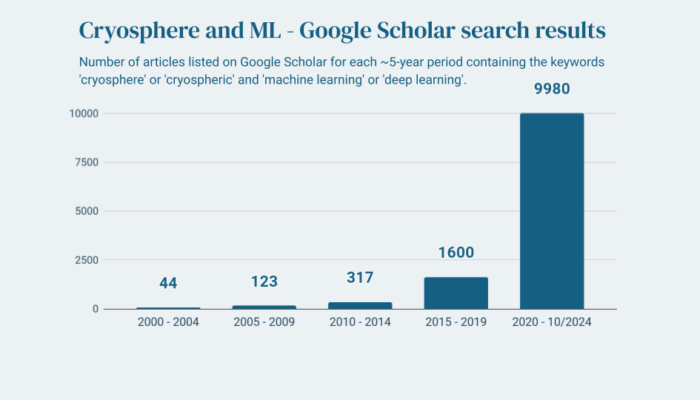
Recently, Machine Learning (ML) has emerged as a powerful tool within cryospheric sciences, offering innovative and effective solutions for observing, modelling and understanding the frozen regions of the Earth. From learning snowfall patterns and predicting avalanche dynamics to speeding up the process of modelling ice sheets, ML has transformed cryospheric sciences and bears many opportunities for future research.
Case study: Sea ice
As an example, let’s consider how ML can help us observe, model, and downscale sea ice—there are a wide range of applications in this space!
Observing: Mapping sea ice
Creating ice charts—maps of where the sea ice is located, crucial to safe maritime navigation—is a manual, tedious, and repetitive process currently carried out by highly trained ice analysts reviewing gigabytes of satellite imagery day in and day out. ML can partially automate this process, as demonstrated by submissions to the AutoIce Challenge, freeing ice analysts to spend time supporting the specific needs of ships navigating in ice.
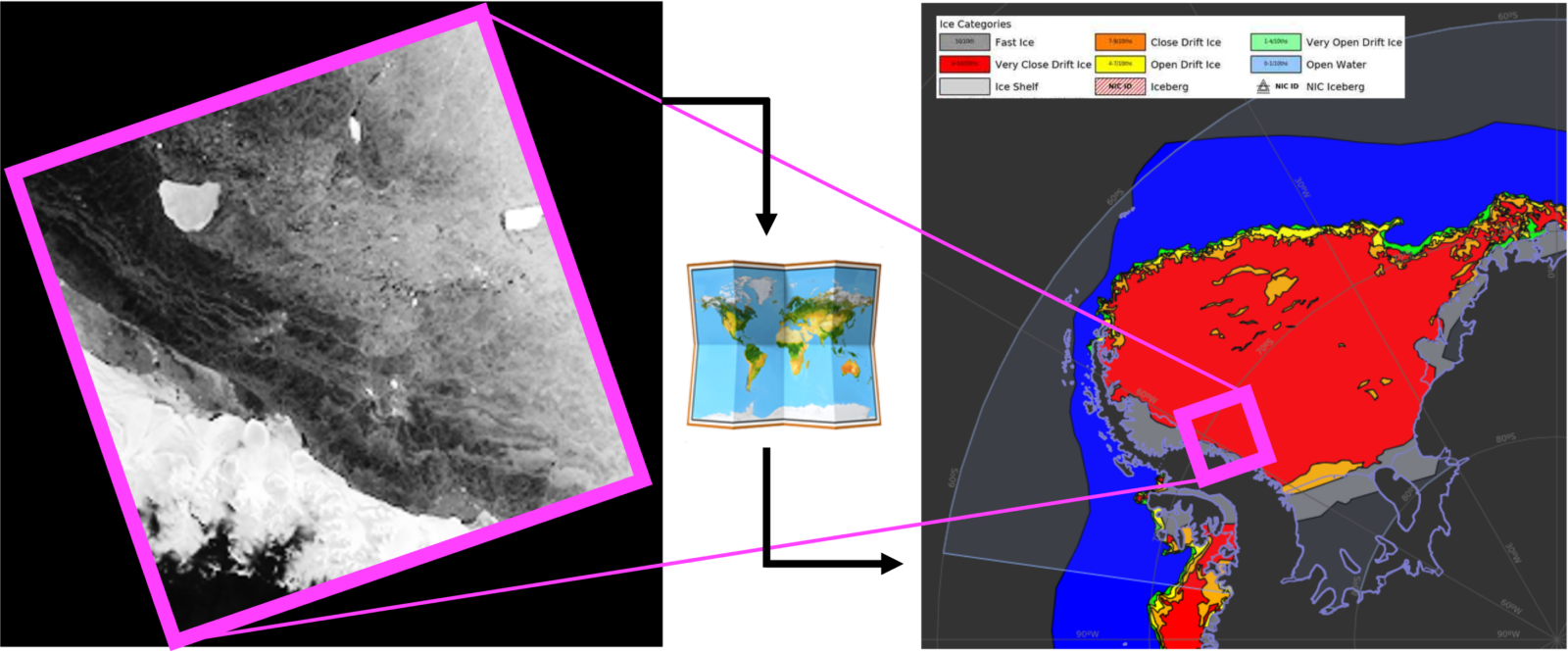
A Sentinel-1 satellite image and associated ice chart from Met Norway for 7 November 2024, retrieved from Polar View. ML could assist in the process of ice chart creation by automatically analysing satellite images, producing the product on the right from the image on the left. Courtesy of Andrew McDonald.
Modelling: Forecasting sea ice
Forecasting sea ice—crucial to our understanding of polar climate dynamics —is a computationally intensive process, conventionally accomplished by solving many complicated equations. ML offers an alternative approach here, as demonstrated by the IceNet project, enabling models to predict sea ice days, weeks, or even months ahead of time, directly from past sea ice conditions and meteorological observations, without the need for tedious model calibration or computationally expensive physics simulations..
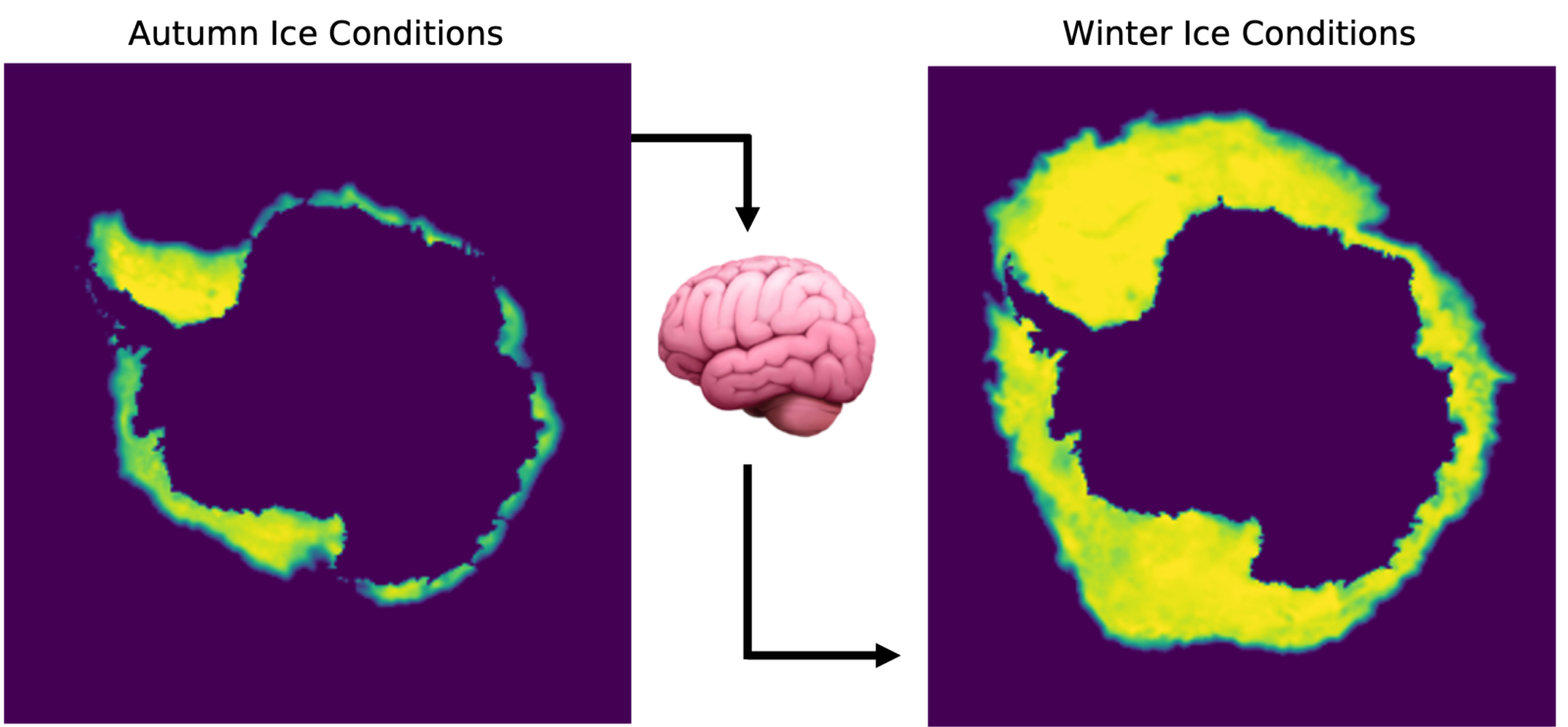
A Sentinel-1 satellite image and associated ice chart from Met Norway for 7 November 2024, retrieved from Polar View. ML could assist in the process of ice chart creation by automatically analysing satellite images, producing the product on the right from the image on the left. Courtesy of Andrew McDonald.
Downscaling: Improving resolution of sea ice maps
High-resolution maps of sea ice are important for scientists’ understanding of sea ice but are not always available, especially as one looks earlier in the historical record. Given more readily available low-resolution maps of sea ice, one can train a ML model to produce a higher resolution map, helping scientists understand smaller-scale processes in sea ice formation and change!
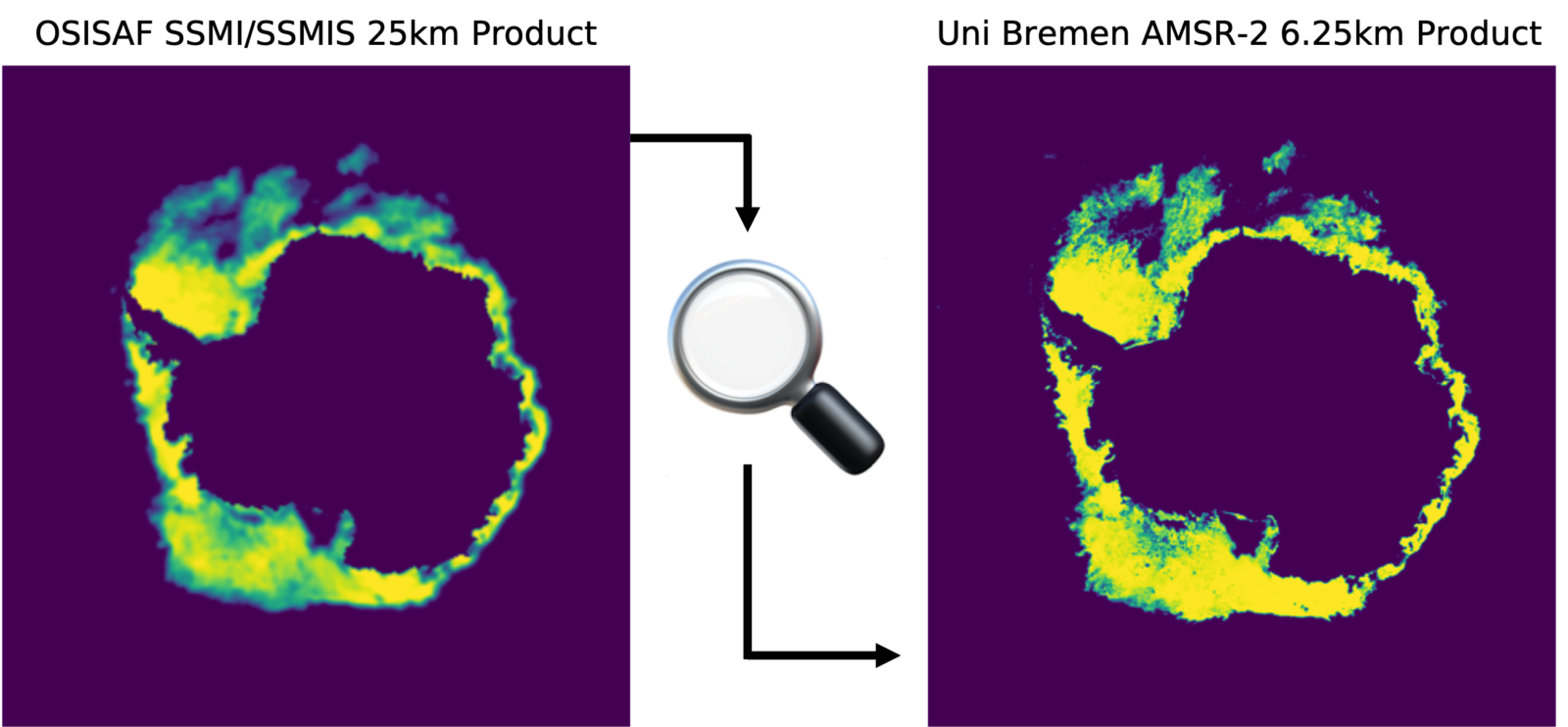
A low-resolution (left) and high-resolution (right) sea ice concentration product from 15 December 2019. ML could be used to downscale (improve the resolution of) the product on the left to resolve the small scale features as seen in the product on the right. Courtesy of Andrew McDonald.
The applications here—observation, modelling, and downscaling—are not unique to sea ice, but rather share several common threads with applications in glaciology, snow and avalanche research, ice sheet dynamics, and beyond.
ML4Cryo: Building a community
As PhD students applying ML within the cryospheric sciences, we have found a considerable gap between the ML and cryosphere research communities. There is insight lost in translation; ML researchers may lack awareness of the most pressing challenges within the cryospheric sciences, while researchers studying the cryosphere may lack awareness of the most recent state-of-the-art models being developed by the ML community. It is incredibly difficult to be a scientific expert in a single field, let alone two! True interdisciplinarity in the 21st century requires collaboration and cooperation between and across research communities.
Towards this end, we have created ML4Cryo, an online community for both cryospheric scientists interested in ML and for ML scientists interested in applications to Earth’s cryosphere. Inspired by the success of Climate Change AI, we envision the community to be a member-driven space in which ideas, collaborations, best practices, and opportunities are shared freely and enthusiastically. More broadly, we hope the community can work together to identify key challenges and key scientific questions across cryospheric subfields, and analyse if and in which form ML has aided or could aid in addressing those. As we prepare for the 2025-2034 Decade of Action for Crysopheric Sciences, the 2025 International Year of Glaciers’ Preservation, and approach the 2032-2033 International Polar Year, we hope ML4Cryo can bridge the aforementioned gap between the ML and cryospheric research communities, forging a path towards meaningful and impactful application of ML to advance our understanding of the cryosphere.
ML4Cryo’s current activity is concentrated within a Slack space which we encourage you to join; in the future, we plan to set up a mailing list and convene in-person meetups, such as networking events at major conferences and skill development workshops.
On that note, we invite you to submit an abstract to our session at EGU25!
Edited by Emma Pearce and Maria Scheel