Ice sheet models are awesome tools that help us learn and predict the fate of ice sheets under human-induced climate change. However, all models have errors. What types of uncertainties exist in an ice sheet model and how can we quantify some of them efficiently? Check out our recipe to quantify one type of uncertainty in sea level rise projections: The model calibration error. Not a numerical mod ...[Read More]
Recipe to quantify calibration errors in a time-dependent ice sheet model
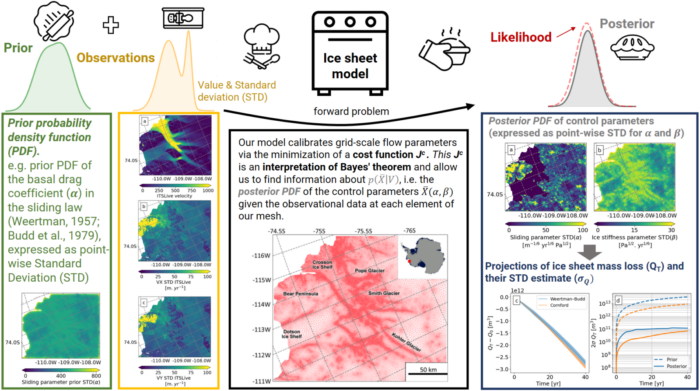
Figure 1: Upper diagram: schematics of baking a pie, representing an idealized 1D Bayesian inverse problem. Lower diagram: schematics of a multi-dimension ice sheet inverse problem solved using FEniCS_ice error propagation framework, created from a compilation of figures from Recinos et al. (2023). FEniCS_ice is designed to solve these problems over a finite element mesh containing (in this study case) more than 100,000 elements. These elements are the vertices, faces, and edges that form our triangular mesh (red map, check out ingredients list below for more information). [Credits: Beatriz Recinos, baking icons from https://www.flaticon.com/].