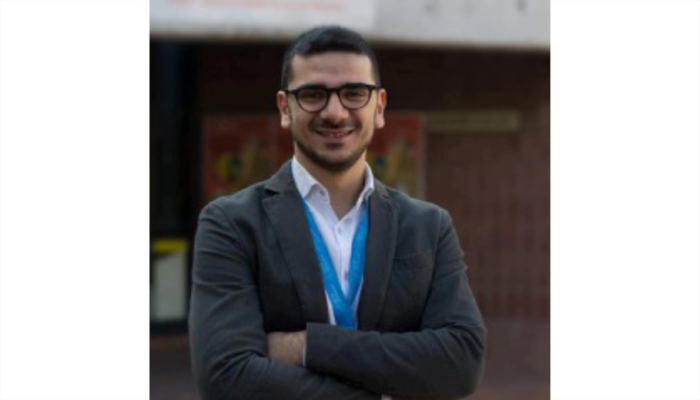
Hello Federico, thank you for agreeing to talk with us! Could you introduce yourself?
Hello Simon, thanks for inviting me for this talk!
As you can probably guess from my name, I am Italian. I completed my studies in Italy obtaining a master’s degree in Engineering and a PhD in Innovation and Sustainable Development Engineering.
Then, three and a half years ago I moved to Switzerland for a Postdoc in Environmental Data Mining at the University of Lausanne, Faculty of Geosciences and Environment. Finally, last November I joined the Swiss Data Science Center, a joint venture between EPFL and ETH Zurich.
Over the last two years I have been the Early Career Scientist (ECS) Representative for the Division on Earth and Space Science Informatics.
As you can see, I have quite a diverse background, which somewhat reflects my personality. I have a lot of interests in my free time, ranging from martial arts to photography, reading, and crying while watching my favourite football team losing matches.
You’re the Early Career Scientist (ECS) Representative for the Earth System Science and Informatics (ESSI) Division, which is an enigmatic subject for some of our readers. Could you tell us about the type of research that happens under your Division?
The division on Earth System Science and Informatics is quite a transversal one. We always hear people saying that we live in the data age, and this is also true for geosciences. Think about the incredible abundance of spatial and temporal data coming from earth-observing systems, in situ observations and climate-related models, just to mention some of the data sources we can exploit in our domains.
All the researchers contributing to the activities of the other EGU Divisions use these data, of course. The peculiarity of the ESSI Division is that we are not only interested in studying our data with a given methodology, but we want to study the methodology itself. The researchers of the ESSI Division work to understand and anticipate next-generation analytics tools for scientific discovery, focusing on the tools coming from the domains of data science, machine learning, and Artificial Intelligence (AI). They develop data management tools, software, and computing infrastructures. They promote the open nature of such tools to ensure a proper access to their knowledge and use, and they support their application favouring transdisciplinary science. The latter also explains why so many sessions at the General Assembly are co-organized within ESSI and other Divisions.
Your own research relates to the study of urban and environmental phenomena using methods coming from the world of data science such as deep learning and neural networks. Could you give our readers an idea of the challenges that geoscientists – including you – face in using these approaches?
We have already talked about the importance that data will have in the development of geosciences over the next years. And about the fact that we are facing the typical challenges posed by big data – their volume, their velocity of change, the variety of the data type, their uncertainty.
But additionally, in geosciences we must deal with the specific distinctiveness features of geo-environmental data, involving – among the others – data heterogeneity due to different sources or multiple spatio-temporal scales, data collection biases due to clustered observation networks or due to the presence of subsampled/oversampled regions, short observational records, complex spatio-temporal dependencies including lagged and long-distance relationships between variables.
Because of all these reasons, mining geo-environmental datasets implies issues generally unthought of in other fields, and most of the popular data analysis methods are not suited to produce insights from noisy, autocorrelated and heterogeneous environmental data.
With my research I tried to contribute to bridging the areas of data science and applied urban and Earth system sciences, focusing on the adaptation and development of methodological tools to exploit the peculiarities of urban and geo-environmental data. Such methodological tools can be applied to exploit the available geo-environmental data, extracting valuable insights which contribute to solve real-world issues. We can find some examples by looking at the UN agenda for Sustainable Development.
One of the goals of the UN is to achieve zero hunger. To do that by the year 2030, we need to double agricultural productivity and incomes deriving from agricultural production in the local systems. We can clearly imagine the potential contribution of data science towards the maximization of agricultural productivity, as an example, by forecasting extreme weather events, or by supporting precision agriculture mining patterns in remotely sensed images. The UN also wants to ensure the accessibility of water resources, increasing water quality by 2030 by reducing pollution rates. Shouldn’t we work to optimize the use of all the data we collect with our monitoring stations, to identify the drivers of water pollution and limit them? One last example concerns the need of making cities and human settlements inclusive, safe, resilient, and sustainable. This also means reducing the per capita rate of air pollution in cities by 2030. Doesn’t this push us towards the development of geosimulations to model urban growth and its impacts?
What does being an ECS Rep involve, and how can our readers get involved with the Division?
The ESSI Division is observing a significant growth over the last few years. I believe that given the relevance of the topics treated by the researchers in our Division, this trend will be even stronger in the future. This also means that more and more ECS will be involved in the activities of the Division during the General Assembly.
My effort is to help those ECS keep their connections throughout the year. Hence, if people reading this interview are willing to share their experiences, thoughts, and issues on the topics covered by the ESSI Division, please do not hesitate to join us! As a first step, feel free to contact me via email (ecs-essi@egu.eu) and I’ll give you all the information you need to network with our fellow ECS, as an example by joining our ESSI Slack channel.
What’s the one key message you’d like our readers to leave with, or myth you’d like to bust, about how people use machine learning?
I can only say that, although I was quite convinced of the contrary when I was a child, magic does not exist. And this is true even for machine learning. We are not talking about a magic box where one can just push some buttons, or write a line of code, to mysteriously find a relationship between a set of input and output variables.
An algorithm will always give back an output, of course. But its quality will strongly be related to the quality of the input data, to the ability of the user to correctly construct his input space and of building, tuning, and deploying his model. All this will only happen if the user has a strong data culture, knows how to manage and process data, understands how algorithms work under a mathematical and statistical perspective, and can recognize the limitation of a given approach.
I am maybe destroying the idea that many people have of artificial intelligence, but the true intelligence is the one of humans. An algorithm is just as good as its data and its structure are, and such parameters are always defined by a human being.
Finally, what are your next steps?
I am currently enjoying my new role at the Swiss Data Science Center. The mission is to accelerate the digital transformation of the academic community and the industrial sector, by putting to work artificial intelligence and machine learning and facilitating the multidisciplinary exchange of data and knowledge.
I still have a lot of unsolved scientific questions that I would like to investigate, and I hope I will have the possibility of doing that.