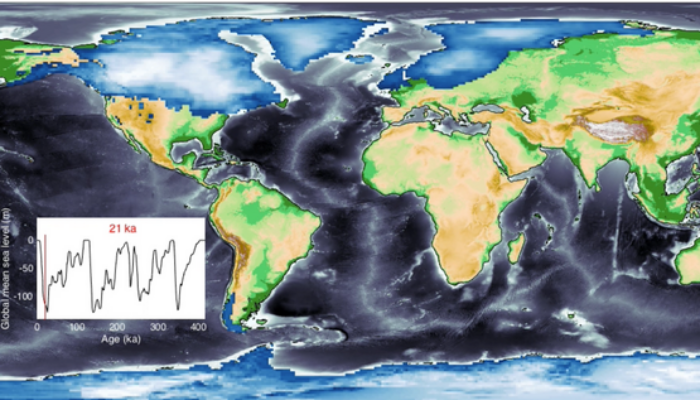
Sea-level change serves as a direct indicator of climate change with profound implications for coastal areas. Since 1900, the global mean sea level (GMSL) has risen over 20 cm, leading to beach erosion, delta inundation, and increased flooding worldwide. Over glacial cycles spanning tens of thousands of years, interactions between ocean and continental-scale ice sheets can cause GMSL to fluctuate by more than 100 meters, radically reshaping our familiar landscapes. For instance, during the Last Glacial Maximum (LGM) around 21,000 years ago, GMSL was approximately 130 meters lower due to extensive ice sheets in North America and Northern Europe, transforming shallow continental shelves into dry land (see Figure 1). To unravel the intricacies of how changing sea levels reshape past landscapes and coastlines, it is essential to comprehend the global impact of ice sheets on sea levels.
When ice sheets melt, the redistribution of freshwater between ice sheets and the ocean can significantly change Earth’s gravitational and rotational field and cause solid Earth deformation. Such gravitational, rotational, and deformational effects can interact to produce complex spatially variable sea-level change patterns. A widely used numerical method to describe these three effects is called the glacial isostatic adjustment (GIA) model, which can predict global sea-level changes and reconstruct past landscapes based on known ice sheets. If you want to know more about GIA, please check this blog post.
One difficulty for GIA modelling is that, due to the erosive nature of ice sheets, our knowledge of their history since the LGM is limited. To overcome this challenge, researchers employ the GIA model to project sea-level changes for various ice history scenarios. This allows them to identify the best-fit ice model by comparing the projections with global sea-level observations. But as you can imagine, there are so many plausible ice histories (easily go up to tens of millions) that computing relative sea-level for them is often prohibited by the computational resources required (e.g., large supercomputers) to repeatedly solve the complex physical equations.
A solution to this problem is using some machine learning algorithms to build a statistical emulator (or surrogate model) to mimic the behaviour of a physics-based simulator (e.g., GIA model), which is computationally cheap to run. Rather than solving the physical equations exactly, these “statistical emulators” learn how the system behaves based on a (comparatively) small set of examples and use this to predict what the simulation would output in other scenarios (Figure 2). Thanks to the modern machine learning framework (e.g., PyTorch), such statistical emulators can be run by users with limited or even no programming experience who can also use these tools.
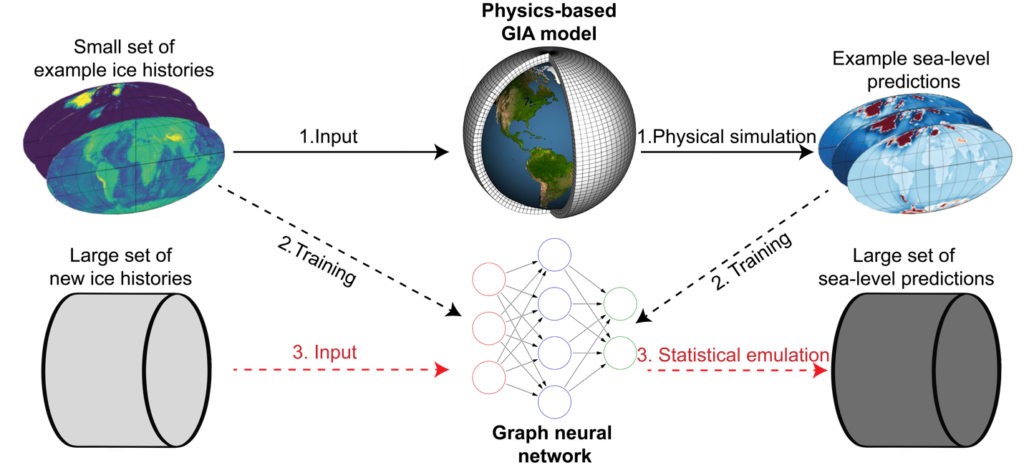
Figure 2. A simplified framework to build GEORGIA. There are three major steps (denoted by numbers and different types of lines): preparing training examples by physical model, training statistical emulator, and statistical emulation.
Using a machine learning algorithm, we built a GIA emulator (called GEORGIA) that can predict sea-level change across the globe (from 27 ka BP to present) within just a few seconds (c.f., 10-20 minutes for a physics-based model), which incorporates three physical processes described above.
By comparing GEORGIA and a physics-based GIA model sea-level predictions on 150 new ice histories (i.e., not being used to train GEORGIA), we find the average difference between them is 0.54 m. This is quite satisfactory because the magnitude of sea-level change is often tens of or hundreds of meters. In other words, GEORGIA performs well enough for some interesting applications! For example, how do different ice histories impact sea-level change in different regions, how do paleo shorelines evolve, and how does it affect ancient human/animal migration? All of these can be informed by GEORGIA. You can find example shoreline migration snapshots in Figure 3, generated using one random choice of ice history. Based on this ice model, we can see a huge plain area across southern Asia back in 20 ka BP, which was gradually inundated by rising sea levels between 17 and 11 ka BP. To explore more about ice history’s impact on shoreline migration, you can try this GEORGIA-based API. No programming skill is required! See a demo here.
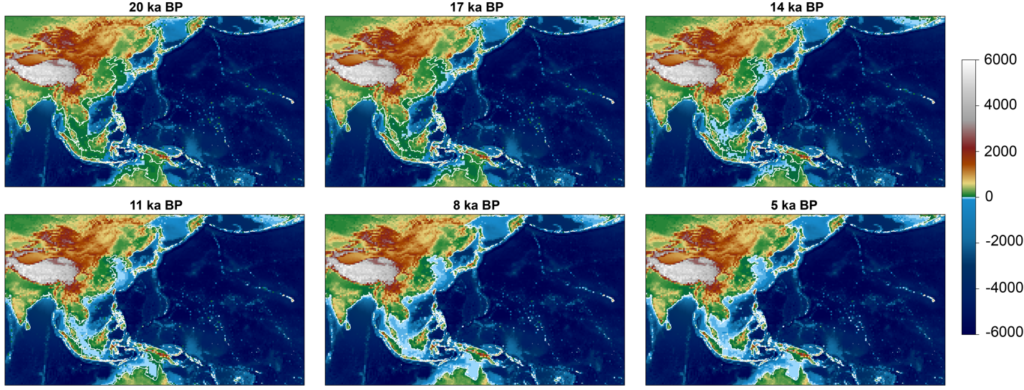
Figure 3. Example coastline evolution maps generated by GEORGIA. Background colors indicate paleo topography reconstruction, and white lines indicate modern coast. ka BP = thousand years before present.
Like other emulators, GEORGIA also has some limitations. For example, it cannot meaningfully predict ice histories that are significantly different relative to the 1,200 examples used to train it. Apart from that, the spatial resolution of GEORGIA’s prediction is quite low (~3.6 degrees), which was chosen to allow a normal laptop to run GEORGIA locally. Lastly, GEORGIA assumes a single Earth rheological model, which controls the magnitude of solid Earth deformation in response to ice sheet melt, a factor that can also have a huge impact on sea-level change. Meanwhile, GEORGIA is fully open-sourced, so users can easily modify it to suit their own needs.
In summary, we built GEORGIA, a new open-sourced statistical emulator for GIA that can predict postglacial sea level with minimum requirements for computational resources and programming skills. Therefore, anyone can use it to investigate past ice sheets, shorelines, and climate change, even just using a laptop!
References
Lin, Y., Whitehouse, P. L., Valentine, A. P., & Woodroffe, S. A. (2023). GEORGIA: A graph neural network based EmulatOR for glacial isostatic adjustment. Geophysical Research Letters, 50(18), e2023GL103672.