! It was a rare moment of gratification. At the same time, I didn’t want to see it as simply a “pat on the back” for a long and productive career. My goal has always been to change the way we view the world, to change science. I see the medal is a step in the direction of bringing the science into the “mainstream”. I could also add that concretely, since I’m the only geo-scientist in the McGill physics department, my colleagues don’t have much idea what I’ve been doing for the last 34 years! Therefore, I haven’t received much institutional support. Since the university administration pays attention to international honours such as the Richardson medal, maybe this will change.
You received it for “pioneering and leading research on multifractal cascade dynamics in hydrology, climate and weather, leading to a new class of comprehensive stochastic, rather than deterministic, sub-grid models”, but when you started a career in nonlinear geophysics in the 1970’s, the field didn’t yet exist. How did you get involved with it?
Before answering the question, I found the committee’s reference to “subgrid models” a bit odd since the models I’ve developed are scaling and hence go way beyond “subgrid scales” right up to scales of planetary extent!
I got into the field in a way that would be virtually impossible today; so for the sake of younger readers I’ll give some details. I started graduate work in high energy physics at McGill in 1976, but in spring 1977 switched to atmospheric physics supervised by Geoff Austin. My PhD topic was the remote sensing of rain from radar and satellites. I soon realized that the variability of precipitation was way beyond anything that had been modelled before – either by standard numerical weather models or by stochastic models. I was just coming to this realization in December 1977, when my mother – an artist – gave me a copy of Mandelbrot’s book: “Fractals form and chance”. I vividly remember the epistemic shock of the stunning computer graphics that included fractal clouds and landscapes. Heavily influenced by Mandelbrot’s book, I soon started making fractal analyses of radar rain data and I developed fractal model of rain based on Levy random variables. When I finally submitted my thesis in November 1980, the first half contained material from three fairly standard papers on remote sensing while the other half was on the fractal analyses and models of rain. Since the first half had already been peer reviewed, I was confident that the thesis would be “rubber stamped” by the external examiner. I therefore busied myself preparing for a Canadian government funded post-doctoral fellowship at the Météorologie Nationale in Paris. But disaster struck: my thesis was rejected! The rejection was not based on a scientific criticism of the contents, but rather on an “unacceptable thesis structure”. Clearly uncomfortable with the fractal material, the external examiner claimed that it was unrelated to the remote sensing part and hence that the structure was badly flawed. This was essentially an invitation by the examiner to remove the material that he thought I would later regret. McGill gave me two options: either I follow the referee’s strictures and remove the fractal material, or I could contest it.
In order to get advice, in January 1981, I therefore visited Mandelbrot at his IBM Yorktown heights office. While Mandelbrot was enthusiastic about the material, after consulting with his friend and colleague Erik Mollo-Christensen at MIT, he advised me to follow the referee’s strictures: remove the fractal material and then to separately publish the highlights of the fractal part in the open literature. I followed this advice, amputated the fractal material and resubmitted the thesis. By the summer, I defended my PhD, arrived in Paris and submitted to Science some of the excised fractal material: a paper on area-perimeter relations for clouds and rain (published early in 1982).
Can you describe what it was like back then?
By the early 1980’s, the nonlinear revolution was just getting seriously underway and it was an exhilarating time to be a geoscientist. In deterministic chaos, universality had just been discovered: the celebrated Feigenbaum constant. For the first time, it was thus possible to compare the predictions of strongly nonlinear models against real world phenomena. Practical data analysis techniques such as the Grassberger-Procaccia algorithm were being developed and were widely applied in attempts to reconstruct phase spaces with “strange” (fractal) attractors. Fractals were also turning up not only in phase space, but everywhere in real space: coastlines, earthquakes and clouds. In 1982, Mandelbrot’s lavish update “The fractal geometry of Nature” appeared. Nothing seemed impossible, it even seemed that we were on the verge of solving the ancient problem of turbulence. Giving expression to the reigning ambiance, Predrag Cvitanovic proclaimed: “junk your old equations and seek guidance in clouds repeating patterns” (in “Universality in Chaos”, 1984).
Weather prediction was transitioning from an art-form to a quantitative science. Satellites, aircraft and other remote and in situ data collection technologies were amply demonstrating not only that atmospheric variability spanned huge ranges of scale in both space and time but that the variability was highly intermittent: it was spiky and displayed sudden transitions. By the early 1990’s, developments outside of science refocused all attention – and funding – to applications. Fundamental science was increasingly viewed as an unnecessary luxury.
What were the main ideas that motivated your research?
I was struck by fractals, but I entered the field more from the empirical than the theoretical side. At the time, most of the scientists working on chaos and fractals were primarily interested in the developing the mathematics. In contrast, my main attraction to fractals was to deal with the real world problem of understanding and modelling atmospheric variability whose full scaling complexity was only then being fully displayed and realized thanks to new technologies. Working with Schertzer at the Météorologie Nationale, we realized that in spite of Mandelbrot’s landmark books, that systematic applications of the fractal idea to the atmosphere would require several new developments. For example, at the time, there was no general statement of the fractal principle: fractals were nearly always “self-similar”. Zooming into a random self-similar fractal, we would typically find smaller structures similar to larger ones: self-similar systems are statistically isotropic. However vertical sections in the atmosphere are highly stratified so that the atmosphere cannot be self-similar: its scaling is highly anisotropic. We were forced to formulate scale invariance as a very general anisotropic scale symmetry principle: “Generalized Scale Invariance” (GSI, 1985). We concluded that atmospheric dynamics led to anisotropic multifractals with the generic multifractal process being a cascade process. It’s barely an exaggeration to say that I’ve spent the last 35 years working out the consequences and trying to empirically validate this basic paradigm.
What were the key people that contributed to the increase of your motivation in nonlinear geophysics?
I owe my initial graduate student freedom and encouragement to my supervisor Geoff Austin; and the confidence to continue in the face of a very uncertain academic future to Mandelbrot. However, my main debt is to Daniel Schertzer with whom I collaborated for over 30 years. Austin was also very supportive when, in 1985 after my post doc in Paris was over, he supported me to come back to McGill. Imagine trying to get a first academic appointment outside of any established area of science – in my case nonlinear geophysics. Getting a tenured position was really a bit of a miracle because then – and even more so now – no university department would ever hire someone that didn’t fit into a conventional niche. Today it would be unthinkable and back then it was only possible because the Canadian government had recently set up its “University Research Fellowship” programme. These fellowships were funded for up to ten years and were awarded in a Canada-wide competition where the only criterion were publications and support letters. Since the money came from outside of McGill, I wasn’t competing with anyone else in the department for a rare departmental position; I was a free “extra”. In today’s world I would never have been even considered for a position in such a nonconventional area: there’s no room for any new fields.
How has nonlinear geophysics changed since the 1970’s?
Although there were workshops focusing on specific areas of nonlinear geophysics – including a series of four organized by Schertzer and I starting at McGill in 1986 on “Nonlinear VAriability in Geophysics” (NVAG), the event that really put nonlinear geophysics on the map was a European Geophysical Society session at the general Assembly in Bologna, in 1988. The session was organized by Catherine Nicolis and Roberto Benzi and featured a wide range of nonlinear themes. Excitement was in the air and strongly supported by European Geophysical Society (EGS) director Arne Richter, it was decided to organize several nonlinear sessions at the next meeting in Barcelona, where the Nonlinear Processes (NP) division was started.
Nonlinear processes in geophysics has always been a collection of nonlinear paradigms; at the beginning the main ones being deterministic chaos and fractals although nonlinear waves (e.g. solitons) were also important. What brought the nonlinear processes in geophysics scientists together was their common conviction that in their respective geophysical subfields, that nonlinearity was not being taken seriously enough. Remember that at the same time – throughout the 1980’s – that brute force numerical techniques were becoming the norm, the prototypical example being numerical weather prediction. This numerical, computer revolution was slowly transforming field after field into highly applied sciences where instead of trying to understand the weather, climate, hydrosphere etc., the focus was more and more on large scale numerics and for data, on remote sensing. In other words, the science was increasingly subservient to technology and to applications such as numerical weather prediction and a little later, the climate.
I discuss the quarter century long decline of fundamental science in my book arguing that it was related to increasing corporate control over publicly funded research. Fundamental science was not considered to be profitable enough. Even when the computer managed to realistically simulate, it still didn’t deliver understanding. A common NP motivation was – and has remained – the desire to fundamentally explain nonlinear phenomena. At first, the main paradigms – deterministic chaos and scaling / fractals were both hegemonistic – each was convinced that it provided an overarching framework, theory for understanding many areas of geoscience. Since in geophysical applications, the fractal, scaling approach was stochastic, the difference between the approaches could starkly be framed as the debate: “deterministic versus stochastic chaos”. Indeed, this was the title of a session at the 1994 EGS general assembly that brought together exponents of the two approaches.
Over the last decades, Nonlinear Processes in Geophysics has been the area where much of the theoretical, fundamental geoscience has occurred. Perhaps the most successful example is the Natural Hazards division that spun off from the NP division around the mid 1990’s. In the last ten years, perhaps the most important new NP paradigm has been network theory developed and applied especially by Jurgen Kurths, Henk Dijkstra and Anastasios Tsonis.
So, how has your work changed this field? Why scaling laws and fractals are so important for understanding our Earth system?
That’s a very tough question! In most areas of science, the measure of success is something fairly tangible: for example an uncontested empirical discovery or a model that becomes the standard in a field. My contributions have not only been fundamental, but have also been misunderstood – and hence opposed – as being in contradiction to more mainstream approaches.
I have my own simple four-step model of how fundamental science advances, at least the first steps apply roughly to my situation:
Stage 1) Business as usual
At first the new idea is ignored; science goes on in a “business as usual” mode.
Mandelbrot’s fractal geometry paradigm initially enthused many (including me): the idea of reducing a coastline or a cloud to a unique fractal dimension was seductive. However, it turned out that the initial isotropic (self-similar) fractal set/fractal function paradigm with a unique exponent (e.g. the fractal dimension) was not directly applicable to geosystems. when in 1993 we showed that the topography was nearly perfectly multifractal and that this explained the earlier dispersion of empirical dimension estimates. By then, the mainstream had already lost interest, effectively throwing out the baby with the bathwater.
Stage 2) Criticism
If the new idea persists and demands a reaction, the second step – often years later – is to criticize it: the new idea is obviously wrong.
The criticism was particularly strong in the 1990’s when my grant applications frequently suffered from very hostile referees. I remember one in particular, around the mid 1990’s an anonymous referee – probably a geographer – who exhorted me to “do physics not geometry”. Unfortunately he had mistakenly lumped my approach in with fractal geometry – even though Schertzer and I had been doing our best to go beyond geometry and to make dynamical multifractal models.
Stage 3) Obvious
If in spite of the criticism, arguments and data continue to accumulate to support the alternative theory or model, then all of a sudden, it becomes an obvious truth – even a triviality. It can then be dismissed as having no important consequences.
As satellite data improved and – perhaps more importantly became accessible over the internet – and NWP and GCMs became larger, it became increasingly obvious that they were all scaling over huge ranges. The main resistance was from turbulence theorists that clung (and still cling!) to the idea that isotropy – not scaling – is the primary symmetry and that the atmosphere is divided into 3D isotropic turbulence (small scales) and 2-D isotropic turbulence (large scales).
If scaling becomes “obvious”, then one of the consequences is that we can clarify atmospheric dynamics. Rather than the dichotomy weather and climate (conceived conventionally as a kind of average weather), we have a new intermediate “macroweather” regime, at time scales beyond the lifetime of planetary structures of about 10 days. The new framework is thus weather, macroweather and climate with typical fluctuations increasing, decreasing and again increasing with time scale.
In place of fuzzy concepts based on theoretical preconceptions, we have a new framework based on empirical evidence. More and more scientists are finding this categorization helpful and it does indeed make atmospheric science much clearer. Indeed, it finally puts on an objective scientific basis the routine use of the otherwise arbitrary monthly scales to define “anomalies” and the 30 year scale to define “climate normals”. These scales turn out to correspond to the transition times between regimes (in the industrial epoch due to anthropogenic forcings, the transition is at 20-30 years, in the pre-industrial epoch the macroweather – climate transition time was at longer multicentennial or multimillennial scales).
Stage 4) Consequences
The very last stage – the most difficult of all – is for the community to accept that there are non trivial scientific consequences that must be taken into account.
To gain serious acceptance of scaling requires accepting the consequences. To return to your question about the impacts of scaling, there needs to be consequences that no one can deny and that are important enough to make people change the way that they do science. In other words, we need a “killer app” for scaling! It’s possible that we’ve recently found it. We’re discovering that beyond the atmospheric deterministic predictability limit (of about 10 days) that the atmosphere behaves as a stochastic but nearly linear system.
Even the GCMs behave this way at scales of a month and longer – at least when subjected to the forcings prescribed by the IPCC. It turns out that to take advantage of this discovery, scaling is useful, even essential. Currently it can be used to make the most accurate macroweather temperature forecasts (e.g. monthly, seasonal or annual), or to make decadal, multidecadal projections (where human actions are important, future scenarios are required), with lower uncertainty. In both cases, scaling is needed.
Why we should concentrate on the stochastic rather than the deterministic?
The choice between a stochastic or a deterministic approach should not be ideological, it should be scientific, it should depend on the problem at hand. It’s like statistical mechanics versus continuum mechanics and thermodynamics, the choice should depend on the problem: in principle both methods work. That being said, the higher level stochastic laws that apply in the atmosphere express the collective behaviour of large numbers of interacting components. Rather than attempting to mechanistically model each bump and wiggle on each cloud, one attempts to work the statistics of the behaviour of huge numbers of structures. As science progresses, we’ll increasingly find ways to take advantage of the higher level stochastic laws.
What do you wish for the future and how young/established researchers can contribute to the growth of the nonlinear geophysics/geosciences?
The world is at a crossroads. There are many deep social and environment problems, not the least of which is climate change. Science is needed more than ever to understand and to solve these problems, to help avoid catastrophe. While the need for research has never been stronger, in many countries – including my own country Canada – research budgets have been shrinking whereas they must be drastically increased.
Geoscience has a special role to play since many of the problems we face are environmental. Within geoscience, there is a growing imbalance between applied and fundamental research so that many of the resources spent on applications is wasted. Correcting this imbalance will be particularly helpful for Nonlinear Processes since it represents the fundamental part of geoscience, yet all science needs much stronger support from society. If young scientists can help in this struggle, then they can play the role that is needed to keep the planet livable.
I think that it’s also important to realize that the scientific world view is under an attack stronger than it has been for generations. We’re all familiar with the proliferation of “alternative facts” and “fake news”, but this is just the surface. Underlying these phenomena is a disillusion with science and progress combined with a pervasive post-modern cognitive relativismthat undermines science’s claims to truth. If truth is no more than a social construct, then who needs science? In many ways, we’re entering a brave new world and I’m counting on the young generation to rise to the challenge!