Invited guest from the EGU 2024 Outstanding Student and PhD candidate Presentation (OSPP) Award Context The Sichuan Basin, located at the eastern slope of the Tibetan Plateau in China, is a lowland region that regularly experiences heavy rainfall during the summer months. This is due to its complex topographical features (Fig. 1) and the influence of the East Asian monsoon. The heavy rainfall can ...[Read More]
Can we improve our understanding of extreme precipitation events in the Sichuan Basin by using high-resolution regional climate models?
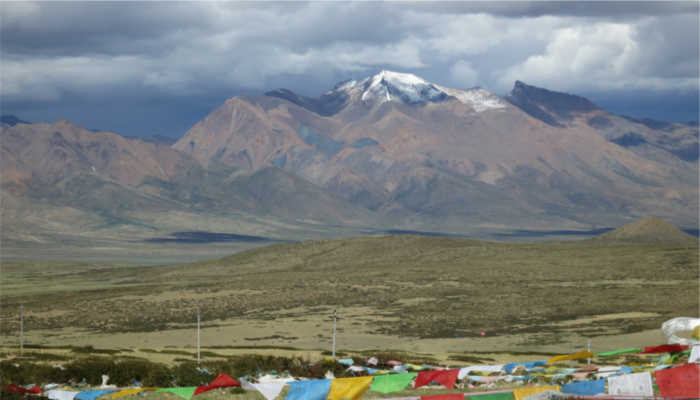