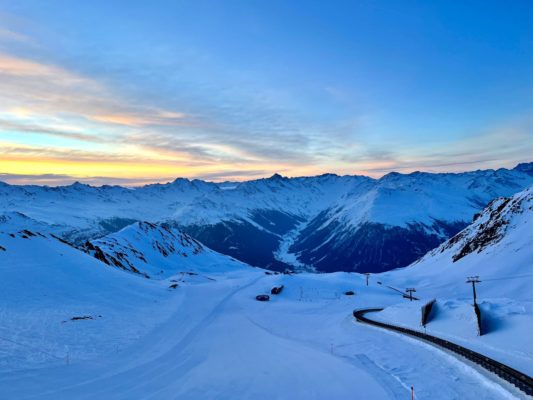
As scientists, we often encounter errors in our own work and in the work of others. As modelers, we call these errors “bugs”. Of course, they are not actual insects, but they definitely keep us awake from time to time. Even though everyone is aware of their existence, we rarely discuss them in a scientific context. In today’s post, I bring to you the work and journey of a snow scientist, Dr. Cécile Ménard, addressing the big elephant in the room: human errors.
Let’s talk about mistakes
Two years ago, at my first conference as a PhD student, I was surprised by the first talk of my session: “Human Error and Limited Scientific Progress in 24 Years of Snow Model Intercomparisons”. As an early-stage snow modeler, I was amazed by the fact that we were going to discuss limited scientific progress and human mistakes in a conference session dedicated to cutting-edge model developments: this is new!
The work presented and led by Dr. Cécile Ménard, from the University of Edinburgh, summarizes the main findings of an international effort to assess the performance of 27 models representing the Earth’s snow cover – the Snow Model Intercomparison Project (ESM-SnowMIP). For many, the title of the talk might be a synonym of failure. However, this work brings to light the true nature of the scientific process, composed of hypothesis, experiments and systematic analysis – all conducted by humans, who naturally make mistakes.
After the conference, I could not resist reaching out to Dr. Cécile Ménard. She agreed on an interview where I got to learn more about her work and, of course, some of the adventures behind the scenes. This is what I found.
The Snow Model Intercomparison Project (ESM-SnowMIP)
A significant part of the Earth’s surface is covered with snow, especially during the boreal winter (see the view of the Arctic from space in the image below). Due to its white and bright nature, snow reflects a significant part of the incoming solar radiation back to space. In addition, its low thermal conductivity protects the underlying ground or ice from extreme air temperatures. Therefore, snow is an important element of the Earth’s climate system. The extent and properties of the snow cover are estimated with the help of snow models, which can have different levels of complexity (e.g., snow can be described as a single layer of uniform properties or as a multi-layer structure composed by different types of snow). The development of snow models requires a comprehensive understanding of the physical processes taking place in the snowpack. In addition, the data collected at weather stations that monitor the weather conditions and the state of the snow is of utmost importance for model validation (see a picture of a weather station in the Alps in the featured image). Snow models are included in most global and regional weather and climate models and play an important role in predicting the consequences of global warming.
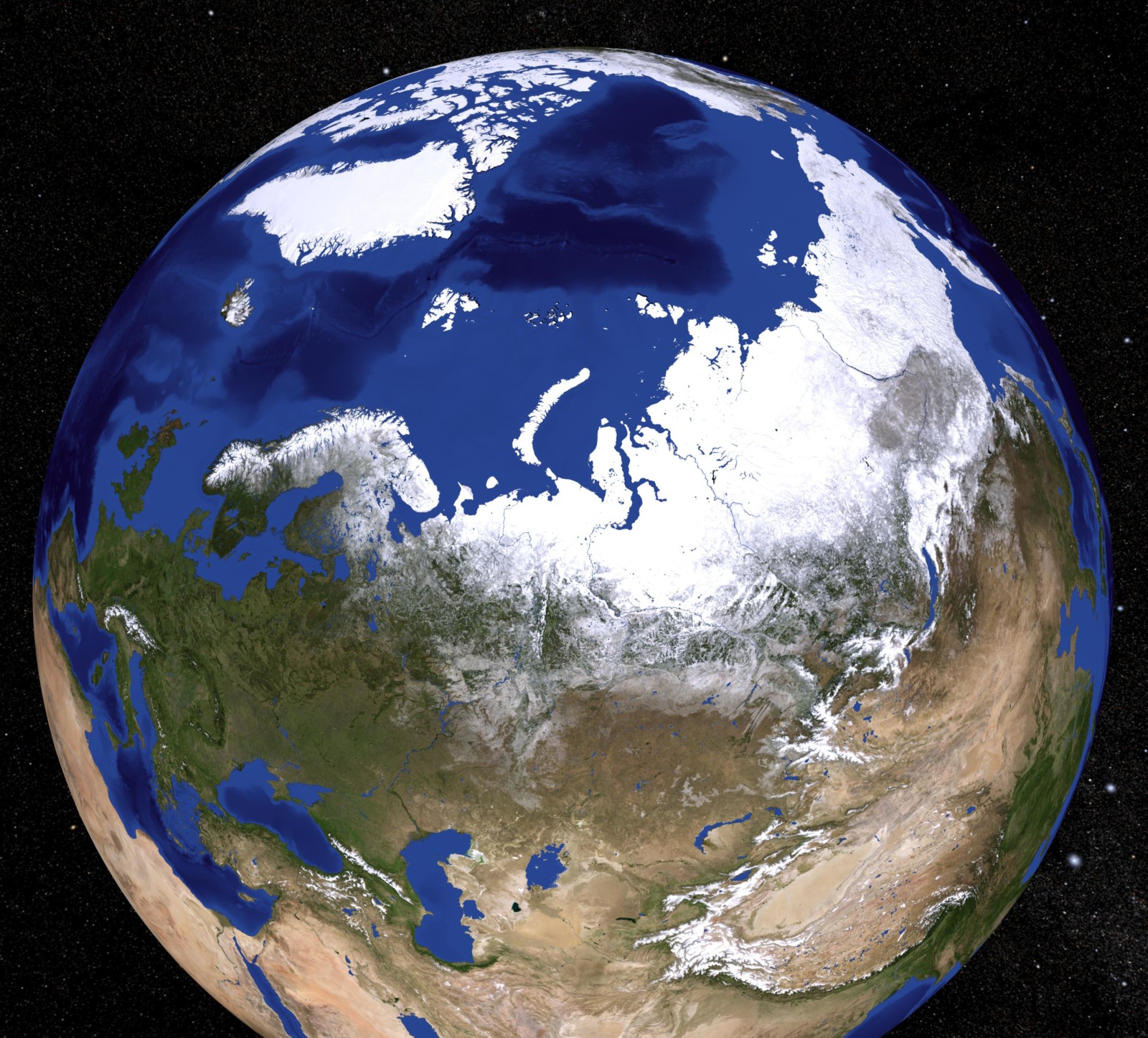
View of the Arctic from space. The picture shows the extent of the snow cover on the northern hemisphere in December. [Credit: NASA/Goddard Space Flight Center Scientific Visualization Studio, 2010]
The ESM-SnowMIP was the third of its kind. Since the previous one, significant model developments and extensive measurement campaigns were undertaken. The ESM-SnowMIP team was therefore expecting to see a better agreement between model results and measurements as well as to gain a clearer insight into the physical processes that needed further development. However, the resulting article published in the Bulletin of the American Meteorological Society, BAMS, pointed to a different direction. “I [could] not find any progress in the models”, says Ménard, the first author of the article, who has been studying snow for 15 years.
The quest for answers
In order to understand the models’ behavior, Ménard contacted all the modeling teams. Together, they found mistakes in the algorithms, undocumented assumptions and highly error-prone user interfaces. The models were corrected and re-evaluated. She found that the difference between measurements and some model results reduced by half. For other models, the average error reduced by a factor of five. Considering the effect of these mistakes on the models’ performance, the contribution of human error to the lack of scientific progress became obvious. After finishing a draft with her findings, Ménard recalls feeling unsure on how the modelers would react. Much to her surprise: “Everybody was so enthusiastic, I could not believe it”, she says.
In addition to human errors, the article mentions several scientific reasons for the lack of progress. For instance, the extreme conditions of cold and snowy environments hinder the acquisition of long-term and spatially varied experimental data (see a picture of a weather station in Antarctica in the image below). Moreover, the existing measurements are not sufficiently detailed to evaluate the most sophisticated models that resolve the multi-layer structure of snow. Therefore, even though there were improvements in the process representation, the accuracy of these new developments is difficult to assess.
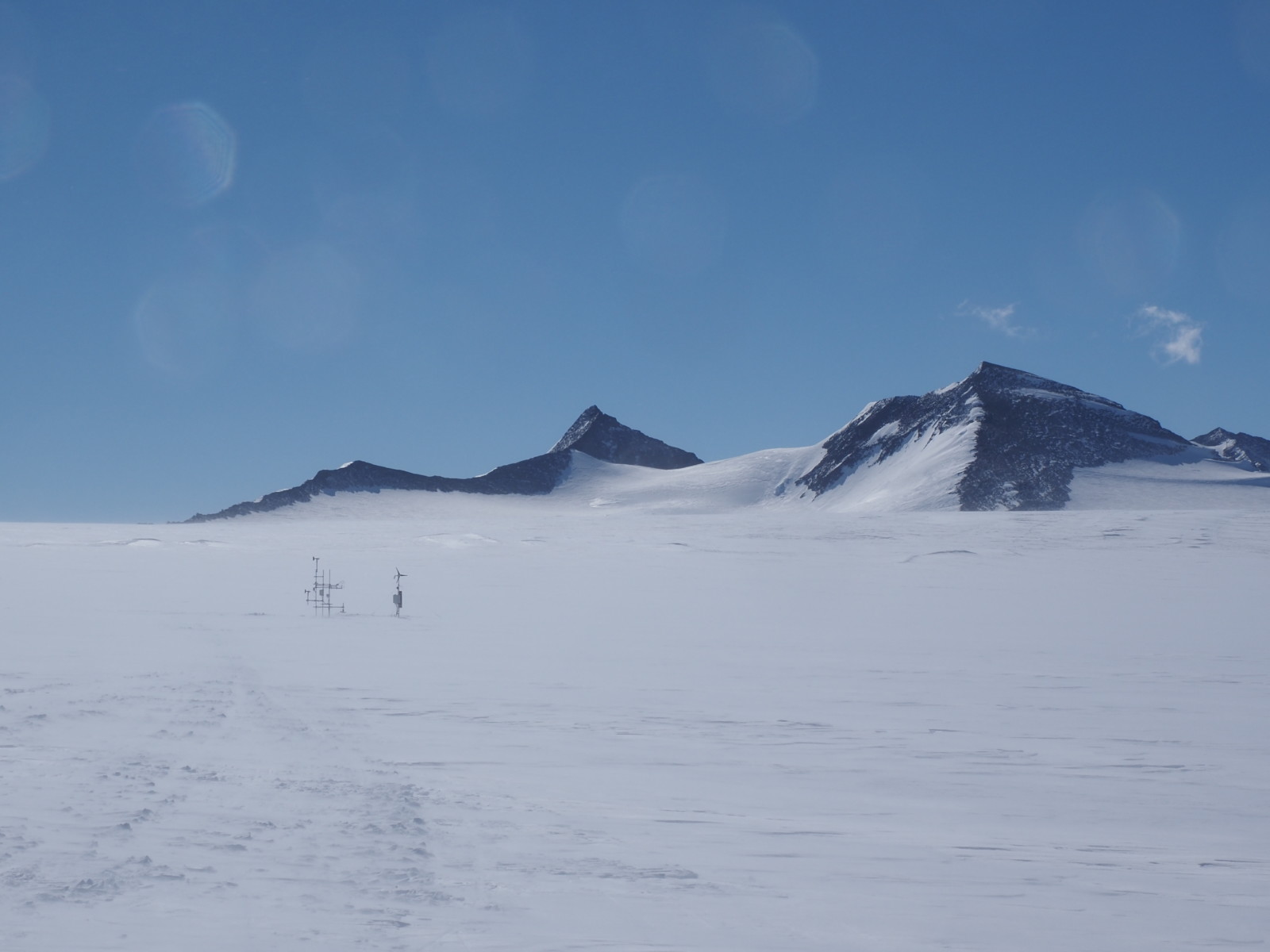
Weather station in Antarctica operated by the Laboratory of Cryospheric Sciences at EPFL, Switzerland, until December 2022. The site is located at an elevation of 2300 m a.s.l. at the edge of the Antarctic plateau, on the south side of the Sør Rondane mountains in Dronning Maud Land. Researchers had to travel by skidoo for 1:30 hrs to reach the station, wait for good visibility and follow pre-determined routes to guarantee their safety. [Credit: Martin Leitl]
Facing the challenge
Human error is not the only source of uncertainty in snow models, but it cannot be neglected. As concluded by Ménard and her co-authors, it is widespread and is slowing down scientific progress. The findings of the ESM-SnowMIP might echo in the mind of several modelers. It certainly echoed in mine. “When you are a model developer, how much time do you spend debugging (finding mistakes) other people’s code and your own code?”, asks Ménard, rhetorically, during the interview. I nodded. “I think, overall, I am saying something that effectively all modelers already know, but nobody ever says it”, says Ménard, referring to the prevalence of human errors in models.
Today, some months away from handing in my PhD thesis, I can say that we are asked to be the best scientists we can be – as long as we stay on schedule. As modelers, we are expected to build upon the findings of those who came before us. Hence, we are frequently both, users of previously implemented algorithms, and developers of new models that will improve the existing ones. It is part of our role to revise and verify the models we use. But without the support of proper documentation, we are left with a single feasible option: to trust.
The authors of the BAMS article emphasize the importance of model documentation, but after recurrently finding the same mistakes in different models, Ménard advocates for something more: “Publishing the process of how we are developing the model, of the mistakes we make, of the parameterizations (simplified models) we are trying […]. To me, it is part of the scientific process and that is never, ever, written.” The classical structure of scientific articles imposes a linear narrative from the methodology to the results and conclusions. Therefore, it leaves aside the scientific journey that precedes it. The mistakes we make are a learning opportunity, not only for us but also for others. “Somebody else will not do [the mistake], and maybe science will progress a bit faster than it does”, says Ménard.
Is there a lesson for the scientific community?
“Errare humanum est” is a well-known Latin saying. Indeed, to make mistakes is nothing but human. The findings of the ESM-SnowMIP invite us to reflect on the potential consequences of human error in science and on the strategies that we could adopt to avoid and detect them. Scientific progress often requires sophisticated measurement devices and complex methodologies. However, sometimes, openness and commitment to clarity and transparency might take us half way.
Further reading
- Menard et al., 2021: Scientific and Human Errors in a Snow Model Intercomparison. Bull. Amer. Meteor. Soc., 102, E61-E79.
- Krinner et al., 2018: ESM-SnowMIP: assessing snow models and quantifying snow-related climate feedbacks. Geosci. Model Dev., 11, 5027–5049.
Edited by Loeka Jongejans and Lina Madaj
Daniela Brito Melo is a PhD student at the Laboratory of Cryospheric Sciences at the École Polytechnique Fédérale de Lausanne (EPFL) in Switzerland. She studies drifting and blowing snow in the Alps and in the polar regions using numerical models. She is particularly concerned about the modeling of snow saltation – the motion of snow particles close to the surface – and in quantifying the importance of drifting snow in large scale climate assessments. Contact email daniela.britomelo@epfl.ch.