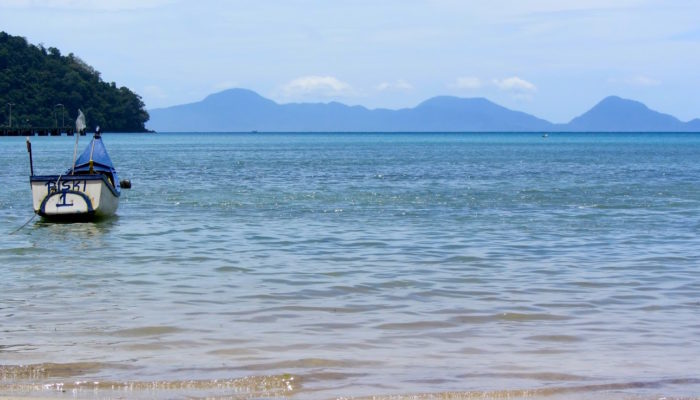
A Natural Hazards Earth System Sciences (NHESS) paper proposes a new sampling method for reducing uncertainty in geographical location in seismic hazard risk analysis, with implications for the insurance industry and risk communication in general.
Globally, rapid urbanisation is increasing the risk of exposure of human populations and infrastructure to natural hazards, including earthquakes (seismic hazards). While the exact magnitude and frequency of earthquakes cannot be predicted, the peak ground acceleration can be predicted through the hazard component of probabilistic seismic risk analysis and is widely used in the insurance and the reinsurance (who insure insurance companies) industries. Probabilistic seismic risk analysis is a useful tool in quantifying exposure risk to earthquakes, however there are many uncertainties associated with it.
For example, one of the major challenges for insurers when predicting ground motion is that ground motion can vary strongly between different geographical locations within a region, such as in western Indonesia where this paper is focused. In the insurance industry, identified risks (such as a commercial building which are called ‘risk items’) often lack precise geographical location information due to geocoding issues. Additionally, geographical location datasets are often large, taking a long time for simulations used within probabilistic seismic risk analysis models to align or ‘converge’, and estimate how frequently losses occur. Yet, these Monte Carlo simulations (which use many random samples to obtain estimates of uncertainty), require fast run times for insurance underwriting. To tackle these issues, the authors of this paper Scheingraber and Käser (2020) use an adaptive location uncertainty sampling scheme to improve efficiency in model convergence and apply this sampling scheme to spatial hazard in western Indonesia.
In contrast to a simple Monte Carlo model, where all risk items have the same location uncertainty sample size, the paper proposes a new adaptive scheme which decides the (unknown) location sample size of individual risk items within a known administrative zone. Three criteria are used to decide sampling: 1) the ‘loss rate variation’ within the zone, which is the spatial variation in how often losses are incurred; 2) the number of risk items within the zone and 3) the individual value of those risk items. The sampling frequency is defined by the minimum of these three criteria. Any of the three criteria can predict that a particular risk item has a low impact on loss uncertainty. For example, if a risk item (take the example of a commercial building) with an unknown geographical location coordinate has a low insured value, it will have a relatively low impact on loss uncertainty, even if the number of such buildings within the zone is high.
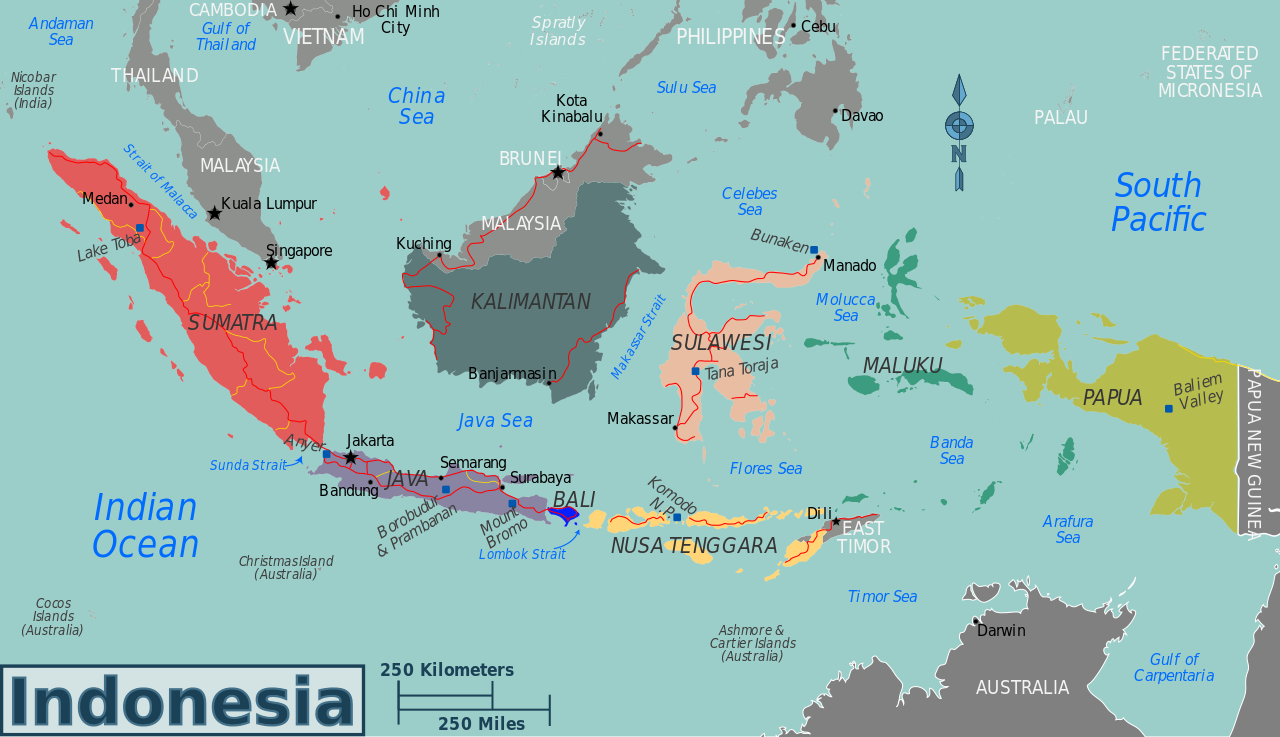
Image credit: Peter Fitzgerald
The seismic risk model used in the paper shows the seismic risk on the islands of Sumatra, Java and Kalimantan in western Indonesia. Peak ground acceleration, the maximum ground acceleration that occurs during earthquake shaking, is used as an indicator of seismic hazard. While seismic hazard is high on the island of Sumatra due to the effect of the Sumatra fault zone, seismic hazard is low on Kalimantan as there are no known crustal faults on this island. Administrative geographical zones with higher seismic hazard have more seismicity data available in order to capture the spatial seismic hazard variation. This variation in seismic hazard spatially is represented by the coefficient of variation. Generally, the coefficient of variation is higher in zones close to shallow faults and Scheingraber and Käser suggest that this is because location uncertainty can be high in these zones.
The new, adaptive approach is tested against a simple Monte Carlo simulation for the seismic risk model of western Indonesia to see whether location uncertainty is reduced, and whether model simulation is faster. Analysing the estimates of probable maximum losses, with a return period of 100 years for 20 risk items with unknown locations, the adaptive location uncertainty sampling scheme reached the same error levels as simple Monte Carlo simulations, but with fewer potential risk location samples. The adaptive approach allows these simulations to converge faster than a simple Monte Carlo model, while being of a similar level of reliability in ‘loss frequency curve’ calculation. Depending on the risk items and relative standard error level, using the adaptive approach instead of a simple Monte Carlo model increases the speed of model convergence by 6% to 37%.
The paper’s findings are of particular importance as reliable ‘loss frequency curves’ are one of the key ways that the insurance industry measures risk. By increasing the speed of the model’s run time the adaptive location uncertainty sampling approach presented in this paper may improve our ability to incorporate location uncertainties in probabilistic seismic risk analysis. This would be a positive contribution to the growing need for efficient and effective communication of uncertainty to decision makers in industry, government, and the general public.