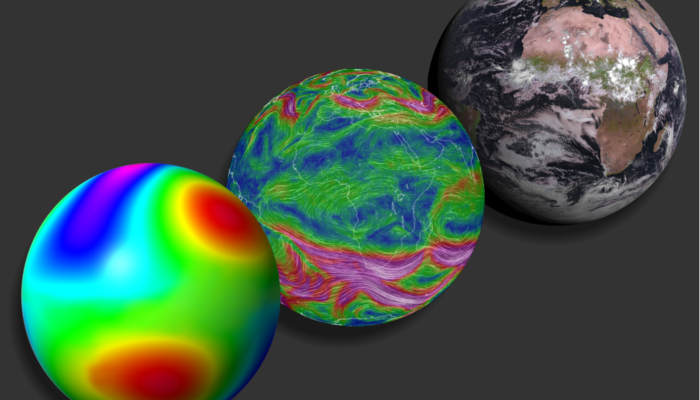
Do you gravitate to science because of the subject’s ability to explain complicated behaviour in nature through experimentation? When we see things or get our hands dirty by conducting experiments, it helps us comprehend scientific theories which are harder to explain. Introducing a complicated scientific theory is often carried out initially by presenting a simplified version. For example, students learn about Newtonian laws of motion prior to special relativity, ball and stick models of the molecule before quantum mechanics, and Euclidean geometry before the more spicy flavours of geometry. Likewise, idealised climate models can be used to teach, study, and explore the different behaviours exhibited by our climate.
Experiments in climate and weather, with few exceptions, such as cloud chambers, are limited to numerical simulations (although, some may argue that anthropogenic climate change is a real-world climate experiment). However, this does not make it easier to conduct. The sensitivity of the weather to the initial conditions and the large number of influencing factors adds to the difficulty and complicated nature of the climate system. The existence of unknown dynamic and thermodynamic processes associated with the weather (often called known unknowns) further compounds this complexity. The result is that, to study the Earth’s climate, researchers often use highly complicated numerical models, which are run on some of the most powerful computers in the world (Prathyush and Mihir used in India, Twin supercomputers Dogwood and Cactus in NOAA). These models are called Global Climate Models (GCMs).
The climate crisis, which refers to wide-ranging climate-driven changes across the planet including a rapid temperature increase, is altering climatic patterns and its occurrences. Understanding the impact of external forcings, either natural- or human- drivers, on the climate as well as predicting how it will change in the future is important now more than ever. Climate scientists are particularly worried that if certain climate thresholds are passed (so called tipping points), this could trigger irreversible changes in the Earth system, such as an ice-free Arctic or loss of permafrost. While GCMs are our best tool for understanding how the future climate will look, their complexity is also a limitation. For example, it can be difficult or sometimes impossible to mathematically analyse the model dynamics, or separate the key processes that cause a certain behaviour in the climate. Understanding where tipping point thresholds are can be difficult to identify in GCMs as there are a wealth of different interacting processes. This is where idealised models, also called toy models, come into play.
Toy models are GCMs ‘stripped to the bone’, which links theoretical understanding to the complexity of more realistic models. The model allows us to focus specifically on one or a small number of physical processes, and better understand how these could behave in a warmer future climate. Using simpler models allow mathematical tools to be more readily applied, which can be used to look for irreversible tipping points, or cascading events, where one tipping point leads to another.
Toy models used by climate scientists are thus a reduced version or low order model of the atmosphere or any other component of the Earth system. They are constructed by incorporating some of the factors and approximations (like Quasi geostrophic approximations if it is considered to happen in midlatitudes, or Hydrostatic approximation) that influence the sphere of interest. For example, the atmosphere, while attempting to eliminate as many irrelevant or superfluous factors as possible. Hence, their aim is not to provide a realistic representation of the climate system as a whole, but rather to mimic specific processes of interest. As a result of these simplifications, toy models are far more computationally efficient than GCMs and can run faster, allowing for many parameters to be tweaked. This results in the ability to test more scenarios.
An example of a toy model is Stommel’s Two-Box Model that was used in interpreting the existence of the two regimes of the Atlantic Meridional Overturning circulation (AMOC) (Stommel, 1961). The complicated dynamics of the Atlantic Ocean, which is driven by numerous physical and biological processes, was simplified into two boxes, dividing the Atlantic into north and south regions. In each box, the model tracked the temperature and the salinity of the water, and these two variables were assumed to be uniform within each box. This simplification boils the AMOC (which is the process that drives the Gulf Stream) down to its most basic processes, and was used to show how water density is the key variable in determining how intense the AMOC is.
Energy balance models, where the atmosphere is simplified into a number of distinct layers, are another example of toy models. In such models, the Earth’s temperature is calculated from measuring how much energy is trapped in the atmosphere from the incoming solar radiation. Such models can crudely simulate global warming and the greenhouse effect by increasing the amount of energy captured by the atmosphere. In addition, ice or snow cover can be simulated through making the ground more or less reflective, where more ice/snow reduces the Earth’s temperature because more sunlight is reflected away from the surface. These models can also be used to show how the temperature in the Arctic will rise faster than in areas closer to the equator with a warming climate (Graversen and Wang, 2009).
Hence, toy models are capable of providing powerful insights to key processes governing the climate system, at a low computational cost. This flexibility and adaptability make them a key complement to complex GCMs, for example by telling us where we should focus our attention on with the more powerful, but costly, GCMs. Hence, for a climate scientist, toy models are regarded as a crucial tool for learning and exploring weather and climate within the hierarchy of climate models.
This blog was edited by the editorial board.
References: [1] Graversen, R.G., Wang, M. Polar amplification in a coupled climate model with locked albedo. Clim Dyn 33, 629–643 (2009). https://doi.org/10.1007/s00382-009-0535-6 [2] Stommel, H., 1961. Thermohaline convection with two stable regimes of flow. Tellus, 13(2), pp.224-230.