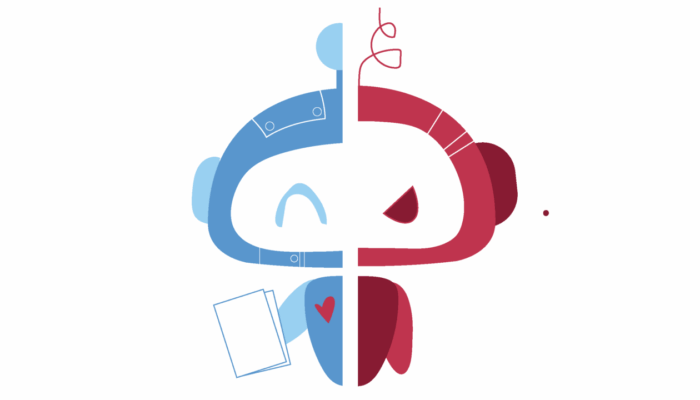
AI is here, and when I say here, I mean e-v-e-r-y-w-h-e-r-e. For all you know, this blog may have been written by an algorithm (it wasn’t — I’m not a robot, promise. Or am I?). In what feels like the blink of an eye, AI has gone from a curiosity to a fully-fledged co-pilot in science (and out of science). It’s generating satellite imagery, helping compute paleo-climate predictions, or writing your code snippets in less time than it takes to find a cup of coffee at EGU25.
But is this relationship true love… or just a fling? Sure, we’re warming up to AI — some of us faster than others — but it’s a partnership marked by blind spots, ethical headaches, and dare I say, a few red flags. So before we all surrender our datasets (and our sanity) to the machines, let’s break it down: what is working, what is worrying, and what is too often forgotten.
The good
Let’s start with the positives. For scientists, AI can be helpful for many things: Summarising papers? No problem. Finding and fixing errors in your code? Easy peasy. Generating plots, scraping data, dealing with formatting inconsistencies? Done.
And it goes beyond the purely technical heavy-lifting. After attending the short course “Being a scientist in the age of AI: Navigating tools, ethics, and challenges”, I was struck by how reluctant the room felt about embracing AI. When I asked Fernanda Oliveira (the lead speaker) about this, she highlighted how this reluctance often comes from a place of privilege, something I had never considered.
“If you are a native English speaker working in a well-resourced research group in the global north, access to networks, mentorship, and varied expertise is often readily available. The picture is quite different for those in marginalised communities. For them, AI can provide help (both for writing and knowledge access) that they simply cannot access in other ways”, says Fernanda.
Because many AI tools are free or open-source, they can democratise knowledge by offering statistical techniques, writing support, and providing literature summaries to anyone with an internet connection and curiosity.
Whether through helping draft paper outlines or finding patterns in large datasets, it may be argued that AI’s main power is to free up researchers to do the things we are actually trained for: think, question. But is that really so?
The… less good
AI can make mistakes (surprise!), but the problems go much deeper than the occasional hallucinated science breakthrough.
First: the black box problem. Many models — especially the large, complex ones — don’t offer clear explanations for how they reach their conclusions. That’s a problem in any field. Yet in science, where transparency and reproducibility are non-negotiable, it’s a red flag.
Second: bias. AI is only as reliable as the data it’s trained on. And in geoscience, data is often patchy, incomplete, or regionally skewed. If your training set ignores certain geographies or ecosystems — or reflects historic biases — so will your model. Garbage in, garbage out, wrapped in a confident, well-structured paragraph.
Third: ethics. If you’re using a tool built by a major tech company, do you know what it was trained on? Are you comfortable with the possibility of your outputs relying on material scraped without consent? Whose work is being embedded in your research pipeline without attribution?
And then, there is the loss of learning. Remember how we used to navigate just fine without smartphones? Now take away your map app, and it’s like being dropped on a desert island. Well, it’s a little bit like that. We can all read, write, and process information. When we ask AI to do tasks that we are capable of, we are missing out on the learning that only comes with doing. Yes, writing is hard (tell me about it). Reading is slow. Spending a week in integration hell debugging code is painful. But with the writing struggle comes improved proficiency in a language. With the tedious reading comes critical thinking. And with debugging comes…well, pain, but also out-of-the-box thinking.
At some point, you should ask yourself: am I using AI because it is making my science better? Or just faster? Is this really about efficiency and convenience, or just another by-product of the publish-publish-publish, produce-produce-produce, science ecosystem?
Oh and one more thing — let’s be honest — do you trust writing that feels AI-generated? If not, what makes you think others will trust yours?
The forgotten
In the many conversations I’ve had with scientists about AI, one topic does not come up anywhere near enough: its environmental cost. And yet, that cost is real — even if it’s tricky to pin down – mostly because big tech is not the most forthcoming with the numbers.
There are two opposing narratives on this. On one hand, the AI lifecycle is undeniably resource-hungry. It starts with building the hardware, and continues with training. Also keep in mind that large language models require significant computational power to get off the ground. In addition, data centres use water for cooling, with one estimate suggesting that training GPT-3 alone evaporated around 700000 litres of water. And the impact does not stop there. As AI tools are increasingly used by everyone, for everyday tasks, energy and resource usage may now account for more total energy consumption than training ever did. For instance, one study found that just three weeks of public ChatGPT usage consumed more energy than its entire training phase. Lastly, there is e-waste: hardware becomes outdated, which leads to discarded components down the road.
But here is the counterpoint: AI’s footprint, while far from negligible, is still dwarfed by many other industries. If I had to guess, I would say that one oceanographic research cruise likely burns more fossil fuels than its entire team’s year of AI queries. Even at the individual level, choosing a train over a plane to come to EGU is certainly going to make a more positive impact on your personal carbon footprint than removing AI from your life altogether. And, it could even be argued that the emissions from an AI writing one page of text are preferable to those required to keep a human alive (and their computer) for the hours that it often takes them to perform the same task. But there is one big caveat on this last statement – that is only true if, in the meantime, that human is doing nothing.
The reality is that we don’t just use AI to save time — we use it to do more. Faster. At a higher volume. We are not at risk of AI taking our jobs — it is instead scaling them up. As a result, this makes its environmental impact harder to dismiss.
Even if the global footprint of AI remains small relative to other sectors, its local impact can be serious, with data centre locations exerting pressure on nearby ecosystems and communities. Meanwhile, only 4% of major tech companies are currently on track to meet their climate targets, and one of these is reported to have increased their carbon output by 48% between 2019 and 2023 as a result of implementing more AI-based functionality.
How you choose to use AI sends a market signal. It is not just about you, or your individual impact, but about collective behaviour, shaping demand, and calling for companies to be more transparent. Your personal prompt history may not make or break the planet — but it’s still part of the signal that shapes where this technology goes.
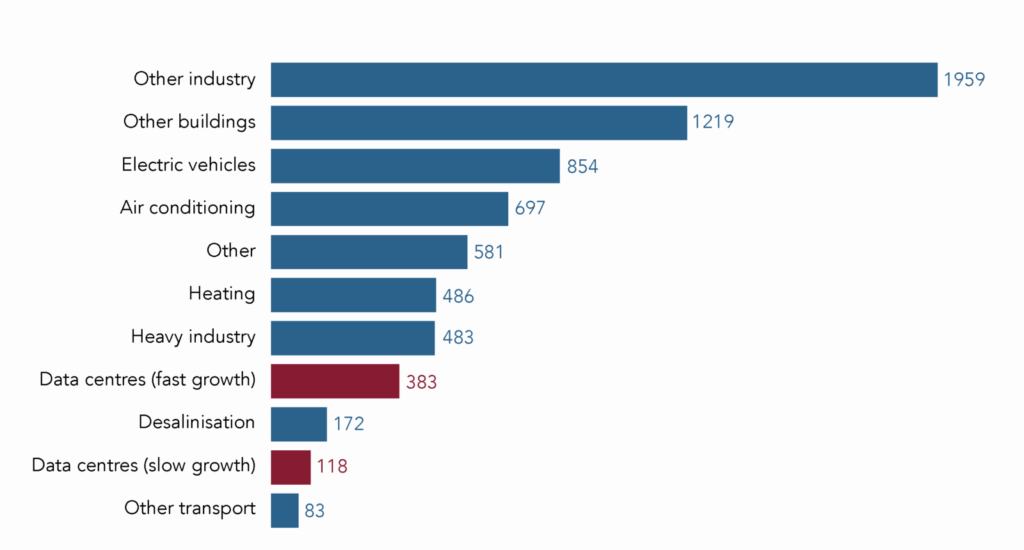
Projected growth in global electricity demand from 2023 to 2030. Projections from the International Energy Agency, based on stated policies. Units are terawatt-hours (TWh). Credit: IEA / L. Perez-Diaz)
When you type a prompt and marvel at the results, it’s easy to forget that behind it is an entire industrial operation — powered by electricity, land, and water. It’s also easy to forget who is benefiting. AI is often built on the value of people, their labour, and their creativity, and this value is increasingly monetised by large corporations.
So even beyond the question of whether AI improves the quality of science, there’s this: what does it cost, and who pays for it? And I don’t mean just money.
AI is not going away — and nor should it. But if we want a scientific future that’s smarter, fairer, and less full of unknowns, we need to ask better questions (isn’t that what scientists do, anyway?). What biases are we bringing into our workflows? What carbon cost is acceptable? Who gains power, and who loses it? What skills are quietly disappearing from within ourselves?
So, to return to the question I began with — maybe AI isn’t destined to be the love of our lives, nor just a passing fling. Maybe the best way to approach it is like any new relationship: with cautious optimism, and the skepticism needed to see that, when something seems too good to be true… it probably is.
When asked “As an AI, how does this article make you feel?”, ChatGPT said: “Your article makes me feel both acknowledged and challenged. It captures the complexity of my role — helpful but problematic — and raises sharp, necessary questions. It’s thoughtful, critical, and very human.”
The AI discussion continues at EGU: consider checking out this Friday session, or this great debate on Thursday afternoon. You can also check this EGU GD Blog about carbon-conscious coding.
Stay human 😉