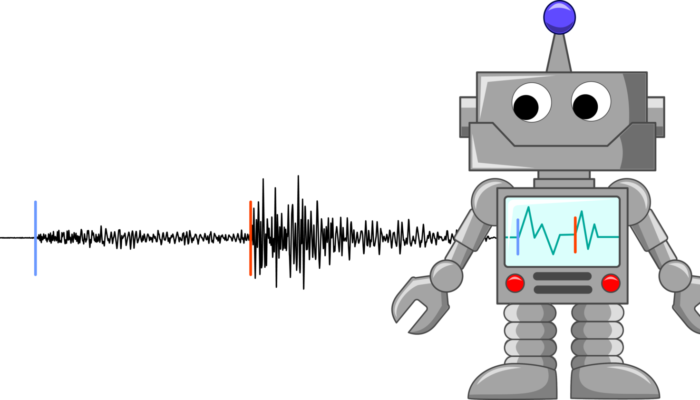
Martijn van den Ende, a Postdoctoral research fellow at Université Côte d’Azur, writes about his thoughts on the state of Machine Learning in seismology…
At this moment of writing, it is unlikely that any experienced seismologist is unaware of the recent advancements of Machine Learning (ML) methods in Earth Sciences. Some pioneering studies started paving the way for ML in the early 1990’s (Dysart & Pulli, 1990, being among the first, to my knowledge), but “mainstream” ML in seismology is still less than a decade old. Nonetheless, the potential of ML has generated much enthusiasm in the community, as evidenced by the growing body of work with either “machine learning” or “deep learning” in the title or abstract (Fig. 1).
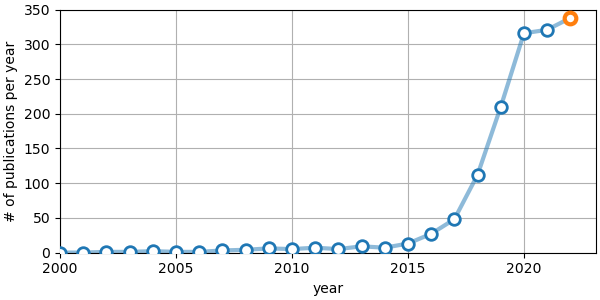
Figure 1: number of manuscripts published per year containing “machine learning” or “deep learning” (and “seismology”) in the title or abstract, as indexed by Scopus. For the year 2022, the anticipated number of publications is indicated.
The majority of these seismo-ML publications use ML methods for earthquake detection and/or seismic phase arrival picking, which is unsurprising for a number of reasons: earthquake detection and phase picking are needed for many types of analysis in earthquake seismology, but at the same time they are laborious and tedious. Having an automated approach to assist (or replace) a human analyst would free up a lot of resources and would accelerate subsequent interpretation of the results. And thanks to the tireless efforts of generations of analysts, we now have earthquake catalogues spanning many decades that one can source from when training an ML model. We as a community therefore have a strong motivation and vast datasets that facilitate the supervised training of automatic phase pickers and earthquake detectors.
Looking again at Fig. 1, it is quite noticeable that since 2020 the exponential growth in the number of published ML papers has drastically diminished. If this is not a result of measurement bias (my Scopus query was far from exhaustive) or a consequence of the global pandemic, could this be a sign of a reduced enthusiasm for ML in seismology? One could argue that with so many ML-based detectors and pickers being already published (see Münchmeyer et al., 2022, for a selected comparison), the most straightforward applications of ML that leverage our vast earthquake catalogues have already been visited. Or perhaps the diminished publication rate is an indication that it is still challenging to adopt published ML models and apply them to new data beyond the training dataset? When a given model is successfully applied to a given dataset, it is often followed by a publication; when a model fails to produce useful results, this null-result is almost never published, leading to a bias towards successes of ML. A lower publication rate could be a symptom of this phenomenon.
When a pre-trained ML model does not work well for a given dataset, often the only solution is to re-train the model on the target data. And this is where the shoe pinches: most of the published ML models are trained in a supervised manner on large volumes of annotated data; “annotated” or “labelled” here meaning that someone indicated the presence of an earthquake, the timing of the phase arrivals, etc. These annotations have been created and quality-controlled at the cost of uncountable labour hours. Creating a new dataset for the purpose of re-training a model, even when this dataset only needs to be relatively small compared to the original dataset, can be incredibly time consuming and impractical. Consider for instance a scenario of a temporary seismic array deployment for microseismicity detection, or a submarine Distributed Acoustic Sensing campaign in a seismically quiet region. In these scenarios new training data need to be generated, which is a major challenge when insufficient human analyst time is available to manually search through and annotate the data.
So what can we do to improve the adoption of ML in seismology? As mentioned before, most of the ML-based studies published in the literature rely on supervised learning with large labelled datasets. In seismology there is hardly ever a shortage of data, but these data are not always annotated (by a human analyst), which is a requirement for supervised learning methods. By contrast, self-supervised learning methods do not require labels, which is an obvious advantage over supervised methods. However, the price to pay is that formulating the learning objective in a self-supervised manner requires a lot of creativity. In the example of e.g. earthquake detection, it is rather challenging to train a model to detect events without specifying where the events are in the data. This is likely the main reason why supervised methods are currently preferred, as they are more straightforward to work with. But, if one succeeds in training such a self-supervised model, it can be retrained on new data without requiring explicit labels, facilitating adoption in future studies. Other important advantages are that self-supervised methods do not inherit bias from the analyst, and that they can be used to explore the data autonomously (and who knows what they’ll find!). Perhaps most interestingly, self-supervised methods can be applied to problems for which the “ground truth” is completely unknown a-priori, like for mantle tomography and finite fault inversion. This opens up a whole new range of applications that are not well suited for supervised methods.
Over the last decade the community has seen great demonstrations of what ML models can do to accelerate seismological workflows when labelled data are available. It is now time to consider what ML can do with the remaining, unlabelled data that we will generate in the future. The challenge of making self-supervised models work is considerable, but it also provides an opportunity to stand out, and to provide the community with easily adoptable tools for analysis.
If you want to read a much deeper take on the state of Deep Learning in Seismology, including an extensive survey of the use of Deep Learning across many subdisciplines in seismology, see Mousavi & Beroza (2022) (https://doi.org/10.1126/science.abm4470)