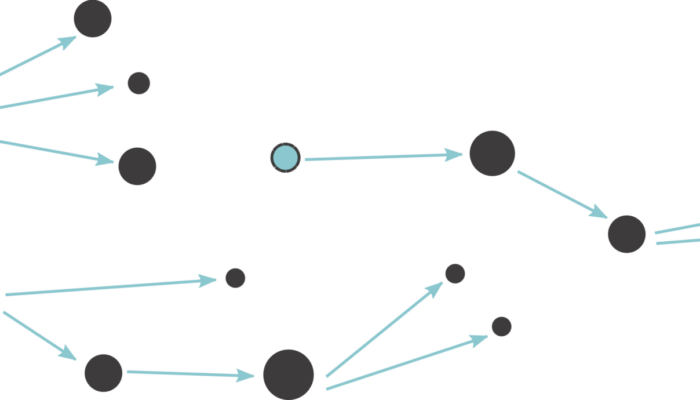
This blog post will take you through what can and can’t be done with statistical earthquake forecasting. We are happy to have it explained by Dr. Leila Mizrahi, who is a postdoctoral researcher at the Swiss Seismological Service (SED) at ETH Zürich. Let’s get started!
“My research topic is earthquake forecasting” – “Oh, really, I thought that’s not possible! So, when is the next one going to happen?”
This is how a conversation about how I spend my workdays usually starts. Quick disclaimer: If you came here to know when the next devastating quake will hit, I don’t know, and this is not what this blog post is about. If, however, you came here to learn what it means to do “earthquake forecasting”, stay with me.
Among statistical seismologists, we like to distinguish the terms “prediction” and “forecast”. A prediction would be what most people have in mind when they ask about the next one. A specification of the exact time, location, and magnitude of the next noteworthy earthquake. A forecast is a little less captivating, at least the first time you hear about it. A probabilistic assessment of the possibility that an earthquake occurs within a specified space-time-magnitude domain. “The probability that an earthquake of magnitude 4 or larger occurs in the next half hour in a 20-kilometer radius around my office is 0.0003%”. What to do with this information? I’m probably not going to leave the office, even if this chance temporarily increases by a factor of one hundred to 0.03%. But someone operating a nuclear power plant will surely want to know about such a hundred-fold increased probability.
So, how do we do it? How do we calculate these probabilities?
Basically, we look at earthquake records from the past, try to find patterns, and use those patterns to say something about future earthquakes. The most widely used model for earthquake forecasting is the so called “Epidemic-Type Aftershock Sequence” model (short: ETAS model; Ogata, 1988). This model partitions earthquakes into background earthquakes and triggered earthquakes.
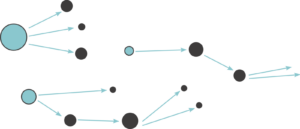
Figure 2: Schematic illustration of background (turquoise) and triggered (black) earthquakes. Source: Mizrahi (2022)
All earthquakes that are not triggered by previous ones are considered “background earthquakes”. Triggered earthquakes, on the other hand, are what we commonly refer to as “aftershocks”. But be careful: These aftershocks can sometimes be larger than the quakes that triggered them, and they can trigger aftershocks of their own. The triggering follows a few empirical laws. These describe how many aftershocks an earthquake will have, given its magnitude, how soon after the “parent event” they will occur and how far away from it, and how large they will be.
Now, what does someone who works on earthquake forecasting do?
Of course, anyone would love to come up with a totally new model that revolutionizes everything and that is just much better than all existing models. How hard could it be? Turns out, it is hard. In a recent elicitation of worldwide earthquake forecasting experts (Mizrahi et al. 2023), a large majority of experts indicated that if they had to choose one single model to issue authoritative earthquake forecasts, they would go for an ETAS model, which, notably, was first described over 30 years ago.
You might ask yourself: “What have statistical seismologists been doing for 30 years then?” Let me illustrate the many different challenges in the endeavor of developing an automated earthquake forecasting system for Switzerland, a task I have been quite involved with lately.
Developing a model
Although the theory behind the ETAS model is relatively simple and well-defined, calibrating the model for Switzerland is not so straightforward. Luckily, the Swiss Seismological Service operates a very dense seismic network which can reliably record earthquakes down to magnitude 1.0 or so. To calibrate a model, we need an earthquake catalog (basically a list of past earthquakes with location, time, and magnitude) that is complete. But how do we know if the catalog is complete? We don’t know about the earthquakes that were not recorded (because they were not recorded). So, we must make assumptions and use models to make a best guess for how complete a catalog is. Even if we knew, this completeness changes over time. We didn’t always have the dense seismic network we now have. So then, the question is: do we prefer an earthquake catalog of a longer time horizon that is less complete, or one from a more recent time horizon that is more complete?
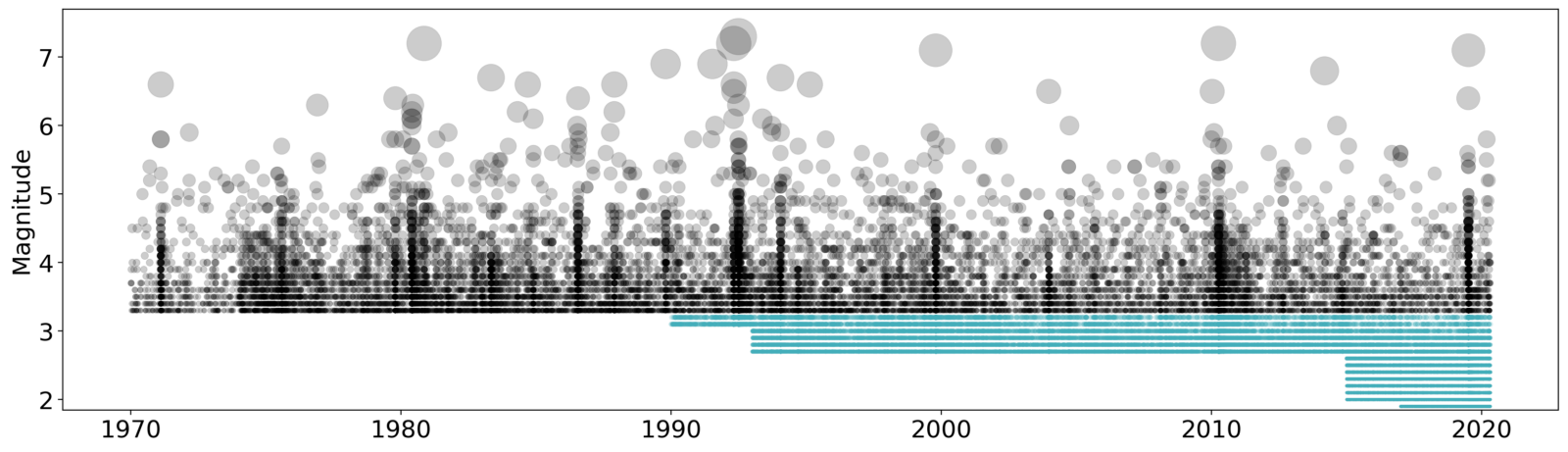
Figure 3: Illustration of an earthquake catalog with improving completeness. Over time, the network can detect smaller and smaller events. Starting around 1990, the turquoise dots show how smaller and smaller earthquakes can be detected over time. This is not the Swiss catalog but the Californian one, with incomplete parts removed. Image credit: Leila Mizrahi
Testing the model
We can try both and compare the models. And while we’re at it, why don’t we also compare 6 other model variants? One that was calibrated on worldwide data, one calibrated for California, one that uses some extra information from the Swiss long-term seismic hazard model, and a few more that combine several of these modifications.
But how do we compare them? The gold standard of forecast testing would be to do “prospective” testing; to test the models on data that did not exist when the models were developed. But with earthquakes, especially in Switzerland, we would potentially have to wait a long time until enough data is gathered to make such tests meaningful.

Figure 4: In pseudo-prospective testing, one pretends not to know a part of the data when developing a model. This data is then used to evaluate the performance of the forecasting models. Source: Mizrahi (2022)
As an alternative, one can do “pseudo-prospective” testing. We exclude the last few years of data when calibrating the different model variants and use those for testing. This is possible, but has its downsides, too. Reserving the testing data makes the dataset that is usable for training smaller than it already is. Where is the right balance between significant test results and enough training data to obtain a good model? There is no definitive answer to this. And who said that if a model does well at forecasting magnitude M≥2.5 earthquakes (we have many of those in the test dataset), it will do well at forecasting M≥5.0 events (none of those are in the test dataset)? This question alone opens an extensive body of research on the scale invariance of seismicity.
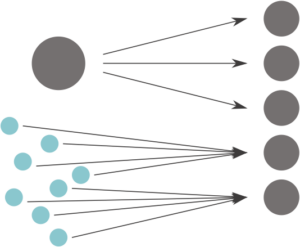
Figure 5: Do many small earthquakes provide the same kind of information that few large ones do? Image credit: Leila Mizrahi
The other thing we can try is “retrospective” testing: We use test data from the past that was possibly used to calibrate the models and see how well the models describe this past data. This has the advantage that we can go back as far as we want and use the large earthquakes for testing. The obvious disadvantage is that we are kind of cheating if the models were built using the data used to test them.
At the Swiss Seismological Service, we are performing a mixture of retrospective and pseudo-prospective tests to select the preferred model, and plan to store the regularly calculated forecasts so that truly prospective tests can be carried out later. Only very few governmental agencies worldwide already produce automated time-dependent earthquake forecasts, and the “best practices” of how to develop and test the models behind those forecasts have yet to be established. Time-independent (long-term) forecasts of earthquake occurrence and the expected levels of ground shaking inferred from them are much more established and central parts of national or international seismic hazard analysis (see for example the new European Seismic Hazard Map; Danciu et al., 2021). The most important measure for saving lives in earthquakes remains to build buildings which can withstand the shaking expected at their location.
Communicating a forecast
Suppose all the choices regarding the development and testing of earthquake forecasting models are made. Suppose we have selected a model. We understand its strengths and weaknesses, know what it can and cannot do. What do we do with it? Do we share forecasts with the public, with the risk that probabilistic forecasts are misunderstood by laypeople who aren’t used to interpreting probabilities? When should a forecast be released, how often updated? Should we provide maps of earthquake probabilities, and if so, which color should be used to symbolize a 1% chance of an earthquake? Even with an explanatory color bar next to it, a red area on the map will seem more threatening than a green one. All these are open questions being investigated by social scientists and earthquake forecasting specialists. In the expert elicitation mentioned earlier (Mizrahi et al., 2023), one main result was the agreement of most experts that communication products for earthquake forecasts should be developed in close collaboration with the end-users.
Figure 6: Two maps showing the same scenario forecast for Switzerland, with different colormaps. No information about the time and magnitude range of the forecast are provided, this is intentional. Did you think 1% is high? Or low? How can it be high or low if you don’t even know if it’s the probability in 5 minutes or 5 years? Source: adapted from Böse et al. (2023, submitted)
Finally
A lot of questions were raised in this blog post, and only a few of them were answered. Some of them could be answered in principle, in a much longer blog post, many of them are a matter of ongoing research, and some of them might never be answered at all. And we have not even touched the universe of earthquake forecasting that is beyond the world of ETAS.
Are we ever going to predict earthquakes? I don’t know.
Should we stop bothering? Certainly not. There are too many open questions.
Want to see more work from Leila? Find out what she’s up to on Twitter/X: @leilamizrahi.
References
- Böse, Maren, et al. “Towards a Dynamic Earthquake Risk Framework for Switzerland.” EGUsphere 2023 (2023, submitted): 1-33.
- Danciu, Laurentiu, et al. “The 2020 update of the European Seismic Hazard Model-ESHM20: Model Overview.” EFEHR Technical Report 1 (2021).
- Mizrahi, L. (2022). Towards Next Generation Time-Dependent Earthquake Forecasting (Doctoral dissertation, ETH Zurich).
- Mizrahi, Leila, et al. “D3. 5 Development, Testing, and Communicating Earthquake Fore-casts: Current Practices and Expert Recommendations.” (tech. rep.).
- Ogata Y. (1988). Statistical models for earthquake occurrences and residual analysis for point processes, J. Am. Stat. Assoc. 83, no. 401, 9–27.
This blog post was written by Leila Mizrahi, Postdoc at the Swiss Seismological Service, ETH Zürich. It was edited by ECS member Katinka Tuinstra and reviewed by ECS member Foivos Karakostas.