Face masks have been widely advocated to mitigate the airborne transmission of viruses including the severe acute respiratory syndrome coronavirus 2 (SARS-CoV-2) (e.g., Lelieveld et al., 2020). They can reduce the emission and spread of respiratory viruses through airborne droplets and aerosols as well as the inhalation of airborne viruses. The effectiveness of masks, however, is still under debate. The high penetration rates (around ~30-70%) of surgical and similar masks means that the wearer may still emit and inhale a large number of particles, and even FFP2/N95 respirators cannot prevent people from inhaling ambient particles (~5% penetration rate). Available randomized clinical trials show inconsistent or inconclusive results on face mask efficacy on preventing airborne virus transmission. On the other hand, observational data show that regions or facilities with a higher percentage of the population wearing masks have better control of the coronavirus disease 2019 (COVID-19).
In a new article published in the science magazine Science (Cheng et al., 2021), researchers from the Max-Planck Institute for Chemistry (Germany), Jinan University (China), and other institutions from Germany and the U.S. explained this discrepancy by combining knowledge from aerosol sciences and epidemiology studies. They point out that due to the nonlinear dependence of infection probability on inhaled virus number, the percentage change of infection probability due to mask use may not equal to the percentage change of inhaled virus number. The sensitivity of infection probability on the inhaled virus number strongly depends on the airborne concentration of viruses (Fig. 1). In a virus-limited regime, reducing inhaled virus number by wearing a mask will lead to a substantial reduction in the infection probability. In a virus-rich regime, however, the infection probability is close to unity and not sensitive to changes in inhaled virus number, thus wearing a mask may not suffice to prevent infection.
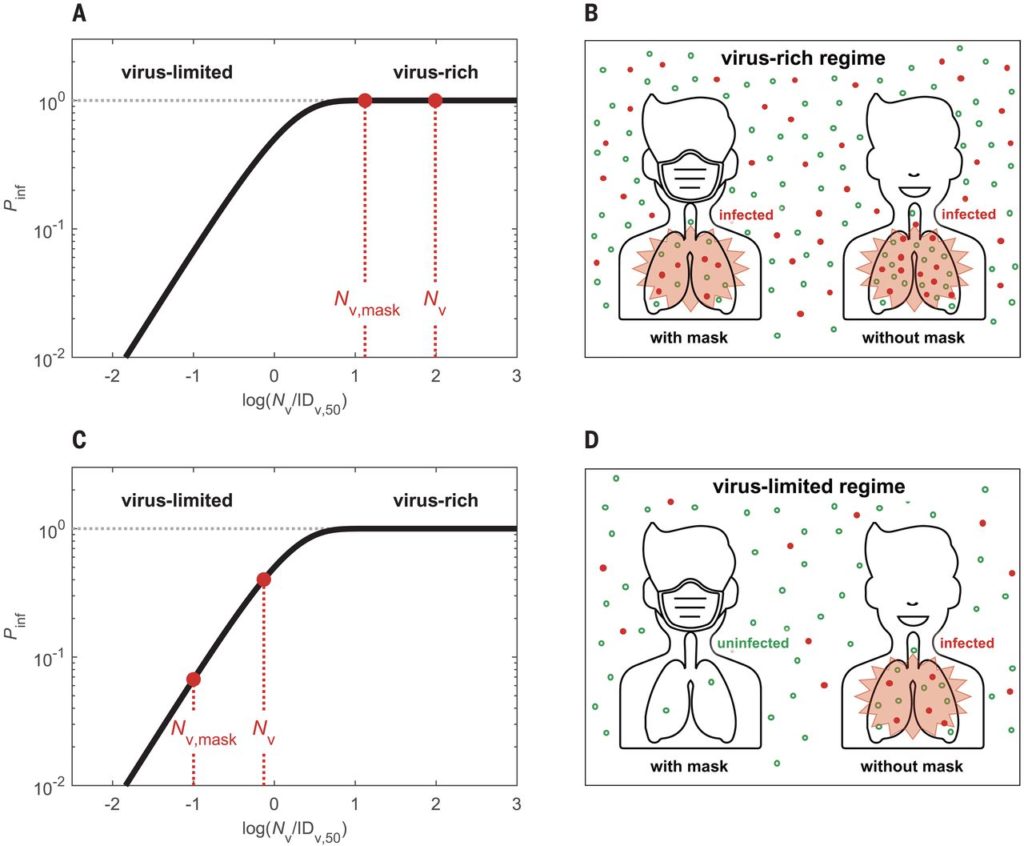
Figure 1 – Schematic illustration of different regimes of abundance of respiratory particles and viruses. The solid curve represents the infection probability (Pinf) as a function of inhaled virus number (Nv) scaled by median infectious dose IDv,50 at which Pinf = 50%. In the virus-rich regime (A, B), the concentration of airborne viruses is so high, that both number of viruses inhaled with or without mask (Nv,mask, Nv) are much higher than IDv,50 and Pinf remains close to ~1 even if masks are used. In the virus-limited regime (C, D), Nv and Nv,mask are close to or lower than IDv,50 and Pinf decreases substantially when masks are used, even if the masks cannot prevent the inhalation of all respiratory particles. In panels B and D, the red dots represent respiratory particles containing viruses, and the open green circles represent respiratory particles without viruses. This figure is direct adoption of Fig. 1 of Cheng et al. (2021).
Therefore, the key to quantifying the mask efficacy is to find out the regime of virus concentration in the ambient air. The basic reproduction number for COVID-19 (R0=2-4) suggests that the airborne concentrations of SARS-CoV-2 are in a virus-limited regime at a population average level (Fig. 2). Therefore, surgical masks can effectively limit the probability of community transmission of SARS-CoV-2 (Fig. 3).
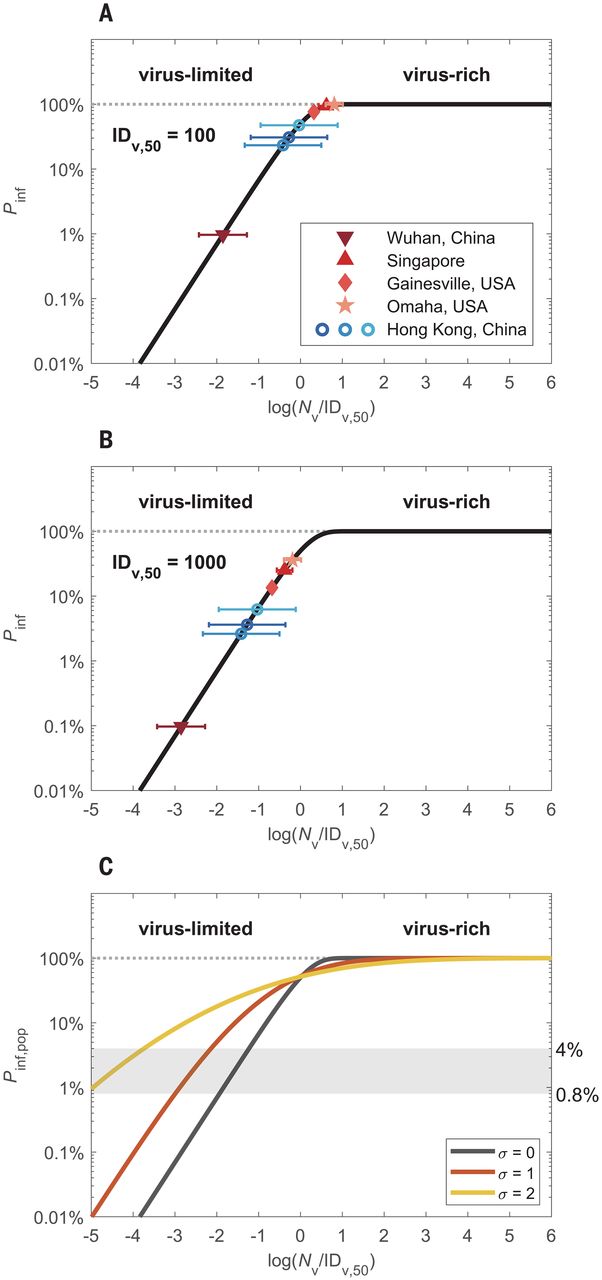
Figure 2 – Infection probabilities and abundance regimes of SARS-CoV-2 and other respiratory viruses. (A), (B) Individual infection probabilities (Pinf) plotted against inhaled virus number (Nv) scaled by characteristic median infectious doses of IDv,50 = 100 or 1000, respectively. The colored data points represent the mean numbers of viruses inhaled during a 30-minute period in different medical centers in China, Singapore, and the USA, according to measurement data of exhaled coronavirus, influenza virus, and rhinovirus numbers (blue circles) and of airborne SARS-CoV-2 number concentrations (red symbols), respectively. The error bars represent one geometric standard deviation. (C) Population-average infection probability (Pinf,pop) curves assuming lognormal distributions of Nv with different standard deviations of = 0, 1, and 2, respectively. The x-axis represents the mean value of log(Nv /IDv,50). The shaded area indicates the level of basic population-average infection probability, Pinf,pop,0, for SARS-CoV-2 as calculated from the basic reproduction number for COVID-19 and estimated values of average duration of infectiousness and daily number of contacts. This figure is direct adoption of Fig. 2 of Cheng et al. (2021).
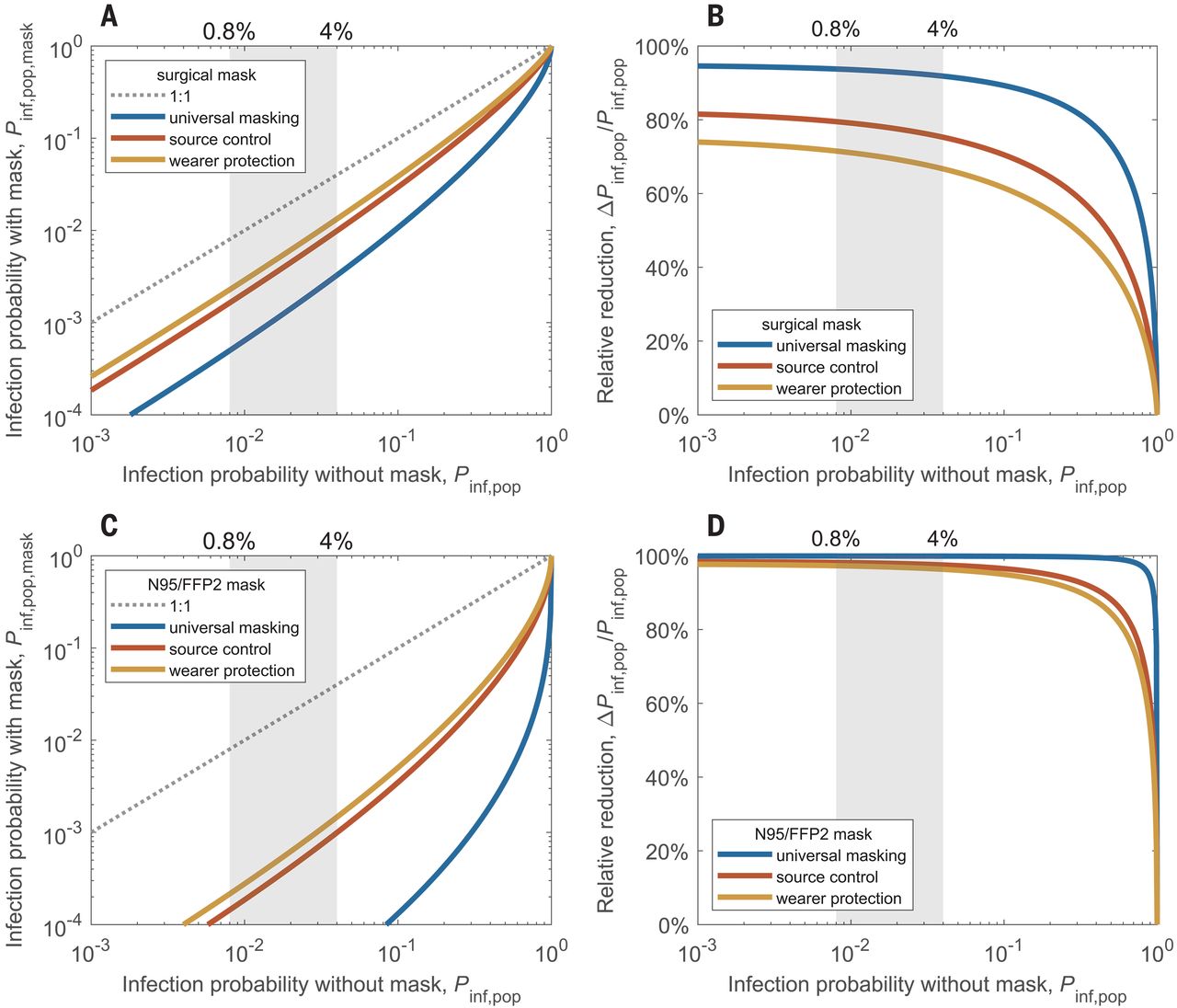
Figure 3 – Reduction of airborne transmission by face masks worn by infectious persons only (source control), by susceptible persons only (wearer protection), or by all persons (universal masking). (A) Population-average infection probability in case of mask use (Pinf,pop,mask) plotted against infection probability without face masks (Pinf,pop); and (B) corresponding mask efficacy, i.e., relative reduction of infection probability, ΔPinf,pop/Pinf,pop, plotted against Pinf,pop for surgical masks. (C) and (D) same as (A) and (B) but for N95/FFP2 masks. The lines represent the results obtained for source control (red line), wearer protection (yellow line), and the combination of both measures, i.e., universal masking (blue line) in a population where the virus exposure is lognormally distributed with a standard deviation of σ = 1. The shaded areas indicate the level of basic population-average infection probability, Pinf,pop,0, corresponding to the basic reproduction number for COVID-19. This figure is direct adoption of Fig. 3 of Cheng et al. (2021).
This study also pointed out that the synergistic effects of combining masks with other preventive measures can improve the effectiveness of each individual preventive measures. For example, ventilation may help to change the exposure environment from virus-rich to virus-limited conditions and increase the efficacy of face masks, which may be particularly important for medical centers with relatively high SARS-CoV-2 abundance (Fig. 2). On the other hand, not only the efficacy of face masks but also the efficacy of distancing may be reduced in virus-rich environments. Moreover, the results of Cheng et al. (2021) emphasized the importance of high compliance and correct use of masks to ensure the effectiveness of universal making in reducing the reproduction number of COVID-19 (Fig. 4).
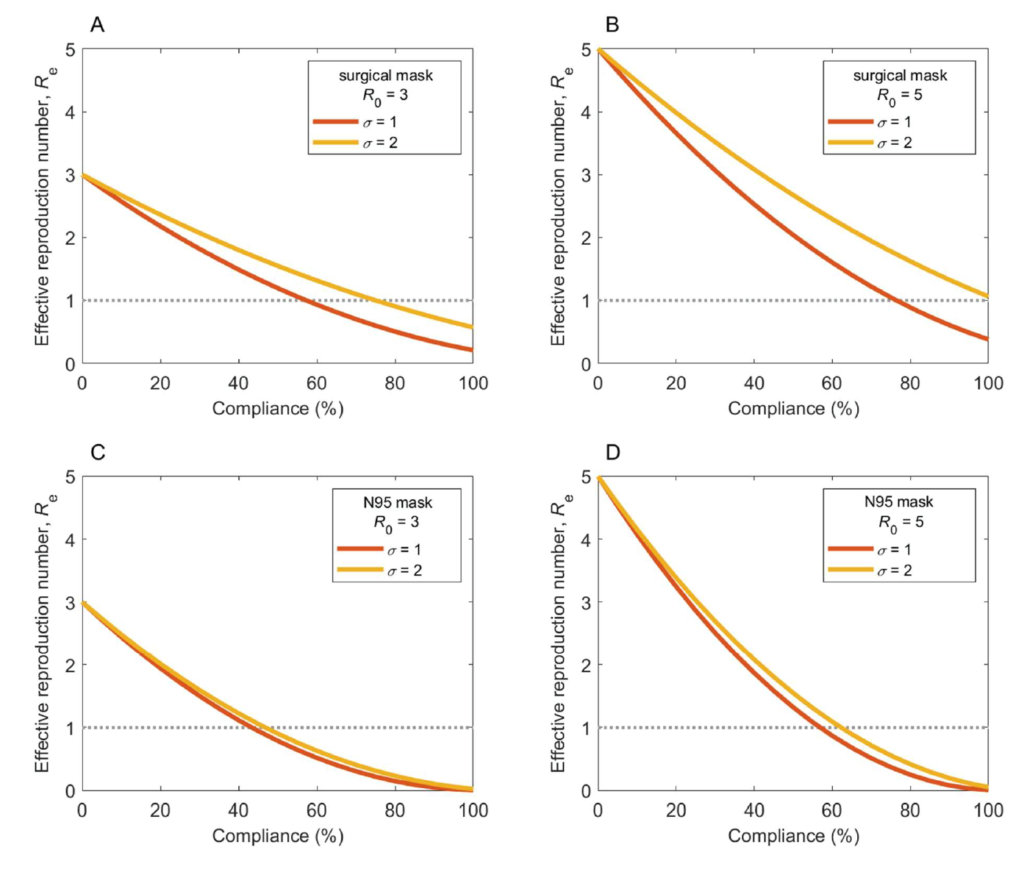
Figure 4 – Influence of compliance with universal masking on effective reproduction number (Re). The model results refer to the effect of universal masking without other preventive measures, assuming different R0 values and a lognormal distribution of Nv with a standard deviation = 1 or 2. (A) and (B) show the results for surgical masks. (C) and (D) show the results for N95/FFP2 masks. This figure is direct adoption of Fig. S11 of Cheng et al. (2021) and more details can be found in the study’s supplementary text S7.3.
Edited by Mengze Li
Reference
Cheng, Y., Ma, N., Witt, C., Rapp, S., Wild, P. S., Andreae, M. O., Pöschl, U., Su, H.: Face masks effectively limit the probability of SARS-CoV-2 transmission, Science, eabg6296, 2021.
Lelieveld, J., Helleis, F., Borrmann, S., Cheng, Y., Drewnick, F., Haug, G., Klimach, T., Sciare, J., Su, H., Pöschl, U.: Model calculations of aerosol transmission and infection risk of COVID-19 in indoor environments, Int. J. Environ. Res. Public Health, 17, 8114, 2020.
About the authors
Dr. Yafang Cheng is the Head of the Minerva Independent Research Group of Max Planck Institute for Chemistry in Germany, and currently is also a visiting professor at the University of Science and Technology of China. She is the senior editor of Atmospheric Chemistry and Physics, and a Steering Committee member of the Exploratory Round Table Conference (ERTC) between the Max Planck Society and the Chinese Academy of Sciences. She is mainly engaged in the research on the causes of air pollution and its climate effects and has achieved breakthrough results in basic theories and application. She received the Minerva Program Award of Max Planck Society, the Atmospheric Science Ascent Award of American Geophysical Union (AGU) and the Schmauss Award of German Association for Aerosol Research (GAef). (https://www.mpic.de/3599133/Profile_Y_Cheng)
Dr. Hang Su is the leader of Aerosol-Cloud-Biosphere Research Group of Max Planck Institute for Chemistry and is also a distinguished visiting professor at Shanghai Academy of Environmental Sciences. He is the associate editor of Atmospheric Chemistry and Physics, Journal of Geophysical Research, and Atmospheric Measurement and Techniques. He is mainly engaged in the research of atmospheric chemistry, atmosphere-biosphere interaction, and aerosol-cloud interaction. He received the Arne Richter Award for Outstanding Young Scientists of European Geosciences Union.
(https://www.mpic.de/3574358/Profile_Su)
Dr. Nan Ma is a professor at Institute for Environmental and Climate Research of Jinan University, China. His research mainly focuses on atmospheric aerosol and its climate, environment and health effects.
(https://ecins.jnu.edu.cn/2019/0725/c16567a370700/page.htm)